Confidence Interval For Slope Of Regression Line Formula
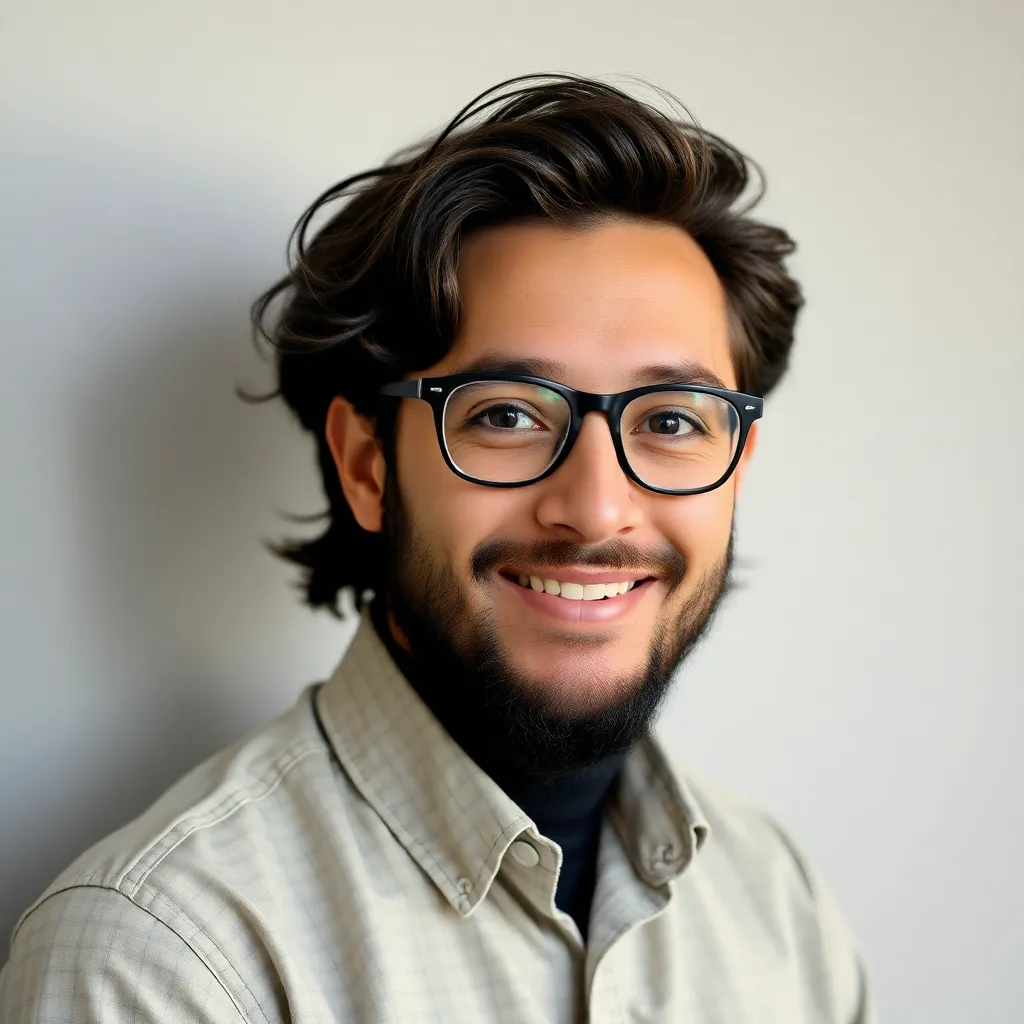
Muz Play
Apr 19, 2025 · 6 min read

Table of Contents
Confidence Interval for the Slope of a Regression Line: A Comprehensive Guide
Understanding the confidence interval for the slope of a regression line is crucial for interpreting the strength and reliability of your statistical model. This article provides a comprehensive guide, walking you through the formula, its interpretation, assumptions, and practical applications. We will explore the nuances of this statistical concept, helping you confidently analyze your regression results.
What is a Regression Line and its Slope?
Before diving into confidence intervals, let's establish a foundational understanding of linear regression. Linear regression aims to model the relationship between a dependent variable (Y) and one or more independent variables (X). The model assumes a linear relationship, expressed as:
Y = β₀ + β₁X + ε
Where:
- Y is the dependent variable.
- X is the independent variable.
- β₀ is the y-intercept (the value of Y when X is 0).
- β₁ is the slope (the change in Y for a one-unit change in X).
- ε is the error term (representing the variability not explained by the model).
The slope (β₁) is the parameter of primary interest in many regression analyses. It quantifies the magnitude and direction of the linear relationship between X and Y. A positive slope indicates a positive relationship (as X increases, Y increases), while a negative slope indicates a negative relationship (as X increases, Y decreases).
Why is a Confidence Interval for the Slope Necessary?
The slope we calculate from our sample data (denoted as 'b₁') is only an estimate of the true population slope (β₁). Because we're working with a sample, there's inherent uncertainty surrounding this estimate. A confidence interval acknowledges this uncertainty, providing a range of plausible values for the true population slope.
Instead of simply stating a point estimate (b₁), a confidence interval provides a range within which we are confident the true population slope lies. This range is crucial for making informed conclusions about the relationship between X and Y. A wide confidence interval suggests substantial uncertainty, while a narrow interval indicates a more precise estimate.
Calculating the Confidence Interval for the Slope
The formula for calculating the confidence interval for the slope of a regression line is:
b₁ ± t(SEb₁)*
Where:
- b₁ is the estimated slope from the sample data.
