According To The Logistic Growth Equation
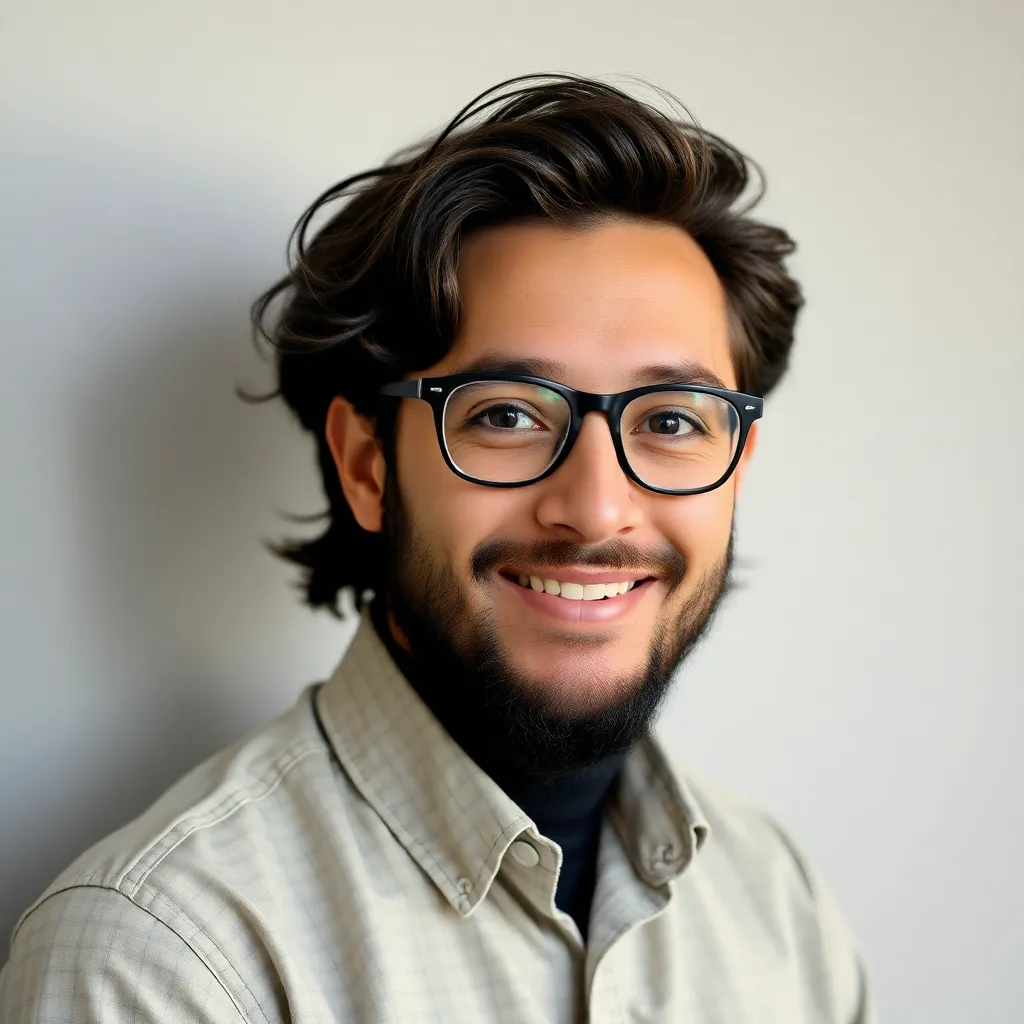
Muz Play
May 10, 2025 · 6 min read
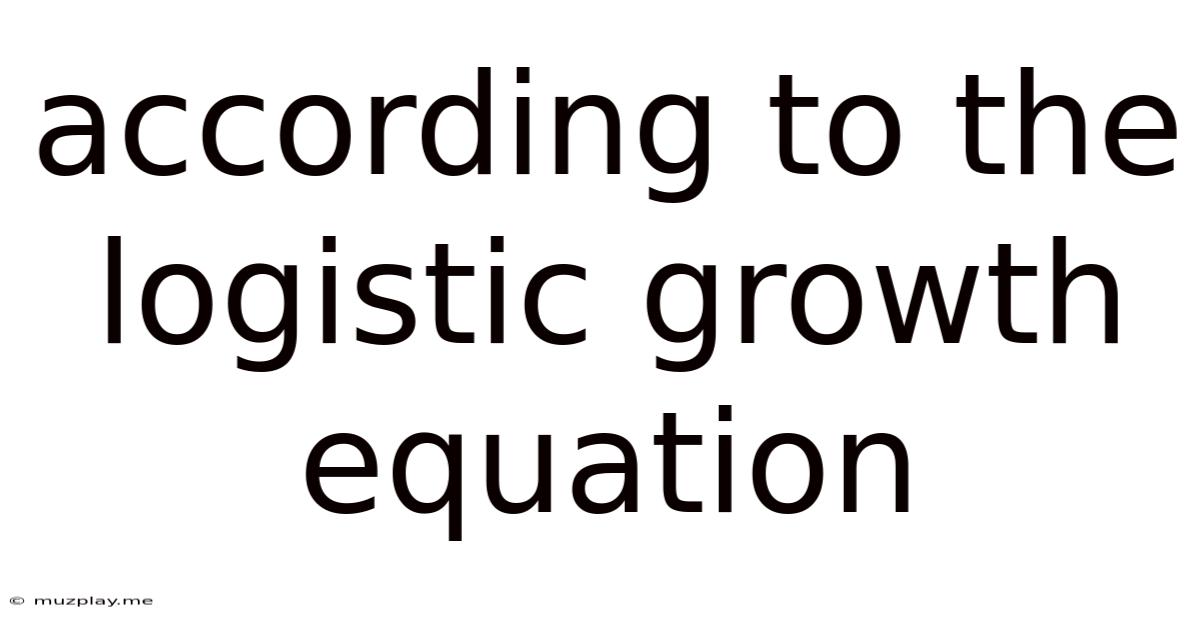
Table of Contents
According to the Logistic Growth Equation: A Deep Dive into Population Dynamics
The logistic growth equation, a cornerstone of population ecology and mathematical biology, provides a more realistic model of population growth than the simpler exponential model. Unlike exponential growth, which assumes unlimited resources and continuous growth, the logistic model incorporates the concept of carrying capacity – the maximum population size an environment can sustain. This article delves into the intricacies of the logistic growth equation, exploring its derivation, applications, limitations, and modifications.
Understanding the Fundamentals: Exponential vs. Logistic Growth
Before diving into the specifics of the logistic equation, it's crucial to understand the limitations of the exponential growth model. The exponential growth equation, often represented as dN/dt = rN (where N is population size, t is time, and r is the per capita rate of increase), assumes an environment with unlimited resources, allowing a population to grow indefinitely. This is rarely the case in real-world scenarios.
Exponential Growth: Imagine a small population of bacteria in a nutrient-rich broth. Initially, their growth appears exponential; their numbers double, then double again, and so on. However, this rapid expansion eventually plateaus as the bacteria deplete the available nutrients and face increased competition for resources.
