Choose The Quadratic Model For The Situation.
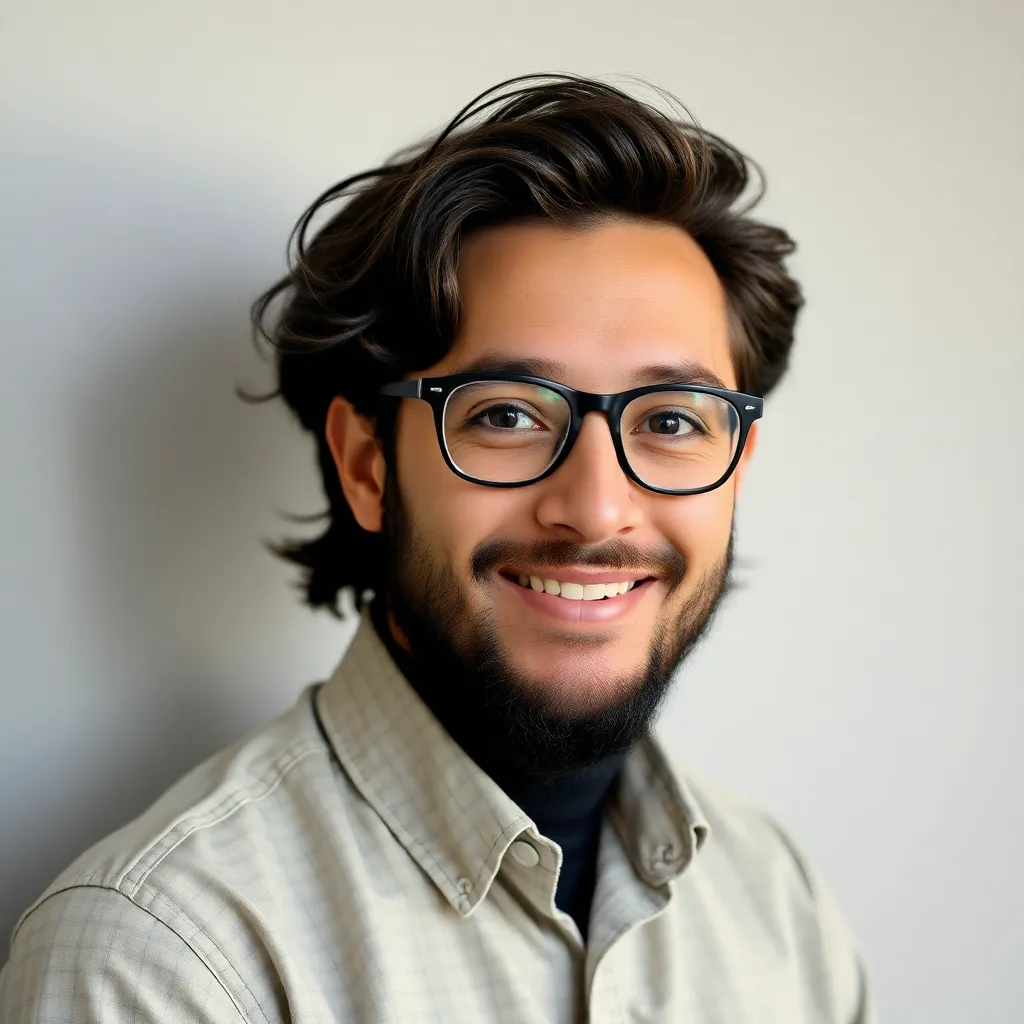
Muz Play
May 10, 2025 · 6 min read
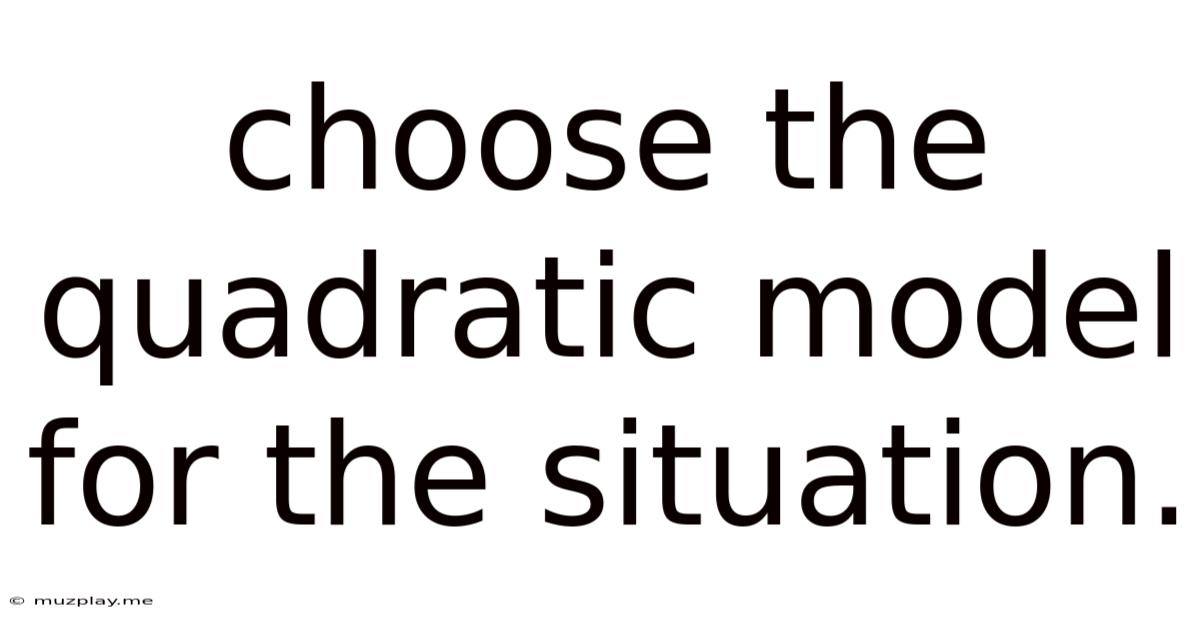
Table of Contents
Choosing the Quadratic Model for the Situation: A Comprehensive Guide
Quadratic models, represented by the equation y = ax² + bx + c, are powerful tools for modeling a wide range of real-world phenomena. Understanding when to choose a quadratic model over other types of functions is crucial for accurate representation and insightful analysis. This comprehensive guide explores various scenarios where a quadratic model proves most appropriate, detailing the characteristics to look for and offering practical examples.
Identifying Situations Suitable for Quadratic Modeling
Before diving into specific examples, let's establish the key characteristics that suggest a quadratic model is the most suitable choice:
1. Parabolic Relationships
The most defining feature of a quadratic function is its parabolic graph. If the data points you're working with visually resemble a parabola – a symmetrical U-shaped curve – then a quadratic model is a strong candidate. This symmetrical nature indicates a relationship where an initial increase or decrease is followed by a reversal in the trend.
2. Rate of Change
Examine the rate at which the dependent variable (y) changes relative to the independent variable (x). In a quadratic relationship, the rate of change itself changes at a constant rate. This means that the slope of the curve is not constant; it increases or decreases linearly. A simple way to visualize this is to observe whether the differences between consecutive y-values are themselves changing at a constant rate.
3. Maximum or Minimum Points
