A Confidence Interval Is Constructed To Estimate The Value Of
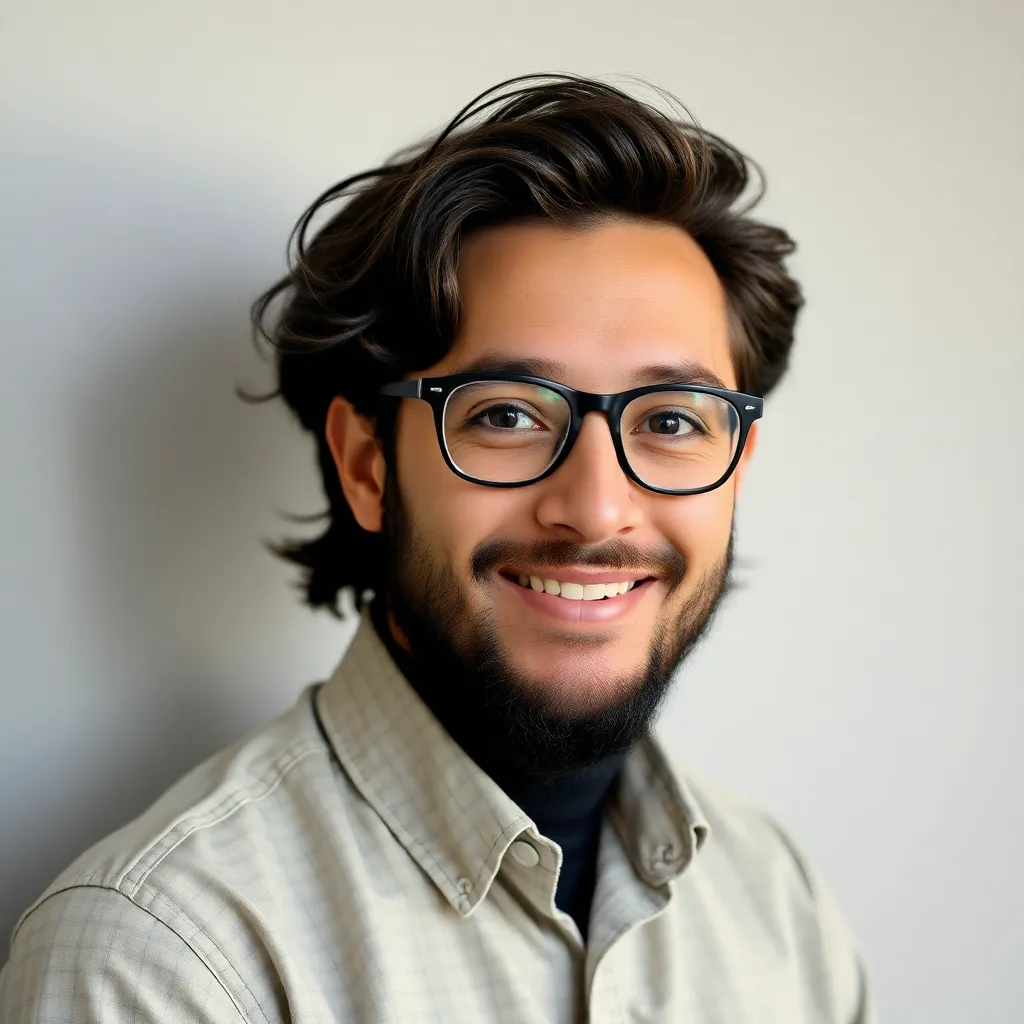
Muz Play
May 10, 2025 · 6 min read
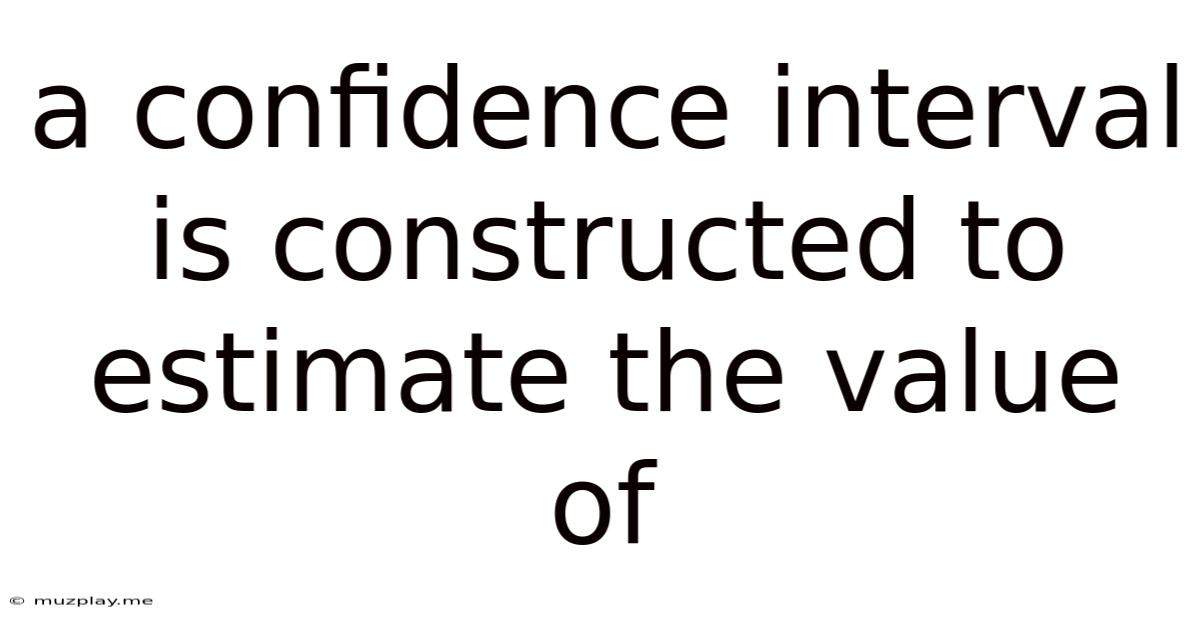
Table of Contents
Confidence Intervals: Estimating Population Parameters with Precision
A confidence interval is a range of values that, with a certain level of confidence, contains the true value of a population parameter. Instead of simply providing a point estimate (like a sample mean), a confidence interval acknowledges the inherent uncertainty in estimating population characteristics from sample data. This uncertainty arises because we're using a sample, not the entire population, and samples inherently vary. This article delves deep into confidence intervals, exploring their construction, interpretation, and practical applications, addressing key concepts like margin of error, confidence level, and the impact of sample size.
Understanding the Core Components of a Confidence Interval
Before diving into the specifics, let's lay the foundation by defining crucial elements:
1. Population Parameter: The Target of Our Estimation
The primary goal when constructing a confidence interval is to estimate an unknown population parameter. This could be:
- Population Mean (μ): The average value of a variable within the entire population. For example, the average height of all adult women in a country.
- Population Proportion (p): The proportion of individuals in a population possessing a certain characteristic. For instance, the percentage of voters who support a particular candidate.
- Population Variance (σ²): A measure of the spread or dispersion of data in the population. It reflects how much the individual data points deviate from the mean.
- Population Standard Deviation (σ): The square root of the population variance, providing another measure of data spread.
2. Sample Statistic: Our Best Guess Based on Available Data
Since we rarely have access to the entire population, we use a sample statistic to estimate the population parameter. The sample statistic corresponds to the population parameter we're estimating:
