Conditions For Goodness Of Fit Test
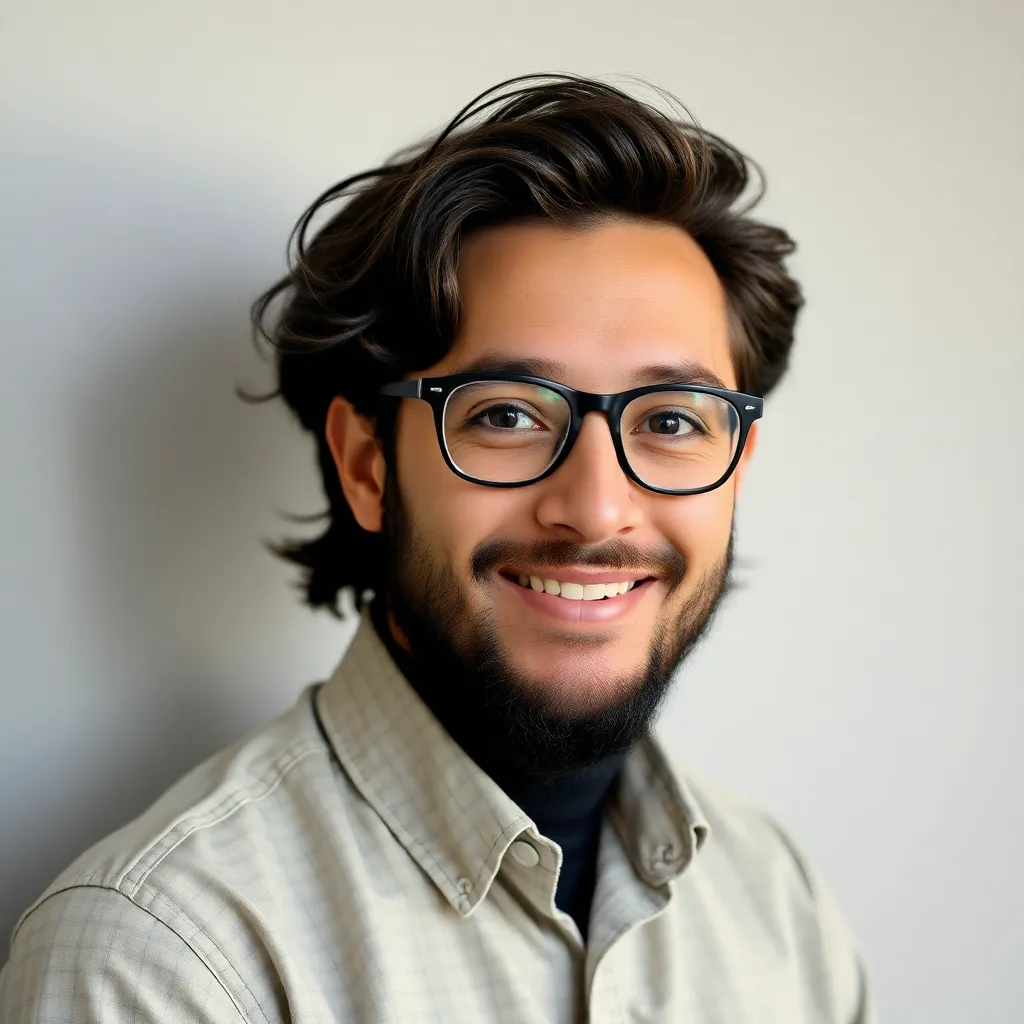
Muz Play
May 09, 2025 · 6 min read
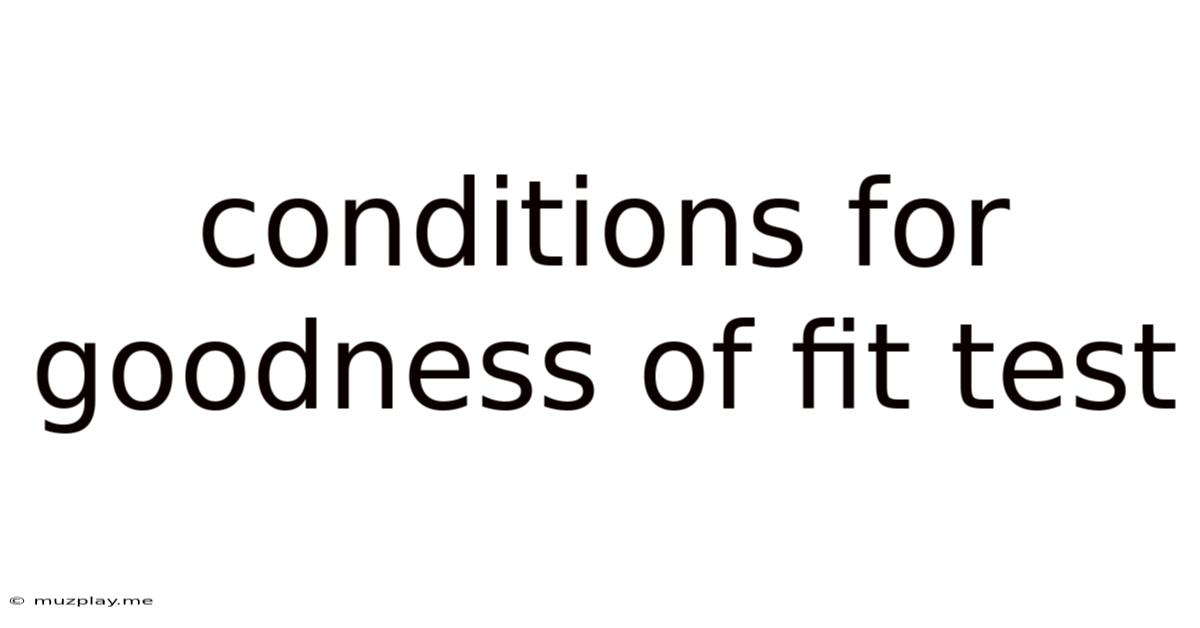
Table of Contents
Conditions for Goodness of Fit Test: A Comprehensive Guide
The goodness-of-fit test is a statistical hypothesis test used to determine whether a sample data distribution conforms to a hypothesized distribution. Understanding the conditions under which this test is valid is crucial for accurate and reliable results. Misinterpreting these conditions can lead to flawed conclusions and misleading interpretations of your data. This comprehensive guide delves into the essential conditions for a valid goodness-of-fit test, ensuring you can apply this powerful statistical tool effectively.
Key Conditions for a Valid Goodness-of-Fit Test
Before diving into the specifics, let's outline the crucial conditions that must be met for a reliable goodness-of-fit test:
-
Random Sampling: The data must be a random sample from the population of interest. Non-random sampling introduces bias, significantly affecting the test's validity and potentially leading to inaccurate conclusions. Randomness ensures the sample is representative of the population, making the inferences drawn from the test more generalizable.
-
Independent Observations: Each observation in the sample must be independent of the others. This means that the value of one observation should not influence the value of any other observation. Violation of independence often occurs in time series data or clustered data. Appropriate techniques, such as accounting for autocorrelation or using clustered standard errors, might be needed to address this issue.
-
Expected Frequencies: The expected frequencies for each category in the hypothesized distribution must be sufficiently large. This is crucial to ensure the validity of the chi-square approximation used in the goodness-of-fit test. A common rule of thumb is that no expected frequency should be less than 5. If this condition is violated, alternative methods, such as combining categories or using Fisher's exact test (especially for small samples), are necessary.
