Confidence Interval Examples In Real Life
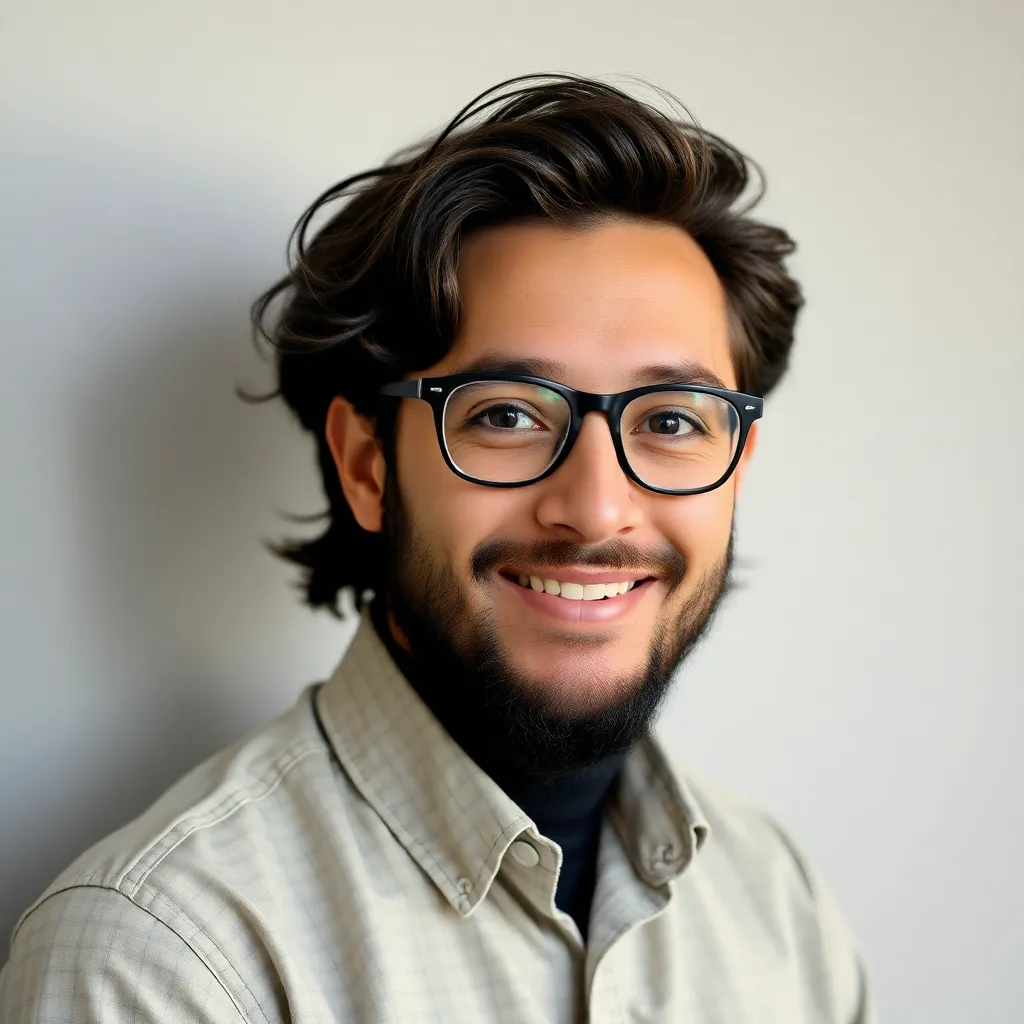
Muz Play
May 11, 2025 · 7 min read
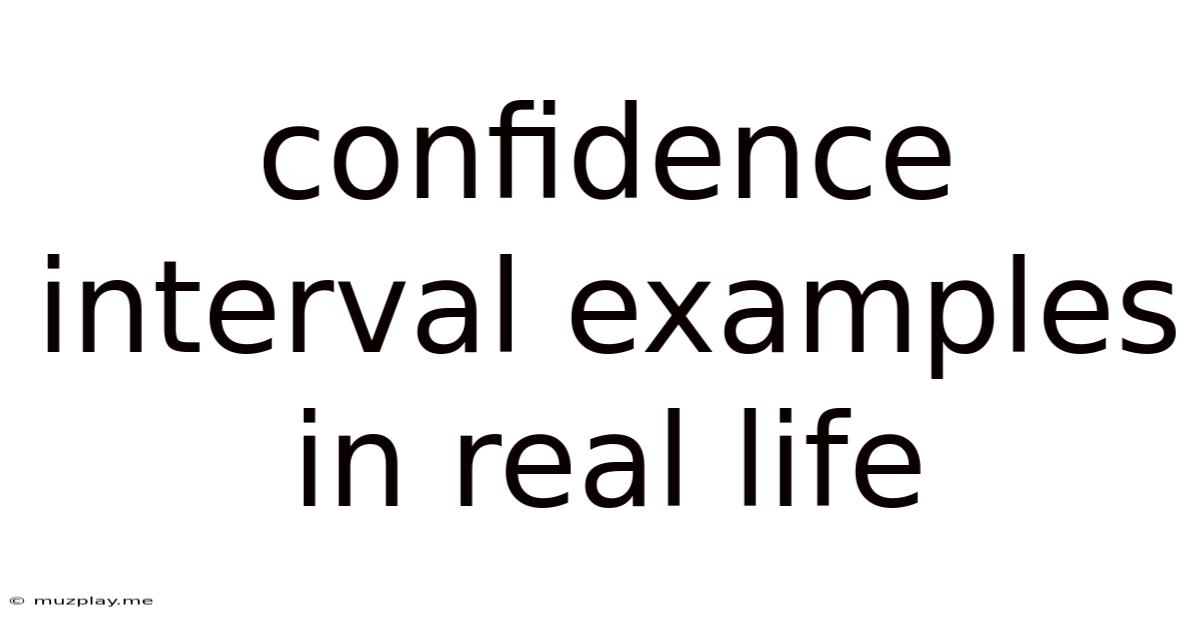
Table of Contents
Confidence Interval Examples in Real Life: Understanding the Margin of Error
Confidence intervals are a cornerstone of statistical inference, providing a range of values within which a population parameter is likely to lie. While the concept might seem abstract, confidence intervals are used extensively in real-life situations, impacting decisions across various fields. This article will explore numerous examples of confidence intervals in action, illustrating their practical application and significance. Understanding confidence intervals allows us to interpret data more effectively and make more informed decisions based on the inherent uncertainty present in any data set.
What is a Confidence Interval?
Before diving into real-life examples, let's briefly review the core concept. A confidence interval provides a range of plausible values for a population parameter, such as the mean or proportion. This range is accompanied by a confidence level, typically 95% or 99%, indicating the probability that the true population parameter falls within the calculated interval. The higher the confidence level, the wider the interval. This is because a higher confidence level requires a larger margin of error to encompass more potential values.
The formula for a confidence interval typically involves a point estimate (like the sample mean), a margin of error, and a critical value derived from the appropriate probability distribution (often the z-distribution or t-distribution). The margin of error accounts for the inherent uncertainty in using a sample to estimate the population parameter.
Real-Life Examples Across Diverse Fields
The applications of confidence intervals are vast and varied. Let's explore some compelling examples across different sectors:
1. Public Health and Epidemiology: Tracking Disease Prevalence
