A Statistical Hypothesis Is A Statement About A Sample
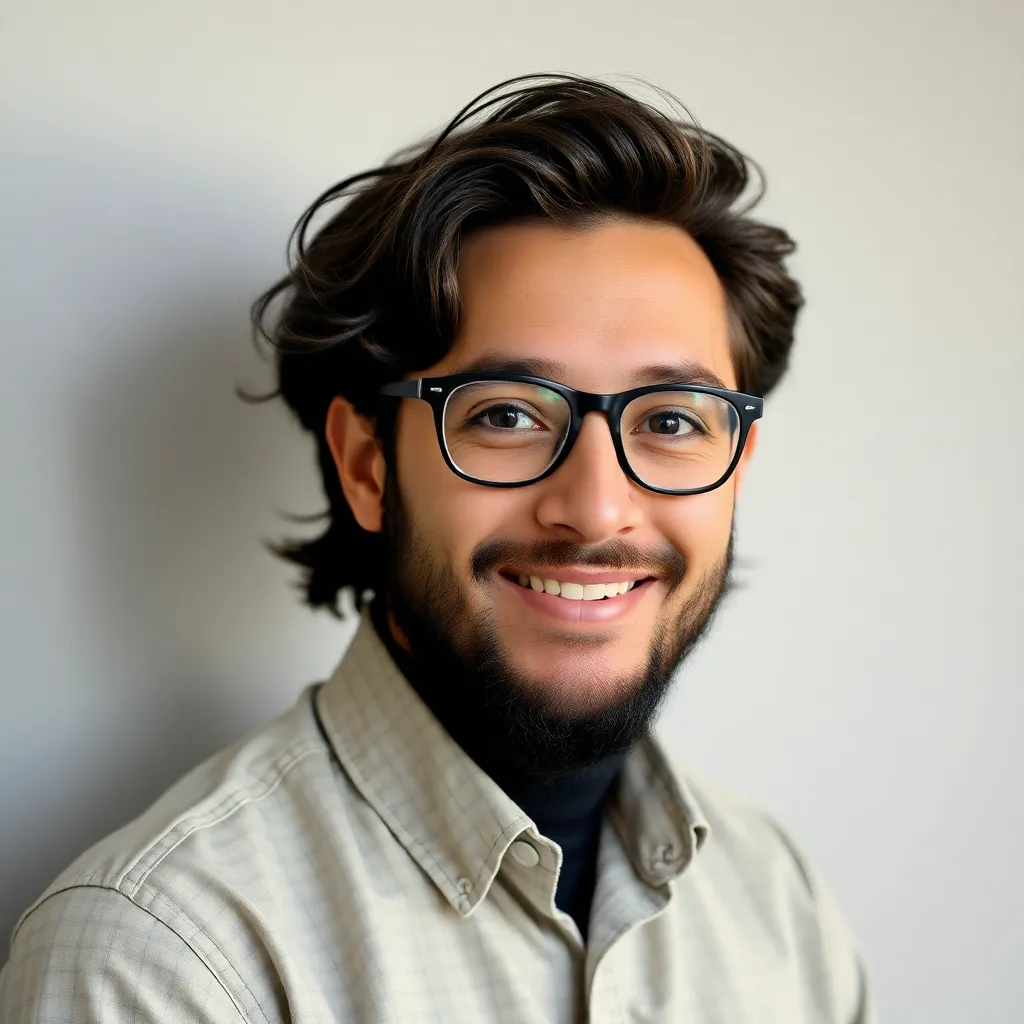
Muz Play
Apr 16, 2025 · 7 min read

Table of Contents
A Statistical Hypothesis is a Statement About a Sample: Delving Deep into Hypothesis Testing
Statistical hypothesis testing forms the bedrock of much of scientific inquiry and data analysis. While often presented in a complex manner, the core concept is surprisingly straightforward: a statistical hypothesis is fundamentally a statement about a sample drawn from a larger population. This article delves into the intricacies of this concept, exploring its various facets and demonstrating its critical role in drawing meaningful conclusions from data.
Understanding the Fundamentals: Population vs. Sample
Before dissecting the notion of a hypothesis as a statement about a sample, let's clarify the fundamental distinction between a population and a sample.
-
Population: The population is the entire group you are interested in studying. It could be anything from all the students in a university, all the trees in a forest, to all the stars in a galaxy. The population is often vast and, in many cases, impossible to study completely.
-
Sample: A sample is a smaller, manageable subset of the population. It's selected from the population to represent its characteristics. The goal is to draw inferences about the population based on the analysis of the sample. The key is that the sample must be representative – meaning it accurately reflects the characteristics of the larger population it's drawn from. Biases in sampling can significantly skew results and render conclusions invalid.
The Hypothesis: A Testable Statement About the Sample
A statistical hypothesis is a testable statement about a population parameter based on the evidence gathered from a sample. It's not a guess or an opinion; rather, it's a precise, falsifiable claim that can be assessed using statistical methods. Crucially, the hypothesis focuses on the sample's characteristics to infer something about the population. This is because directly examining the entire population is often impractical or impossible.
There are two main types of hypotheses:
-
Null Hypothesis (H₀): This is a statement of "no effect" or "no difference." It represents the status quo or the default assumption. For example, if comparing the effectiveness of two drugs, the null hypothesis might be that there's no difference in their efficacy. The null hypothesis is usually what the researcher tries to reject.
