A Frequency Table For Qualitative Data Has Class Limits.
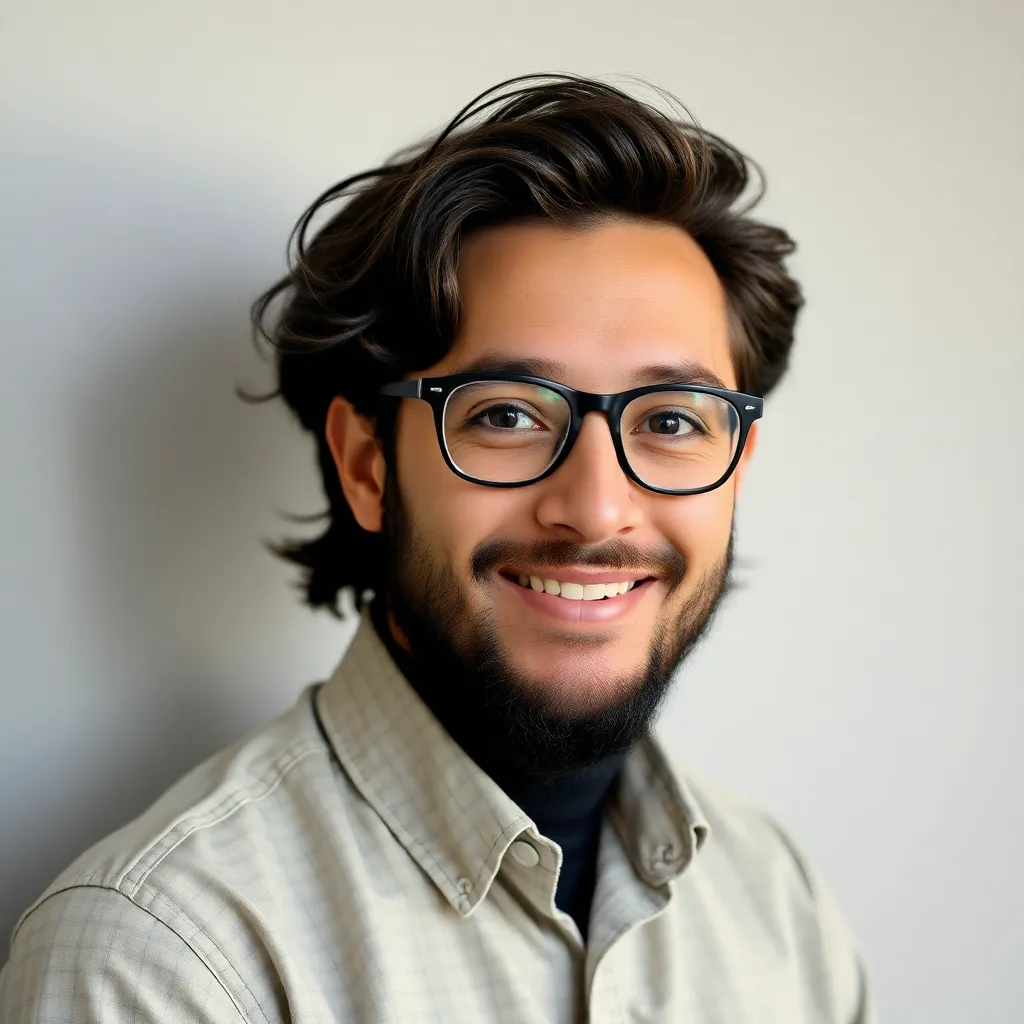
Muz Play
May 10, 2025 · 5 min read
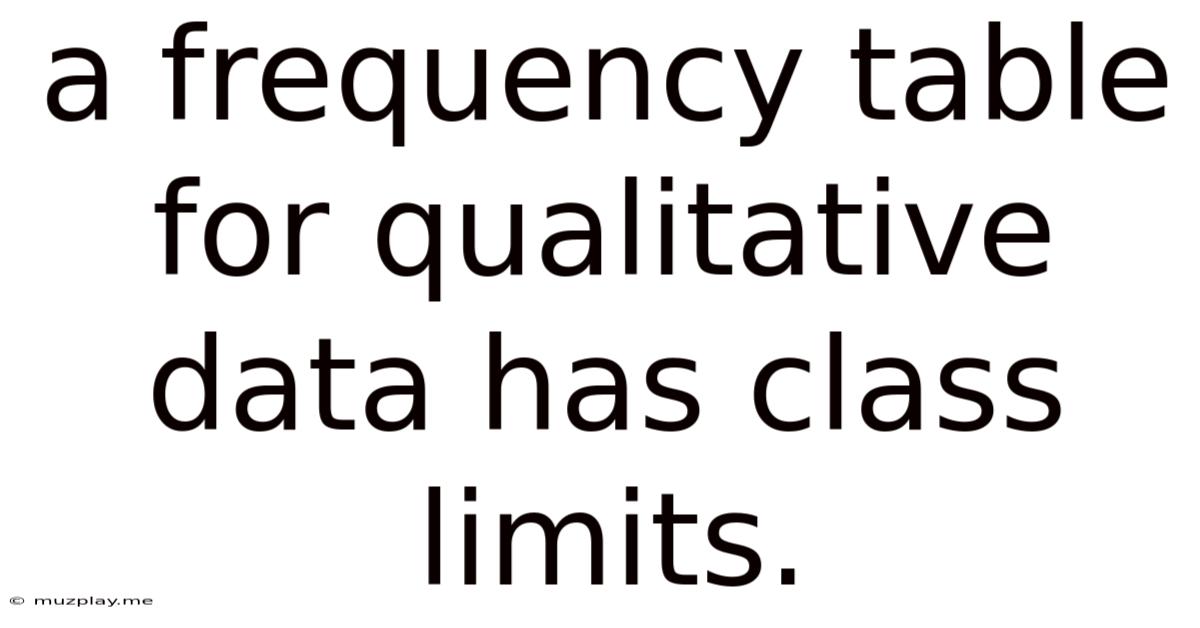
Table of Contents
Frequency Tables for Qualitative Data: Dispelling the Myth of Class Limits
The statement "a frequency table for qualitative data has class limits" is fundamentally incorrect. This misconception often arises from conflating the principles of organizing and presenting quantitative and qualitative data. Understanding the key differences is crucial for accurate data analysis and effective communication of research findings. This article will delve into the nature of frequency tables for both quantitative and qualitative data, explaining why class limits are inapplicable to the latter and exploring alternative methods of representation.
Understanding Frequency Tables: A Foundation
A frequency table is a method of summarizing data by grouping values and counting their occurrences. It provides a concise and organized overview of the distribution of data, making it easier to identify patterns, trends, and outliers. However, the construction and interpretation of frequency tables differ significantly depending on whether the data is quantitative or qualitative.
Quantitative Data: The Realm of Class Limits
Quantitative data consists of numerical values that can be measured and ordered. Examples include height, weight, age, temperature, and income. When creating a frequency table for quantitative data, we often group the data into class intervals or bins. These intervals have defined class limits:
- Lower Class Limit: The smallest value that can belong to a particular class interval.
- Upper Class Limit: The largest value that can belong to a particular class interval.
- Class Width: The difference between the upper and lower class limits of a class interval.
For example, consider a dataset of student ages. We might group them into intervals like 18-20, 21-23, 24-26, etc. Here:
- 18 is the lower class limit of the first interval.
- 20 is the upper class limit of the first interval.
- The class width is 3 (20 - 18 + 1 = 3).
Why Class Limits are Necessary for Quantitative Data: Class limits provide clear boundaries for each interval, ensuring that each data point is assigned to only one class. This is essential for avoiding ambiguity and maintaining the accuracy of the frequency count. The use of class intervals allows for a manageable representation of a large dataset, highlighting the overall distribution and identifying potential central tendencies and dispersion.
Qualitative Data: A Different Approach
Qualitative data, on the other hand, represents characteristics or attributes that are not numerical. Examples include gender, eye color, favorite food, brand preference, and country of origin. Qualitative data is typically categorical, and the categories are not inherently ordered. While we can still create frequency tables for qualitative data, the concept of class limits is entirely irrelevant.