Imagine a public health agency investigating the prevalence of a new virus. They collect a random sample of individuals and test them for the virus. Based on the sample proportion infected, they calculate a 95% confidence interval for the true population prevalence. This interval might be, for example, 5% to 10%. This means they are 95% confident that the true percentage of infected individuals in the population lies somewhere between 5% and 10%. This information is crucial for resource allocation, public health messaging, and the development of effective control measures. A narrower confidence interval indicates more precise information, while a wider interval highlights greater uncertainty. Factors like sample size significantly influence the width of the interval. A larger sample size generally leads to a narrower interval, providing more precise estimates.
2. Manufacturing and Quality Control: Ensuring Product Standards
In manufacturing, confidence intervals are vital for quality control. Suppose a factory produces bolts with a specified diameter. They randomly sample bolts from the production line and measure their diameters. By calculating a confidence interval for the mean diameter, they can assess whether the production process is meeting the required specifications. If the confidence interval falls entirely within the acceptable range, they can be confident that the production process is under control. However, if the interval extends beyond the acceptable range, it suggests a need to investigate and adjust the production process to maintain quality standards. This continuous monitoring using confidence intervals prevents the widespread production of defective products.
3. Marketing and Market Research: Gauging Consumer Preferences
Confidence intervals are indispensable tools in market research. Consider a company launching a new product. They conduct a survey to gauge consumer interest. Using the sample data, they can calculate a confidence interval for the proportion of consumers who would purchase the product. This information informs marketing strategies, sales projections, and investment decisions. For example, if the 95% confidence interval for the purchase intention is 30% to 40%, the company can be reasonably confident that somewhere between 30% and 40% of the population will buy the product. This understanding allows for more accurate resource allocation and marketing campaign planning.