- t is the critical t-value from the t-distribution, corresponding to the desired confidence level and degrees of freedom (df = n - 2, where n is the sample size).
- SEb₁ is the standard error of the slope.
Let's break down each component:
1. Estimated Slope (b₁)
This is obtained from your regression analysis. Most statistical software packages readily provide this value.
2. Critical t-value (t)
This value depends on:
- Confidence Level: The probability that the true population slope falls within the calculated interval (commonly 95% or 99%).
- Degrees of Freedom (df): This is equal to n - 2, where n is the number of data points in your sample.
You can find the critical t-value using a t-table or statistical software.
3. Standard Error of the Slope (SEb₁)
This measures the variability of the estimated slope. It's calculated as:
SEb₁ = s / √(Σ(xᵢ - x̄)²)
Where:
- s is the standard error of the regression (a measure of the variability of the data around the regression line).
- xᵢ represents individual values of the independent variable.
- x̄ represents the mean of the independent variable.
- Σ(xᵢ - x̄)² represents the sum of squared deviations of the independent variable from its mean. This is a measure of the variability in the independent variable. A larger variability in X leads to a smaller standard error of the slope, suggesting a more precise estimate.
The standard error of the regression (s) is often provided directly by statistical software. It can also be calculated as:
s = √[Σ(yᵢ - ŷᵢ)² / (n - 2)]
Where:
- yᵢ represents the observed values of the dependent variable.
- ŷᵢ represents the predicted values of the dependent variable from the regression equation.
Interpreting the Confidence Interval
Once you've calculated the confidence interval (lower bound, upper bound), you can interpret it as follows:
-
Confidence Level: We are [confidence level]% confident that the true population slope (β₁) lies within this interval. For example, a 95% confidence interval means we are 95% confident that the true slope is within the calculated range.
-
Range: The width of the interval reflects the precision of your estimate. A narrow interval indicates a more precise estimate, while a wide interval suggests greater uncertainty.
-
Zero in the Interval: If the confidence interval includes zero, it suggests that there is not enough evidence to conclude a statistically significant linear relationship between X and Y. The effect of X on Y could be zero. This does not necessarily mean there's no relationship at all; it simply means the relationship isn't strong enough to be detected with the given data and confidence level.
-
Sign of the Slope: The sign of the slope (positive or negative) within the confidence interval indicates the direction of the relationship. If the entire interval is positive, you can confidently conclude a positive relationship. Similarly, a negative interval suggests a negative relationship.
Assumptions of Linear Regression and Confidence Interval Validity
The validity of the confidence interval relies on several assumptions of linear regression:
- Linearity: The relationship between X and Y is linear.
- Independence: Observations are independent of each other.
- Homoscedasticity: The variance of the error term (ε) is constant across all levels of X.
- Normality: The error term (ε) is normally distributed.
Violations of these assumptions can affect the reliability of the confidence interval. Diagnostic plots and tests (e.g., residual plots, Breusch-Pagan test for homoscedasticity, Shapiro-Wilk test for normality) can help assess these assumptions. If assumptions are violated, transformations of the data or the use of alternative regression techniques might be necessary.
Practical Applications and Examples
Confidence intervals for the slope of a regression line are widely used across various fields, including:
- Economics: Analyzing the relationship between economic variables (e.g., inflation and unemployment).
- Medicine: Evaluating the effectiveness of a treatment by analyzing the relationship between treatment and outcome.
- Engineering: Assessing the strength of materials by examining the relationship between stress and strain.
- Environmental Science: Studying the relationship between pollution levels and environmental impacts.
- Social Sciences: Investigating the association between social factors and behavior.
Example: Suppose a researcher is investigating the relationship between hours studied (X) and exam scores (Y). After conducting a regression analysis, they obtain:
- b₁ = 0.8 (estimated slope)
- SEb₁ = 0.2 (standard error of the slope)
- n = 50 (sample size)
- 95% Confidence Level
For a 95% confidence level and df = 48 (50 - 2), the critical t-value is approximately 2.01.
The 95% confidence interval is:
0.8 ± 2.01 * 0.2 = (0.40, 1.20)
This indicates that the researcher is 95% confident that the true population slope lies between 0.40 and 1.20. Since the interval does not contain zero, there is statistically significant evidence of a positive linear relationship between hours studied and exam scores.
Conclusion
The confidence interval for the slope of a regression line is a powerful statistical tool providing a range of plausible values for the true population slope. Understanding its calculation, interpretation, and underlying assumptions is crucial for drawing accurate and reliable conclusions from your regression analysis. By carefully considering these aspects, researchers can confidently assess the strength and significance of the relationship between variables in their study. Remember to always check the assumptions of linear regression to ensure the validity of your results. Using appropriate statistical software can greatly simplify the calculation and interpretation of these intervals.
Latest Posts
Latest Posts
-
Current Source To Voltage Source Conversion
Apr 19, 2025
-
Indicate The Two Statements That Describe Chemical Bonds
Apr 19, 2025
-
Dispersion Forces Are Specifically The Interaction Between
Apr 19, 2025
-
Where Does The Citric Acid Cycle Occur In Eukaryotic Cells
Apr 19, 2025
-
Any Atoms That Have Gained Electrons Are Called
Apr 19, 2025
Related Post
Thank you for visiting our website which covers about Confidence Interval For Slope Of Regression Line Formula . We hope the information provided has been useful to you. Feel free to contact us if you have any questions or need further assistance. See you next time and don't miss to bookmark.