Logistic Growth: The logistic model accounts for this reality by introducing the concept of carrying capacity (K). Carrying capacity represents the maximum population size that a given environment can support indefinitely, given the available resources. As the population approaches K, the growth rate slows down, eventually reaching zero when the population size equals K.
The Logistic Growth Equation: Derivation and Interpretation
The logistic growth equation is expressed as:
dN/dt = rN[(K - N)/K]
Let's break down each component:
-
dN/dt: This represents the rate of change in population size (N) over time (t). It essentially describes how quickly the population is growing or shrinking.
-
r: This is the intrinsic rate of increase, representing the per capita rate of population growth under ideal conditions (i.e., unlimited resources). It's analogous to the 'r' in the exponential growth equation.
-
N: This is the current population size.
-
K: This is the carrying capacity, representing the maximum sustainable population size in the given environment.
(K - N)/K: This term is the key difference between the logistic and exponential models. It represents the environmental resistance to growth as the population approaches its carrying capacity. As N approaches K, this term approaches zero, slowing the rate of population growth. When N = K, the growth rate becomes zero, indicating that the population has reached its carrying capacity.
Solving the Logistic Growth Equation: Analytical and Numerical Approaches
Solving the logistic growth equation analytically yields a sigmoid (S-shaped) curve. This curve visually represents the transition from exponential growth at lower population sizes to a plateau as the population approaches carrying capacity. The analytical solution is:
N(t) = K / [1 + (K/N₀ - 1)e^(-rt)]
Where:
- N(t) is the population size at time t.