-
Categorical Data: The goodness-of-fit test is designed for categorical data. The data must be categorized into distinct groups or classes. Continuous data needs to be discretized (grouped into intervals) before applying the test. The choice of intervals can impact the results, so careful consideration is required.
-
Hypothesized Distribution: You must have a specific hypothesized distribution to compare your sample data against. This could be a theoretical distribution like a normal distribution, Poisson distribution, uniform distribution, or a distribution derived from prior knowledge or expectations. The test assesses how well your observed data aligns with this pre-defined distribution.
Detailed Examination of Each Condition
Let's now delve into a more detailed explanation of each condition:
1. Random Sampling: The Foundation of Inference
The cornerstone of any statistical inference is random sampling. If your sample is not representative of the population, your results will be biased and unreliable. Random sampling ensures every member of the population has an equal chance of being selected for the sample. This minimizes selection bias and increases the generalizability of your findings.
Techniques like simple random sampling, stratified random sampling, and cluster random sampling are employed to achieve randomness. Carefully documenting your sampling method is critical for transparency and reproducibility. If your sampling method introduces systematic bias, the goodness-of-fit test results will be severely compromised.
2. Independent Observations: Avoiding Influence
Independence of observations is crucial for the validity of the chi-square test statistic. If observations are correlated, the test's assumptions are violated, leading to inaccurate p-values and potentially incorrect conclusions.