Quadratic functions always have either a maximum or minimum point (vertex), a crucial characteristic to consider. If your data suggests a peak or trough, representing a maximum or minimum value, a quadratic model can accurately capture this turning point. Many real-world situations involve optimization problems, searching for a maximum profit, minimum cost, or optimal trajectory, all naturally modeled by quadratic equations.
4. Second Differences
A powerful mathematical test for quadratic relationships lies in examining the second differences. Calculate the differences between consecutive y-values (first differences). Then, calculate the differences between these first differences (second differences). If the second differences are approximately constant, it strongly suggests a quadratic model. This method is particularly useful when dealing with numerical data.
Real-World Applications of Quadratic Models
The versatility of quadratic models extends to numerous fields. Here are some examples illustrating their applicability:
1. Projectile Motion
Perhaps the most classic example is the trajectory of a projectile under the influence of gravity. Ignoring air resistance, the height of a projectile over time follows a parabolic path, perfectly described by a quadratic equation. Factors like initial velocity and launch angle influence the specific parameters (a, b, and c) of the equation.
Example: A ball thrown upwards will initially rise, reach a maximum height (vertex of the parabola), and then fall back to the ground. This trajectory can be accurately modeled using a quadratic equation, allowing for calculations of maximum height, time of flight, and range.
2. Area Optimization
Many optimization problems involve maximizing or minimizing area. Consider designing a rectangular enclosure with a fixed perimeter. The area of the rectangle is a quadratic function of its dimensions, enabling the determination of the dimensions that maximize the enclosed area (a square in this case).