- N₀ is the initial population size.
- e is the base of the natural logarithm.
While the analytical solution provides a precise mathematical description, numerical methods are often employed, especially when dealing with complex scenarios or variations of the logistic model. Numerical methods, such as Euler's method or Runge-Kutta methods, approximate the solution by iteratively calculating the population size at small time intervals. These methods are particularly useful when the equation cannot be solved analytically or when incorporating additional factors influencing population dynamics.
Applications of the Logistic Growth Equation
The logistic growth model finds extensive application in various fields:
1. Ecology and Population Biology:
-
Modeling animal populations: The logistic equation is used to predict the growth of animal populations in controlled environments or natural ecosystems. Factors influencing carrying capacity, such as food availability, predation, and disease, can be incorporated into the model to improve its predictive accuracy.
-
Invasive species management: Understanding the growth patterns of invasive species is crucial for effective management strategies. The logistic model can help predict the spread and potential impact of invasive populations, aiding in the development of control measures.
-
Fisheries management: Sustainable fishing practices rely on accurate assessments of fish populations. The logistic model can help estimate the maximum sustainable yield – the highest rate at which fish can be harvested without depleting the population.
2. Epidemiology:
-
Modeling disease outbreaks: The logistic model can be adapted to model the spread of infectious diseases within a population. The carrying capacity in this context might represent the total susceptible population.
-
Predicting epidemic peaks: Understanding the growth dynamics of an epidemic allows for better resource allocation and public health interventions. The logistic model can help predict the peak of an outbreak and its overall duration.
3. Other Applications:
-
Economics: The logistic model can be used to model the growth of markets, the diffusion of innovations, and other economic phenomena that exhibit a period of rapid growth followed by saturation.
-
Social Sciences: It can be applied to model the growth of social movements, the spread of ideas, and other social processes that exhibit similar patterns.
Limitations and Modifications of the Logistic Growth Equation
Despite its usefulness, the logistic model has several limitations:
-
Assumption of constant parameters: The logistic equation assumes that parameters like r and K remain constant over time. In reality, these parameters can fluctuate due to environmental changes, resource availability, and other factors.
-
Simplified representation of interactions: The model simplifies the complex interactions within a population and between the population and its environment. It doesn't explicitly account for factors such as age structure, competition, or density-dependent mortality.
-
Lack of stochasticity: The basic logistic model is deterministic, meaning it doesn't account for random fluctuations in population size. Stochastic models, which incorporate random variation, provide a more realistic representation of population dynamics.
To address these limitations, various modifications and extensions of the logistic equation have been developed:
-
Density-dependent models: These models incorporate the influence of population density on birth and death rates, allowing for more realistic representation of intraspecific competition.
-
Time-varying parameters: Models with time-varying parameters allow for the incorporation of environmental fluctuations and other external influences.
-
Stochastic logistic models: These models incorporate random variation, providing a more realistic representation of population fluctuations.
-
Stage-structured models: These models account for the different life stages within a population, recognizing that different age classes may have different growth rates and survival probabilities.
Conclusion
The logistic growth equation, despite its simplifying assumptions, provides a valuable framework for understanding and modeling population dynamics. Its applications span diverse fields, from ecology and epidemiology to economics and social sciences. While the basic model has limitations, modifications and extensions continue to refine its predictive power, offering more nuanced and realistic representations of population growth in various contexts. Understanding the strengths and weaknesses of the logistic model, along with its various adaptations, is crucial for accurately modeling and predicting population trends in a complex and ever-changing world. Future research will likely continue to explore and refine these models, incorporating more complex interactions and incorporating data-driven approaches to improve their accuracy and predictive capabilities.
Latest Posts
Latest Posts
-
Color Mass Shape And Density Are All Examples Of What
May 11, 2025
-
The Humoral Immune Response Depends On Which Cells
May 11, 2025
-
How Do Fish Eggs Get Fertilized
May 11, 2025
-
Calculating Heat Of Reaction From Constant Pressure Calorimetry Data
May 11, 2025
-
Are Chloroplasts Part Of The Endomembrane System
May 11, 2025
Related Post
Thank you for visiting our website which covers about According To The Logistic Growth Equation . We hope the information provided has been useful to you. Feel free to contact us if you have any questions or need further assistance. See you next time and don't miss to bookmark.