As The Sample Size Increases The Sample Mean Approaches The
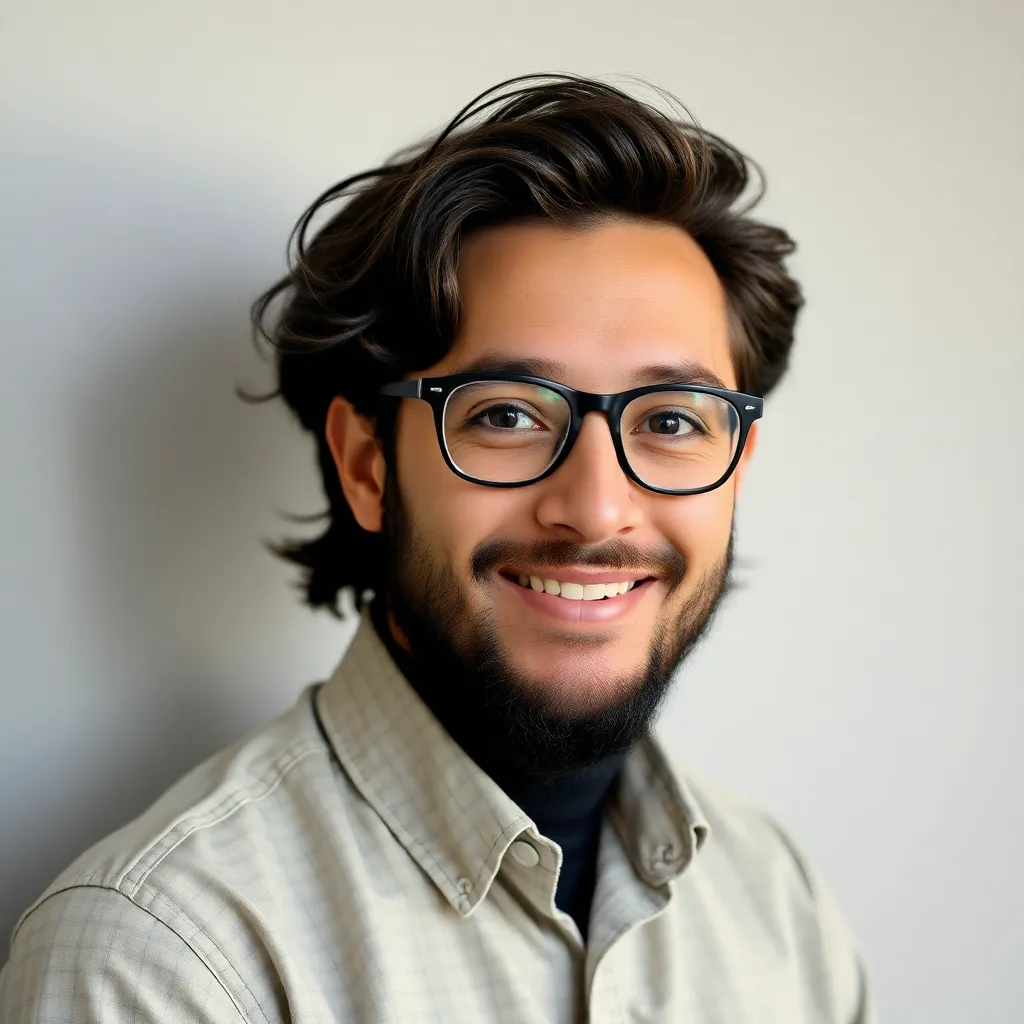
Muz Play
May 09, 2025 · 7 min read
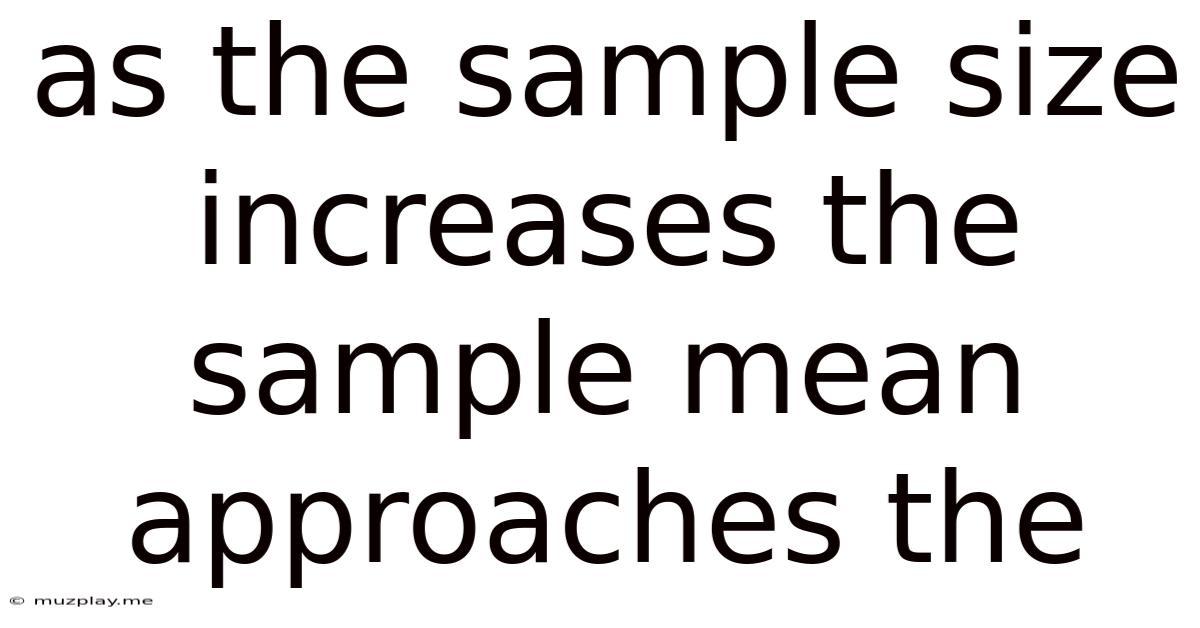
Table of Contents
As the Sample Size Increases, the Sample Mean Approaches the Population Mean: A Deep Dive into the Central Limit Theorem
The fundamental concept in statistics that governs how sample means relate to population means is the Central Limit Theorem (CLT). This theorem is incredibly powerful because it allows us to make inferences about a population based on the information gleaned from a sample, even if we don't know the population's true distribution. In essence, the CLT states that as the sample size increases, the distribution of the sample means approaches a normal distribution, regardless of the shape of the population distribution. This means the sample mean becomes a increasingly reliable estimator of the population mean. Let's delve deeper into this crucial statistical principle.
Understanding the Central Limit Theorem
The CLT doesn't simply say that the sample mean gets closer to the population mean; it describes the distribution of these sample means. Imagine repeatedly taking samples of a specific size from a population, calculating the mean of each sample, and then plotting those means. What the CLT tells us is that this distribution of sample means will, under certain conditions, approximate a normal distribution.
Key Aspects of the Central Limit Theorem:
-
Sample Size: The larger the sample size (n), the closer the distribution of sample means will be to a normal distribution. A general rule of thumb is that a sample size of 30 or more is often sufficient for the CLT to hold, even if the population distribution is non-normal. However, the closer the population distribution is to normal, the smaller the sample size required.
-
Independence: The samples must be independent. This means that the selection of one data point doesn't influence the selection of any other data point. This is crucial for accurate estimations.
-