-
Alternative Hypothesis (H₁ or Hₐ): This is a statement that contradicts the null hypothesis. It suggests there is an effect, a difference, or a relationship. In the drug example, the alternative hypothesis might be that one drug is more effective than the other. The alternative hypothesis is what the researcher hopes to support with evidence from the sample.
How Sample Data Informs the Hypothesis
The process of hypothesis testing involves collecting data from a sample, performing statistical analyses, and then using the results to either reject or fail to reject the null hypothesis. The choice depends on the calculated probability (p-value) that the observed results could have occurred by chance alone if the null hypothesis were true. A low p-value (typically below a pre-determined significance level, often 0.05) suggests strong evidence against the null hypothesis, leading to its rejection. A high p-value indicates insufficient evidence to reject the null hypothesis. Importantly, failing to reject the null hypothesis does not prove it is true; it simply means there isn't enough evidence from the sample to reject it.
Examples Illustrating Hypotheses about Samples
Let's consider some real-world examples to solidify our understanding:
Example 1: Average Height of College Students
- Population: All college students in a particular country.
- Sample: A randomly selected group of 100 college students from various universities across the country.
- Null Hypothesis (H₀): The average height of college students in the country is 175 cm.
- Alternative Hypothesis (H₁): The average height of college students in the country is not 175 cm.
The researcher would measure the heights of the 100 students in the sample and use statistical tests (like a t-test) to determine if the sample average is significantly different from 175 cm. This would provide evidence to either reject or fail to reject the null hypothesis regarding the average height of the entire population of college students.
Example 2: Effectiveness of a New Fertilizer
- Population: All tomato plants that could be grown using a specific type of soil.
- Sample: 50 tomato plants grown using the new fertilizer and 50 tomato plants grown using a standard fertilizer (control group).
- Null Hypothesis (H₀): The new fertilizer has no effect on the yield of tomatoes.
- Alternative Hypothesis (H₁): The new fertilizer increases the yield of tomatoes.
The researcher would measure the tomato yield from both groups and use statistical tests (like an ANOVA or t-test) to compare the average yields. A significant difference in the sample means could lead to the rejection of the null hypothesis, suggesting the new fertilizer is effective.
Example 3: Relationship Between Exercise and Blood Pressure
- Population: All adults aged 30-50.
- Sample: A randomly selected group of 200 adults aged 30-50, with half participating in a regular exercise program and the other half serving as a control group.
- Null Hypothesis (H₀): There is no relationship between regular exercise and blood pressure.
- Alternative Hypothesis (H₁): Regular exercise lowers blood pressure.
The researcher would measure the blood pressure of both groups and use correlation or regression analysis to determine the strength and direction of the relationship between exercise and blood pressure in the sample. The results would inform whether the null hypothesis should be rejected or not.
The Importance of Proper Sampling
The accuracy and reliability of hypothesis testing hinge critically on the quality of the sample. A biased or non-representative sample can lead to erroneous conclusions, regardless of the sophistication of the statistical methods employed. Several sampling techniques aim to ensure representativeness, such as:
- Simple Random Sampling: Each member of the population has an equal chance of being selected.
- Stratified Random Sampling: The population is divided into strata (subgroups), and random samples are drawn from each stratum.
- Cluster Sampling: The population is divided into clusters, and a random sample of clusters is selected. All members within the selected clusters are then included in the sample.
Choosing the appropriate sampling method is crucial for ensuring the validity and generalizability of the results.
Limitations and Misinterpretations of Hypothesis Testing
While a powerful tool, hypothesis testing has limitations and is prone to misinterpretations:
- Type I Error: Rejecting the null hypothesis when it is actually true (false positive). The significance level (alpha) controls the probability of a Type I error.
- Type II Error: Failing to reject the null hypothesis when it is actually false (false negative). The power of the test affects the probability of a Type II error.
- Statistical Significance vs. Practical Significance: A statistically significant result might not be practically significant. A small difference might be statistically significant with a large sample size but have little real-world impact.
- Correlation vs. Causation: Statistical tests can reveal correlations between variables, but correlation does not imply causation. Other factors could be responsible for the observed relationship.
Conclusion: The Sample as a Window to the Population
A statistical hypothesis is fundamentally a statement about a sample drawn from a larger population. By carefully selecting a representative sample and employing appropriate statistical methods, researchers can use the sample data to draw inferences about the population, test hypotheses, and contribute to scientific understanding. However, it is crucial to be mindful of the limitations of hypothesis testing and to avoid common misinterpretations. Understanding the relationship between sample and population is essential for the correct interpretation and application of statistical findings. The sample acts as a window through which we attempt to understand the properties and characteristics of the much larger, often inaccessible, population. The rigor of the sampling method and the careful application of statistical analysis are crucial steps to ensure this window offers a clear and accurate view.
Latest Posts
Latest Posts
-
Why Does Radius Decrease Across A Period
Apr 19, 2025
-
The Krebs Cycle Takes Place In
Apr 19, 2025
-
Confidence Interval For Slope Of Regression Line Formula
Apr 19, 2025
-
What Is The Difference Between Culture And Society
Apr 19, 2025
-
During His Trip On The Beagle Darwin Found That
Apr 19, 2025
Related Post
Thank you for visiting our website which covers about A Statistical Hypothesis Is A Statement About A Sample . We hope the information provided has been useful to you. Feel free to contact us if you have any questions or need further assistance. See you next time and don't miss to bookmark.