- Sample Mean (x̄): The average value of the variable in our sample.
- Sample Proportion (p̂): The proportion of individuals in our sample possessing the characteristic of interest.
- Sample Variance (s²): The measure of spread in our sample data.
- Sample Standard Deviation (s): The square root of the sample variance.
3. Confidence Level: Expressing Certainty in Our Interval
The confidence level indicates the probability that the confidence interval will contain the true population parameter. Common confidence levels are 90%, 95%, and 99%. A 95% confidence level means that if we were to repeatedly draw samples and construct confidence intervals using the same method, 95% of those intervals would contain the true population parameter. This does not mean there's a 95% chance the true value lies within this specific interval; the true value is either within the interval or it isn't. The confidence level reflects the long-run performance of the method.
4. Margin of Error: Quantifying Uncertainty
The margin of error is the amount added and subtracted from the sample statistic to create the upper and lower bounds of the confidence interval. It represents the uncertainty associated with using a sample to estimate a population parameter. A smaller margin of error indicates greater precision in our estimate. The margin of error is directly related to the confidence level and the sample size; larger sample sizes lead to smaller margins of error.
5. Critical Value: Linking Confidence Level to the Distribution
The critical value is a number obtained from a probability distribution (typically the Z-distribution for large samples or the t-distribution for small samples) that corresponds to the chosen confidence level. It determines how many standard errors to add and subtract from the sample statistic to calculate the margin of error. For example, a 95% confidence level corresponds to a critical Z-value of approximately 1.96.