Frequency Tables for Qualitative Data: Categorical Counts
For qualitative data, the frequency table directly lists the categories and their corresponding frequencies (counts). There's no need for class intervals or class limits because each category is already a distinct and independent unit. The focus is on determining the frequency of each qualitative attribute within the dataset.
Example: Consider a survey on preferred coffee types. The categories might include "Espresso," "Latte," "Cappuccino," "Americano," and "Other." A frequency table would simply list these categories and count how many respondents chose each option. There's no concept of an upper or lower limit for "Latte" – it's simply a distinct category.
Coffee Type | Frequency |
---|---|
Espresso | 25 |
Latte | 38 |
Cappuccino | 20 |
Americano | 12 |
Other | 5 |
This table effectively summarizes the qualitative data without the need for class limits or intervals. The focus is on the count of observations within each distinct category.
Misinterpretations and Potential Errors
The erroneous application of class limits to qualitative data can lead to several problems:
- Meaningless Intervals: Creating artificial intervals for qualitative data destroys the inherent meaning of the categories. For example, trying to create intervals for "favorite colors" would be nonsensical.
- Incorrect Frequency Counts: Arbitrarily grouping qualitative categories can lead to inaccurate frequency counts and a distorted representation of the data.
- Loss of Information: The natural distinctions between qualitative categories are blurred by grouping them into intervals. This loss of granularity can prevent researchers from extracting valuable insights.
Advanced Techniques for Qualitative Data Analysis
While simple frequency tables suffice for basic analysis, more sophisticated techniques can provide deeper insights into qualitative data:
- Relative Frequency: Expressing the frequency of each category as a percentage of the total number of observations offers a more comparative perspective.
- Cumulative Frequency: Calculating the cumulative frequency shows the accumulated count up to each category. This is particularly useful for understanding the proportion of responses that fall within a certain range of categories.
- Bar Charts and Pie Charts: These visual representations provide intuitive ways of showcasing the frequency distribution of qualitative data, facilitating easier interpretation and communication.
- Cross-tabulation: Analyzing the relationships between two or more qualitative variables through cross-tabulation reveals potential correlations and interactions. This technique is highly valuable in understanding the combined effects of multiple factors.
- Qualitative Data Analysis Software: Specialized software packages, such as NVivo or Atlas.ti, are designed for more in-depth analysis of qualitative data, enabling researchers to explore themes, identify patterns, and perform more complex analysis.
Conclusion: Accurate Representation is Key
The accurate representation and analysis of data are fundamental to successful research. Confusing the methodologies for quantitative and qualitative data analysis can lead to misleading interpretations and flawed conclusions. Remembering that frequency tables for qualitative data do not utilize class limits is a critical step towards accurate data analysis and the effective communication of research findings. By employing the appropriate techniques and recognizing the unique characteristics of each data type, researchers can extract meaningful insights and effectively communicate their results. Utilizing relative frequencies, cumulative frequencies, and visual representations such as bar charts and pie charts enhances the understanding and communication of findings derived from qualitative data analysis. Employing more advanced techniques and potentially utilizing specialized software empowers deeper analysis and facilitates the uncovering of intricate relationships within the dataset. The key takeaway is to always align the chosen method of analysis with the nature of the data being studied for accurate and meaningful results.
Latest Posts
Latest Posts
-
Consider A Circuit Consisting Of Several Resistors Connected In Series
May 10, 2025
-
Plants Make Their Own Food Therefore They Are Classified As
May 10, 2025
-
Endocytosis And Exocytosis Are Means Of Transport Used By
May 10, 2025
-
Employers Must Not Place Construction Loads On Concrete Structures
May 10, 2025
-
Electric Field Of A Line Of Charge
May 10, 2025
Related Post
Thank you for visiting our website which covers about A Frequency Table For Qualitative Data Has Class Limits. . We hope the information provided has been useful to you. Feel free to contact us if you have any questions or need further assistance. See you next time and don't miss to bookmark.