Population Mean (μ) and Population Standard Deviation (σ): The distribution of sample means will have a mean equal to the population mean (μ) and a standard deviation (standard error) equal to the population standard deviation (σ) divided by the square root of the sample size (√n). This standard error represents the variability of the sample means.
-
Approximation to Normality: The CLT states that the distribution of sample means approaches a normal distribution. It doesn't say it becomes exactly normal, especially with small sample sizes from highly skewed populations. The approximation becomes increasingly accurate as the sample size grows.
The Implications of the CLT for Statistical Inference
The CLT is the cornerstone of many statistical procedures used for hypothesis testing and confidence interval estimation. Its implications are far-reaching:
-
Confidence Intervals: The CLT justifies the use of the normal distribution to construct confidence intervals for population means. A confidence interval provides a range of values within which the true population mean is likely to fall with a certain level of confidence (e.g., 95%). The width of this interval decreases as the sample size increases, reflecting increased precision.
-
Hypothesis Testing: Many statistical hypothesis tests rely on the assumption that the sampling distribution of the mean is approximately normal. This allows us to calculate p-values and make inferences about whether observed differences between groups or samples are statistically significant or likely due to chance.
-
Understanding Sampling Error: The CLT helps us understand sampling error – the difference between a sample statistic (like the sample mean) and the corresponding population parameter (like the population mean). By understanding this error, we can assess the reliability of our sample estimates.
Visualizing the CLT: A Simulation
Let's imagine a population with a distinctly non-normal distribution – say, an exponential distribution. If we repeatedly draw samples of different sizes (e.g., n=5, n=25, n=100) from this population and plot the distribution of the sample means, we would observe the following:
-
Small Sample Size (n=5): The distribution of sample means would be skewed, mirroring the original exponential distribution, although less so.