Constructing Confidence Intervals: Different Scenarios
The specific formula used to construct a confidence interval depends on the parameter being estimated and the characteristics of the data.
1. Confidence Interval for a Population Mean (Large Sample)
When the sample size is large (generally considered n ≥ 30) and the population standard deviation (σ) is known, we use the Z-distribution:
Confidence Interval = x̄ ± Z * (σ / √n)
Where:
- x̄ is the sample mean
- Z is the critical Z-value corresponding to the chosen confidence level
- σ is the population standard deviation
- n is the sample size
If the population standard deviation (σ) is unknown, we can substitute the sample standard deviation (s) provided that the sample size is large enough. The Central Limit Theorem justifies this substitution.
2. Confidence Interval for a Population Mean (Small Sample)
When the sample size is small (n < 30) and the population standard deviation is unknown, we use the t-distribution:
Confidence Interval = x̄ ± t * (s / √n)
Where:
- x̄ is the sample mean
- t is the critical t-value from the t-distribution with (n-1) degrees of freedom and the chosen confidence level
- s is the sample standard deviation
- n is the sample size
The t-distribution accounts for the extra uncertainty introduced by estimating the population standard deviation from the sample.
3. Confidence Interval for a Population Proportion
For estimating a population proportion, we use the following formula:
Confidence Interval = p̂ ± Z * √[(p̂(1-p̂)) / n]
Where:
- p̂ is the sample proportion
- Z is the critical Z-value corresponding to the chosen confidence level
- n is the sample size
This formula assumes a large enough sample size to ensure the sampling distribution of p̂ is approximately normal. A rule of thumb is that both np̂ and n(1-p̂) should be greater than 5.
Interpreting Confidence Intervals: What Does it Really Mean?
A confidence interval doesn't provide a probability that the true population parameter falls within the calculated range; it reflects the long-run reliability of the estimation method. The correct interpretation is: "We are 95% confident (or whatever the chosen confidence level is) that the true population parameter lies within this interval." This confidence arises from the repeated sampling process; if we were to repeatedly sample and construct intervals using this method, 95% of those intervals would contain the true population parameter.
Factors Affecting Confidence Interval Width
The width of the confidence interval, which reflects the precision of the estimate, is influenced by several factors:
- Confidence Level: Higher confidence levels (e.g., 99% vs. 95%) result in wider intervals because greater certainty requires a larger range.
- Sample Size: Larger sample sizes lead to narrower intervals. Larger samples provide more precise estimates, reducing uncertainty.
- Sample Variability: Higher variability in the sample data (larger standard deviation) results in wider intervals, reflecting greater uncertainty.
Applications of Confidence Intervals
Confidence intervals have widespread applications across various fields:
- Market Research: Estimating the proportion of consumers who prefer a certain product.
- Medical Research: Estimating the effectiveness of a new treatment by comparing treatment and control group outcomes.
- Quality Control: Monitoring the consistency of a manufacturing process by measuring the mean and variance of a key characteristic.
- Political Polling: Predicting election outcomes by estimating the proportion of voters supporting each candidate.
- Environmental Science: Estimating population sizes of endangered species.
Conclusion: Embracing Uncertainty for Better Estimation
Confidence intervals are invaluable tools for statistical inference, providing a more nuanced and realistic way to estimate population parameters than simple point estimates. By explicitly acknowledging the uncertainty inherent in using sample data, confidence intervals offer a more complete and reliable picture of the population characteristic being studied. Understanding the principles behind confidence interval construction and interpretation is crucial for anyone working with statistical data, enabling more informed decision-making across diverse fields. Remember to carefully consider the choice of confidence level, sample size, and the appropriateness of the chosen statistical method when constructing and interpreting these valuable statistical tools. The correct interpretation, focusing on the long-run reliability of the method, is essential for avoiding common misconceptions.
Latest Posts
Latest Posts
-
Stomata Appear In Which Group Of Plants
May 10, 2025
-
De Donde Son Los Aztecas Y Mayas
May 10, 2025
-
How Many Elements Belong To The Halogen Family
May 10, 2025
-
Which Of The Following Are Only Found In Animal Cells
May 10, 2025
-
An Inoculating Loop Or Needle Is Sterilized Using Heat
May 10, 2025
Related Post
Thank you for visiting our website which covers about A Confidence Interval Is Constructed To Estimate The Value Of . We hope the information provided has been useful to you. Feel free to contact us if you have any questions or need further assistance. See you next time and don't miss to bookmark.