Examples of situations where independence might be violated:
- Repeated measurements on the same individual: Measurements taken over time on the same subject are likely to be correlated.
- Spatial clustering: Data from geographically close locations may exhibit spatial autocorrelation.
- Family data: Data collected from members of the same family may show familial correlation.
Addressing non-independence often requires specialized statistical techniques beyond the standard goodness-of-fit test. These techniques might involve adjusting the standard errors or using more advanced models that account for the correlation structure in the data.
3. Expected Frequencies: Maintaining Accuracy
The expected frequencies are the number of observations expected in each category under the hypothesized distribution. The chi-square test relies on an approximation that becomes increasingly accurate as the expected frequencies get larger. The rule of thumb of no expected frequency less than 5 is widely accepted, though some sources suggest a more stringent criterion of no expected frequency less than 10. The consequences of violating this condition include:
- Inflated Type I error rate: An increased likelihood of rejecting the null hypothesis when it is actually true.
- Inaccurate p-values: The p-value, which represents the probability of observing the data if the null hypothesis is true, becomes less reliable.
If the expected frequencies are too low, combining categories, using exact tests (like Fisher's exact test for 2x2 contingency tables), or collecting more data might be necessary.
4. Categorical Data: The Nature of the Test
The goodness-of-fit test is specifically designed for categorical data, which represents observations classified into distinct categories. If your data is continuous, you must first group it into meaningful intervals or categories.
The process of categorizing continuous data involves:
- Choosing appropriate intervals: The width and number of intervals should be carefully considered. Too few intervals might obscure important details, while too many intervals might lead to sparse cell counts and violate the expected frequency condition.
- Defining clear boundaries: The boundaries between intervals should be unambiguous to avoid ambiguity in the classification of observations.
The choice of categorization can affect the results of the goodness-of-fit test. Sensitivity analysis, exploring different categorization schemes, can help assess the robustness of your conclusions.
5. Hypothesized Distribution: A Clear Target
The goodness-of-fit test requires a clearly defined hypothesized distribution to compare your observed data against. This distribution might be based on:
- Theoretical considerations: For example, you might hypothesize that your data follows a normal distribution or a Poisson distribution based on theoretical arguments or previous research.
- Prior knowledge or expectations: Your prior knowledge about the process generating the data might suggest a specific distribution.
- Empirical data: A previous dataset or a pilot study might provide an empirical distribution to serve as a basis for comparison.
Clearly stating your hypothesized distribution is crucial for the interpretation of your results. The test assesses the compatibility between your observed data and this specific hypothesized distribution, not other possible distributions.
Consequences of Violating Conditions
Ignoring or violating these conditions can have significant repercussions:
- Biased results: Non-random sampling or correlated observations introduce bias, leading to inaccurate conclusions.
- Inflated Type I error rate: Violating the expected frequency condition increases the chance of incorrectly rejecting the null hypothesis.
- Inaccurate p-values: Violated assumptions lead to unreliable p-values, making it difficult to draw valid inferences.
- Misleading interpretations: Incorrect conclusions can have serious consequences, particularly in applications involving decision-making or policy implications.
Conclusion: Ensuring Valid Goodness-of-Fit Tests
The goodness-of-fit test is a powerful tool for assessing the fit between observed data and a hypothesized distribution. However, its validity hinges on satisfying the conditions outlined above. Careful consideration of random sampling, independence of observations, expected frequencies, the nature of the data, and the specification of the hypothesized distribution are paramount. By adhering to these conditions, you can ensure the accuracy and reliability of your results, enabling you to draw valid conclusions and make informed decisions based on your data analysis. Remember to always document your methodology meticulously, allowing for transparency and reproducibility of your findings. Understanding these conditions empowers you to use the goodness-of-fit test effectively and confidently, enhancing the rigor and validity of your statistical analyses.
Latest Posts
Latest Posts
-
Apparent Enlargement Of An Object Is Known As
May 09, 2025
-
The Atomic Mass Of An Atom Is Determined By
May 09, 2025
-
Do Limits Exist At Vertical Asymptotes
May 09, 2025
-
Ejemplos De Organizaciones Sin Fines De Lucro
May 09, 2025
-
Predict The Relative Solubility Of The Following Substances In Water
May 09, 2025
Related Post
Thank you for visiting our website which covers about Conditions For Goodness Of Fit Test . We hope the information provided has been useful to you. Feel free to contact us if you have any questions or need further assistance. See you next time and don't miss to bookmark.