4. Political Polling and Election Forecasting: Predicting Outcomes
Political polls rely heavily on confidence intervals. Polling organizations collect data from a sample of voters to estimate the proportion of voters who support a particular candidate. They use confidence intervals to present their findings, acknowledging the inherent uncertainty due to sampling variability. For example, a poll might report that Candidate A has 45% support, with a 95% confidence interval of 40% to 50%. This means the pollsters are 95% confident that the true level of support for Candidate A is somewhere between 40% and 50%. This is crucial information for campaign strategists, media outlets, and voters. The margin of error explicitly highlights the limitations of the sample data in predicting the overall election outcome.
5. Environmental Science: Monitoring Pollution Levels
In environmental science, confidence intervals are used to assess pollution levels. For instance, researchers might measure the concentration of a pollutant in a lake's water samples. They calculate a confidence interval for the average pollutant concentration, helping determine if the levels exceed safety standards. A narrow confidence interval indicates a more precise measurement of the pollution levels, while a wider interval reflects greater uncertainty. This information is essential for environmental protection agencies to make informed decisions about pollution control and remediation strategies.
6. Medical Research and Clinical Trials: Evaluating Treatment Efficacy
Clinical trials frequently employ confidence intervals to assess the effectiveness of new treatments. Researchers compare the outcomes of a treatment group with a control group. They calculate a confidence interval for the difference in the means (or proportions) of the two groups. This interval determines whether the observed difference is statistically significant or simply due to chance. For example, a confidence interval for the difference in blood pressure between a treatment group and a control group could help determine if the treatment is truly effective in lowering blood pressure. A confidence interval that does not include zero would suggest a statistically significant effect. This rigorous approach ensures the safety and effectiveness of new medical treatments before widespread implementation.
7. Finance and Investment: Assessing Investment Returns
In finance, confidence intervals are used to estimate investment returns. Investors use historical data to estimate the average return of an investment, along with its variability. They often use confidence intervals to present a range of plausible future returns, highlighting the risks and uncertainties associated with the investment. For instance, an investor might report that the expected return of a specific stock is 8%, with a 95% confidence interval of 2% to 14%. This implies that the investor is 95% confident that the actual return will fall within this range. This provides a realistic expectation of potential returns, assisting investors in making informed decisions.
8. Education: Evaluating Teaching Methods
Confidence intervals can assess the effectiveness of different teaching methods. Educators might compare the test scores of students taught using two different methods. By calculating a confidence interval for the difference in mean scores, they can determine whether one method consistently leads to better student performance than the other. A confidence interval not containing zero suggests a statistically significant difference in the effectiveness of the teaching methods. This evidence-based approach informs curriculum development and pedagogical practices.
Factors Affecting Confidence Interval Width
Several factors influence the width of a confidence interval:
- Sample size: Larger sample sizes lead to narrower confidence intervals, providing more precise estimates.
- Confidence level: Higher confidence levels (e.g., 99% vs. 95%) result in wider intervals.
- Population variability: Higher population variability leads to wider intervals.
- Sampling method: A well-designed sampling method minimizes bias and leads to more accurate and narrower intervals.
Conclusion: The Importance of Understanding Confidence Intervals
Confidence intervals are not merely a statistical tool; they are an essential component of informed decision-making across numerous disciplines. By understanding how confidence intervals work and their implications, we can interpret data more critically, acknowledge uncertainty, and make more robust conclusions. The examples presented illustrate the versatility and practical significance of confidence intervals in navigating the complexities of real-world data analysis. Their use is not limited to the examples presented but extends to virtually any field involving data collection and analysis where uncertainty needs to be quantified and understood. Understanding confidence intervals empowers us to make decisions based on reliable and statistically sound information.
Latest Posts
Latest Posts
-
Write The Equations In Cylindrical Coordinates
May 12, 2025
-
Defining Describing Demonstrating And Explaining Are Methods Of
May 12, 2025
-
The First Amino Acid Enters Through The A Site
May 12, 2025
-
The Mean Is Larger Than The Median When
May 12, 2025
-
In Which Phase Of Mitosis Do Spindle Microtubules Shorten
May 12, 2025
Related Post
Thank you for visiting our website which covers about Confidence Interval Examples In Real Life . We hope the information provided has been useful to you. Feel free to contact us if you have any questions or need further assistance. See you next time and don't miss to bookmark.