Alpha Value For 95 Confidence Interval
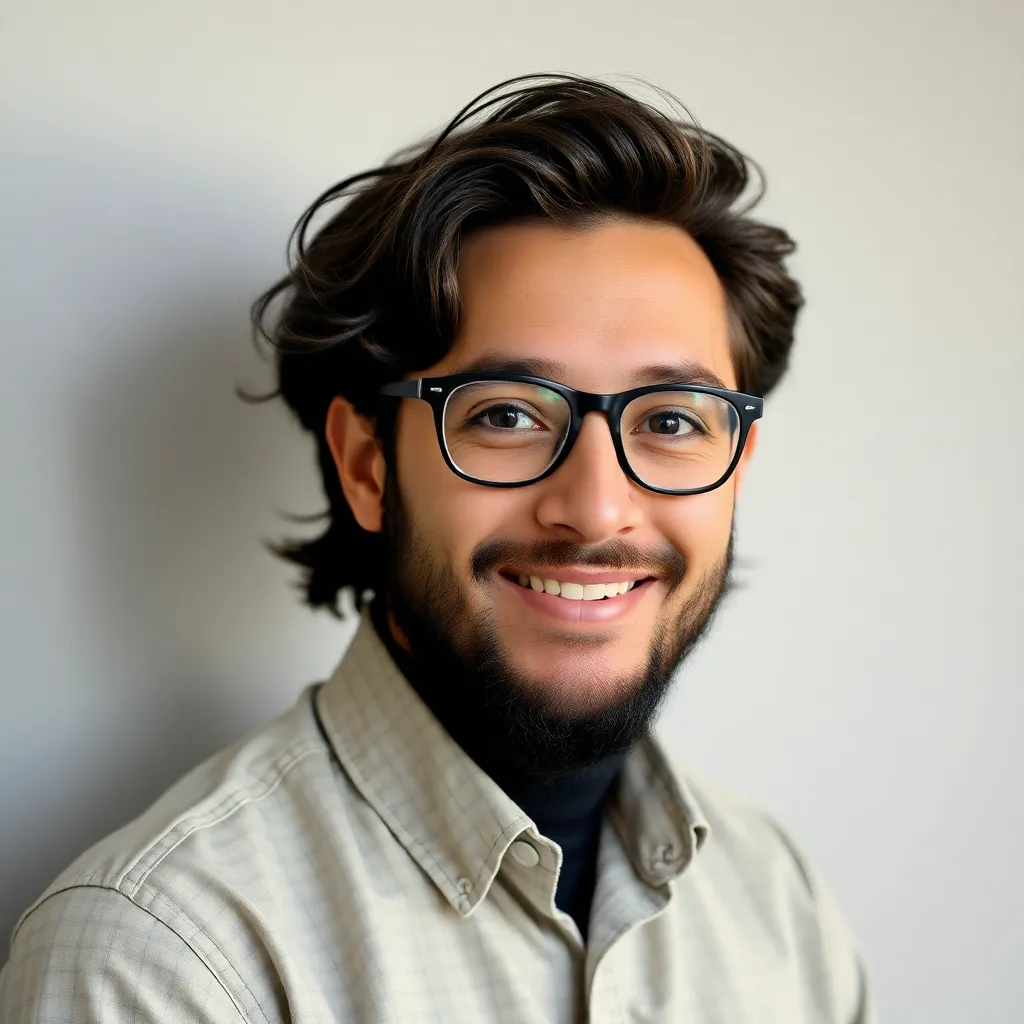
Muz Play
May 10, 2025 · 6 min read
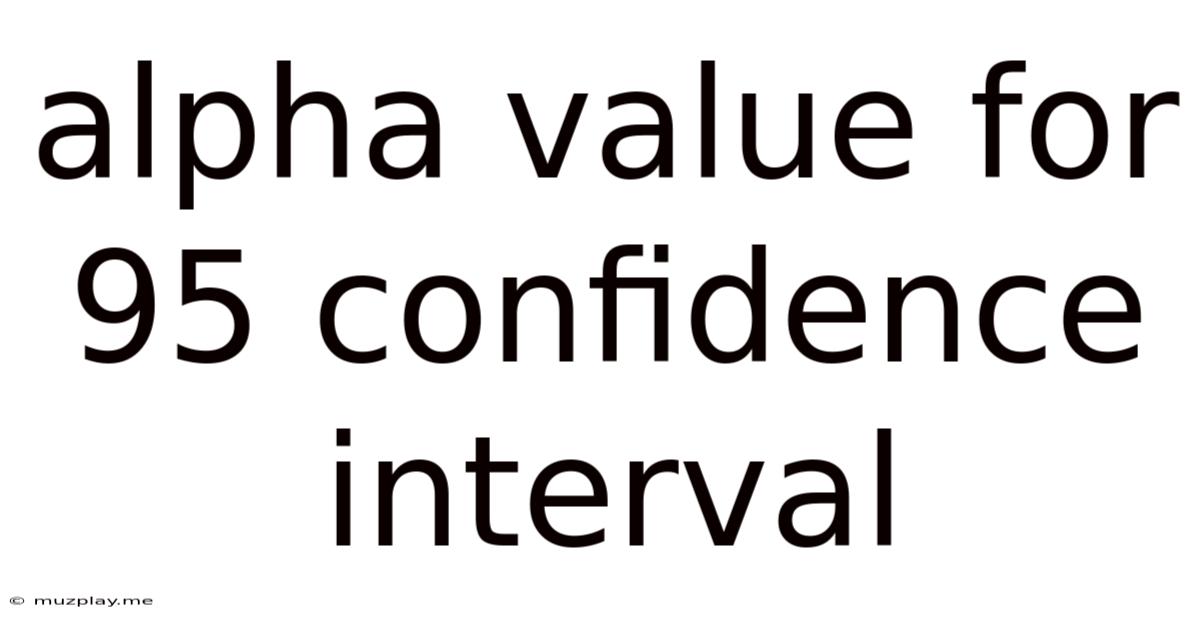
Table of Contents
Understanding the Alpha Value for a 95% Confidence Interval
The alpha value (α) is a critical concept in statistics, particularly when constructing confidence intervals. It represents the probability of making a Type I error – rejecting a true null hypothesis. In the context of a 95% confidence interval, understanding alpha is key to interpreting the results and making informed decisions. This comprehensive guide will delve into the meaning of alpha, its relationship with the confidence level, how it's used in calculating confidence intervals, and its implications for different statistical tests.
What is Alpha (α) and Why is it Important?
The alpha value, often expressed as a decimal (e.g., 0.05), signifies the significance level of a statistical test. It defines the probability of rejecting the null hypothesis when it is actually true. In simpler terms, it's the risk you're willing to take of being wrong when concluding there's a significant effect or difference.
Why is this important for a 95% confidence interval? A 95% confidence interval means we are 95% confident that the true population parameter (e.g., mean, proportion) lies within the calculated interval. This implies a 5% chance (1-0.95 = 0.05) that the true parameter falls outside the interval. This 5% chance directly corresponds to our alpha value (α = 0.05).
The Relationship Between Alpha and Confidence Level
The alpha value and the confidence level are inversely related. They always add up to 1 (or 100%). For example:
- 95% Confidence Interval: α = 1 - 0.95 = 0.05
- 99% Confidence Interval: α = 1 - 0.99 = 0.01
- 90% Confidence Interval: α = 1 - 0.90 = 0.10
The choice of alpha depends on the context and the consequences of making a Type I error. A lower alpha (e.g., 0.01 for a 99% confidence interval) reduces the probability of a Type I error but increases the probability of a Type II error (failing to reject a false null hypothesis). Conversely, a higher alpha increases the chance of a Type I error but reduces the chance of a Type II error.
Calculating Confidence Intervals: The Role of Alpha
The alpha value plays a crucial role in determining the critical value used to calculate a confidence interval. The critical value is a z-score or t-score that defines the boundaries of the confidence interval.
1. Z-scores (for large samples): For large sample sizes (generally n ≥ 30), the standard normal distribution (Z-distribution) is used. The critical z-score for a 95% confidence interval (α = 0.05) is found by looking up the value corresponding to 1 - α/2 = 0.975 in a standard normal table or using statistical software. This value is approximately 1.96. This means that 95% of the area under the standard normal curve lies between -1.96 and 1.96.