Example: A farmer wants to enclose a rectangular field using a given length of fence. The area of the field is a quadratic function of the length and width. Finding the maximum area involves determining the vertex of the corresponding parabola.
3. Revenue and Cost Analysis
In business, the relationship between the price of a product and the resulting revenue often follows a quadratic pattern. Initially, increasing price leads to increased revenue, but beyond a certain point, higher prices result in decreased demand and lower revenue. This creates a parabolic relationship with a maximum revenue point. Similarly, cost functions can sometimes be approximated by quadratic models, particularly when considering economies of scale or diminishing returns.
Example: A company analyzes the relationship between the price of its product and the total revenue generated. A quadratic model can help determine the optimal price to maximize revenue.
4. Engineering and Physics
Beyond projectile motion, quadratic models find extensive applications in various engineering and physics disciplines. These include:
- Bridge Design: The parabolic shape of many suspension bridges is inherently linked to quadratic functions. The curve helps distribute weight effectively and optimize structural integrity.
- Signal Processing: Quadratic models are used in analyzing and processing signals, particularly in identifying and filtering noise.
- Optics: The path of light rays in certain optical systems can be modeled using quadratic equations.
5. Biological and Ecological Modeling
While less frequent than in physics and engineering, quadratic models can find application in biological and ecological studies. For example, the growth of a certain population might initially exhibit exponential growth, but eventually level off due to resource constraints. A modified quadratic model could capture this initial rapid growth followed by a slowdown.
Example: Modeling the population growth of a species where the growth rate is influenced by both birth and death rates that change depending on population density.
Choosing the Right Model: Comparing Quadratic with Other Functions
While quadratic models excel in capturing parabolic relationships, it's crucial to compare them with other function types before making a decision. Here's a brief comparison:
- Linear Models (y = mx + c): Linear models describe situations with a constant rate of change. If your data points show a consistent slope, a linear model is more appropriate.
- Exponential Models (y = ab<sup>x</sup>): Exponential models depict situations with rapid, multiplicative growth or decay. If your data shows a consistently increasing or decreasing percentage change, an exponential model might be better suited.
- Polynomial Models (higher order): For more complex relationships with multiple turning points, higher-order polynomial models (cubic, quartic, etc.) could be necessary. However, the principle of parsimony suggests using the simplest model that adequately fits the data; more complex models increase the risk of overfitting.
Practical Steps for Implementing a Quadratic Model
- Data Collection: Gather a sufficient amount of reliable data relevant to the situation you're modeling.
- Data Visualization: Create a scatter plot of your data. This visual representation will give you an initial indication of whether a quadratic model might be suitable.
- Regression Analysis: Use statistical software or programming tools (e.g., Excel, R, Python) to perform quadratic regression. This process involves finding the best-fitting quadratic equation (values of a, b, and c) that minimizes the difference between the model's predictions and the actual data points.
- Model Evaluation: Assess the goodness of fit of your quadratic model using metrics like R-squared, which indicates the proportion of variance in the dependent variable explained by the model. A higher R-squared value generally indicates a better fit. Also, check for any systematic deviations between the model predictions and the data.
- Interpretation and Application: Once you have a satisfactory model, interpret the coefficients (a, b, and c) in the context of the situation you're modeling. Use the model to make predictions, understand trends, and gain insights into the underlying process.
Conclusion
Choosing the appropriate model is a crucial step in any quantitative analysis. Quadratic models offer a versatile tool for understanding and representing numerous real-world phenomena characterized by parabolic relationships, rate of change variations, and maximum/minimum points. By carefully examining the characteristics of your data and comparing quadratic models to other functional forms, you can effectively leverage this powerful technique for accurate modeling and insightful decision-making. Remember to always visually inspect your data, perform rigorous statistical analysis, and interpret the results within the relevant context.
Latest Posts
Latest Posts
-
Do Living Things Use Heat As An Energy Source
May 11, 2025
-
What Is The Volume Of The Solid In The Figure
May 11, 2025
-
How Does A Strong Acid Differ From A Weak Acid
May 11, 2025
-
Is Water A Homogeneous Or Heterogeneous Mixture
May 11, 2025
-
Definition Of Skeleton Equation In Chemistry
May 11, 2025
Related Post
Thank you for visiting our website which covers about Choose The Quadratic Model For The Situation. . We hope the information provided has been useful to you. Feel free to contact us if you have any questions or need further assistance. See you next time and don't miss to bookmark.