-
Medium Sample Size (n=25): The skewness would be reduced considerably, and the distribution would begin to resemble a bell-shaped curve.
-
Large Sample Size (n=100): The distribution would closely approximate a normal distribution, even though the original population was far from normal.
This demonstrates the power of the CLT: even with a non-normal population, the distribution of sample means converges towards normality as the sample size increases. This convergence makes it possible to employ techniques reliant on normality, despite the original data not conforming to a normal distribution.
Mathematical Formulation of the Central Limit Theorem
While the intuitive explanation is crucial, understanding the mathematical basis solidifies the concept. The CLT can be formally expressed as follows:
Let X₁, X₂, ..., Xₙ be a random sample of size n from a population with mean μ and variance σ². Then, as n approaches infinity, the distribution of the sample mean (X̄ = (ΣXᵢ)/n) approaches a normal distribution with mean μ and variance σ²/n.
This can be written as:
X̄ ~ N(μ, σ²/n) as n → ∞
This notation indicates that the sample mean (X̄) follows a normal distribution with a mean equal to the population mean (μ) and a variance equal to the population variance divided by the sample size (σ²/n).
Conditions for the Central Limit Theorem to Apply
While the CLT is remarkably robust, there are some conditions that ideally should be met for it to provide the most accurate approximation:
-
Random Sampling: The samples must be randomly selected from the population to ensure independence and avoid bias.
-
Independence: Observations within a sample should be independent of each other. This means the value of one observation doesn't influence the value of another. Violations of independence can occur in time series data or clustered data, requiring specific statistical techniques to address the correlation.
-
Finite Variance: The population from which the samples are drawn should have a finite variance (σ²). Distributions with infinite variance (like the Cauchy distribution) do not satisfy the CLT.
Practical Applications and Examples
The CLT's applications are widespread across various fields. Here are a few examples:
-
Quality Control: In manufacturing, the CLT is used to assess the quality of products by taking samples and estimating the mean and variability of a characteristic (e.g., weight, length).
-
Medical Research: Clinical trials often use the CLT to analyze the effectiveness of a new treatment by comparing the average outcome in the treatment group to the average outcome in a control group.
-
Finance: The CLT is used extensively in finance to model and analyze financial assets' returns, often using the assumption that asset returns are approximately normally distributed.
-
Opinion Polls: Polling organizations use the CLT to estimate the proportion of the population who support a particular candidate or policy by sampling a smaller subset of the population.
When the CLT Might Not Apply
While the CLT is generally very robust, it may not be entirely reliable under certain circumstances:
-
Extremely Small Sample Sizes: With very small sample sizes (significantly less than 30), the approximation to normality may be poor, especially if the population distribution is highly skewed.
-
Highly Skewed Populations: For populations with extreme skewness, a larger sample size may be needed to achieve a good approximation to normality. Transforming the data (e.g., using logarithms) might improve the situation.
-
Dependent Data: If the observations within the sample are not independent, the CLT may not hold. Specialized statistical methods are necessary to account for this dependence.
-
Non-finite Variance: The CLT does not apply to distributions with infinite variance, requiring alternative methods for inference.
Conclusion
The Central Limit Theorem is a cornerstone of statistical inference, providing a powerful framework for making inferences about population parameters based on sample data. It demonstrates that as the sample size increases, the distribution of sample means tends towards a normal distribution, regardless of the underlying population distribution. This allows statisticians to use normal distribution-based techniques for hypothesis testing and confidence interval estimation, even when dealing with non-normal data. However, it's crucial to remember that the CLT is an approximation, and its accuracy depends on factors like sample size and the nature of the population distribution. Understanding these limitations is essential for proper application and interpretation of statistical results. Always consider the assumptions of the CLT before applying it to any data analysis. By carefully considering sample size, independence, and the shape of the data, you can confidently harness the power of the CLT for reliable statistical inference.
Latest Posts
Latest Posts
-
Predicting Whether Simple Electrochemical Reactions Happen
May 11, 2025
-
Noted Example Of French Gothic Architecture
May 11, 2025
-
Select All The Characteristics Of Life
May 11, 2025
-
Multiunit Smooth Muscle Cells Are Stimulated
May 11, 2025
-
Determine Whether 2 Chloro 3 Methylbutane Contains A Chiral Center
May 11, 2025
Related Post
Thank you for visiting our website which covers about As The Sample Size Increases The Sample Mean Approaches The . We hope the information provided has been useful to you. Feel free to contact us if you have any questions or need further assistance. See you next time and don't miss to bookmark.