2. T-scores (for small samples): For smaller sample sizes (n < 30), the t-distribution is more appropriate. The critical t-score depends on both the alpha value and the degrees of freedom (df = n - 1). The degrees of freedom account for the loss of information due to estimating the sample mean from the sample data. For a 95% confidence interval, you would look up the t-score corresponding to α/2 = 0.025 and the relevant degrees of freedom in a t-table or use statistical software.
The Formula: The general formula for a confidence interval for a population mean (μ) is:
Confidence Interval = Sample Mean ± (Critical Value) * (Standard Error)
Where the standard error is the standard deviation of the sample divided by the square root of the sample size.
Implications of Alpha for Different Statistical Tests
The choice of alpha influences the outcome and interpretation of various statistical tests, including:
Hypothesis Testing:
In hypothesis testing, the alpha value sets the threshold for rejecting the null hypothesis. If the p-value (the probability of observing the obtained results if the null hypothesis were true) is less than alpha, the null hypothesis is rejected. For example, with α = 0.05, a p-value of 0.04 would lead to rejection of the null hypothesis, while a p-value of 0.06 would not.
Regression Analysis:
In regression analysis, alpha is used to determine the significance of individual regression coefficients. If the p-value associated with a coefficient is less than alpha, the coefficient is considered statistically significant, indicating a meaningful relationship between the predictor and response variables.
ANOVA (Analysis of Variance):
In ANOVA, alpha is used to determine if there are significant differences between the means of different groups. The F-statistic is compared to a critical F-value determined using alpha and the degrees of freedom.
Chi-Square Tests:
In chi-square tests, alpha is used to determine if there's a significant association between two categorical variables. The chi-square statistic is compared to a critical chi-square value determined using alpha and the degrees of freedom.
Choosing the Right Alpha Value
The selection of an appropriate alpha value is a crucial decision in statistical analysis and depends heavily on the context and consequences of potential errors. Several factors should be considered:
-
The cost of Type I and Type II errors: A Type I error (false positive) might lead to unnecessary actions or expenses, while a Type II error (false negative) might lead to missed opportunities or harmful consequences. The relative costs of these errors should guide the choice of alpha.
-
The field of study: Some fields, such as medicine and drug development, typically use stricter alpha levels (e.g., 0.01 or even 0.001) due to the high stakes involved. Other fields might be more lenient.
-
Sample size: Larger sample sizes allow for more precise estimations, potentially enabling the use of a stricter alpha level without significantly increasing the risk of a Type II error.
-
Prior research and expectations: If there's prior evidence supporting a particular effect, a stricter alpha level might be appropriate.
Interpreting Confidence Intervals in Relation to Alpha
A 95% confidence interval, with α = 0.05, does not mean that there is a 95% probability that the true population parameter lies within the calculated interval. Instead, it means that if we were to repeatedly sample from the population and construct many 95% confidence intervals, 95% of those intervals would contain the true population parameter. The remaining 5% (corresponding to alpha) would not.
Conclusion
The alpha value is a cornerstone of statistical inference, playing a pivotal role in constructing confidence intervals and conducting hypothesis tests. Understanding its meaning, its relationship with the confidence level, and the implications of different alpha values is essential for proper interpretation of statistical results and informed decision-making. The choice of alpha should be carefully considered based on the context, potential errors, and the balance between Type I and Type II error risks. By thoughtfully selecting and interpreting the alpha value, researchers can enhance the reliability and validity of their findings. Remember that while a 95% confidence interval offers a high degree of confidence, it doesn't guarantee that the true parameter lies within the interval; it simply reflects the long-run probability of the procedure accurately capturing the parameter.
Latest Posts
Latest Posts
-
What Does The Process Of Post Translational Control Refer To
May 10, 2025
-
A Symbiotic Relationship In Which Both Members Benefit Is Called
May 10, 2025
-
An Acid Is A Substance That
May 10, 2025
-
What Helps A Plant Cell Maintain Its Shape
May 10, 2025
-
What Is The Main Source Of Free External Dna
May 10, 2025
Related Post
Thank you for visiting our website which covers about Alpha Value For 95 Confidence Interval . We hope the information provided has been useful to you. Feel free to contact us if you have any questions or need further assistance. See you next time and don't miss to bookmark.