Convert Z Score To Raw Score
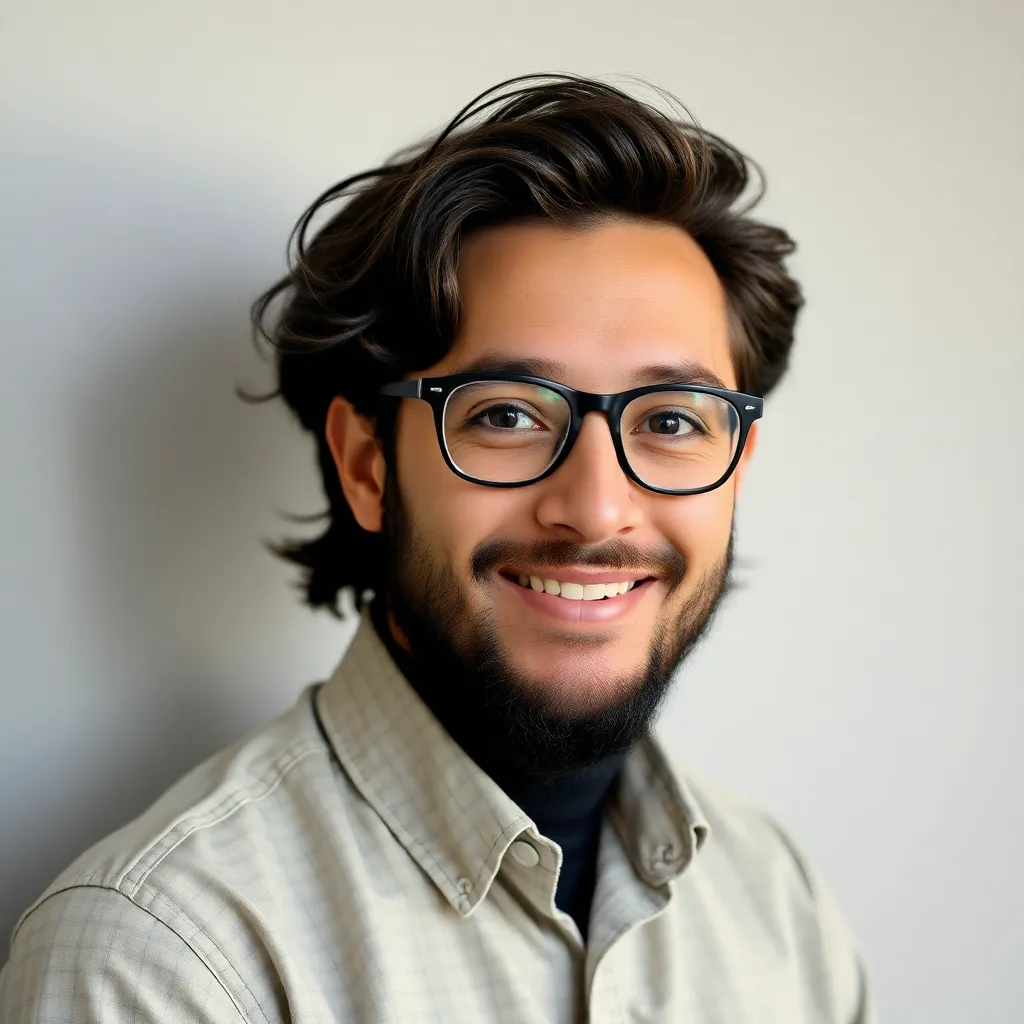
Muz Play
Apr 19, 2025 · 6 min read

Table of Contents
Converting Z-scores to Raw Scores: A Comprehensive Guide
Understanding and utilizing Z-scores is a fundamental skill in statistics. Z-scores, also known as standard scores, represent the number of standard deviations a data point is from the mean of its distribution. They allow us to compare data points from different distributions and assess the relative position of a specific value within a dataset. While Z-scores are incredibly useful for standardization and analysis, often we need to convert them back into their original, raw score units. This article provides a comprehensive guide on how to convert Z-scores to raw scores, covering the underlying concepts, the necessary formula, step-by-step examples, and various applications.
Understanding Z-Scores and Raw Scores
Before diving into the conversion process, let's solidify our understanding of Z-scores and raw scores.
-
Raw Score: This is the original, unstandardized value of a data point. It's the score you obtain directly from a measurement or observation, like a student's test score (e.g., 85 out of 100), a person's height (e.g., 175 cm), or a company's profit margin (e.g., 15%).
-
Z-Score: This is a standardized score that indicates how many standard deviations a raw score is above or below the mean of the distribution. A positive Z-score means the raw score is above the mean, a negative Z-score means it's below the mean, and a Z-score of 0 means the raw score is equal to the mean.
The Formula for Converting Z-scores to Raw Scores
The core formula for converting a Z-score back to a raw score is:
Raw Score = (Z-score * Standard Deviation) + Mean
Where:
- Z-score: The standardized score you want to convert.
- Standard Deviation: The standard deviation of the original dataset. This represents the spread or dispersion of the data.
- Mean: The average (mean) of the original dataset. This represents the center of the data distribution.
Step-by-Step Guide to Converting Z-scores to Raw Scores
Let's walk through a step-by-step example to illustrate the conversion process.
Example:
Suppose a student scored a Z-score of 1.5 on a math exam. The mean score on the exam was 75, and the standard deviation was 10. What was the student's raw score on the exam?
Steps:
-
Identify the Z-score: Z-score = 1.5
-
Identify the Mean: Mean = 75
-
Identify the Standard Deviation: Standard Deviation = 10
-
Apply the formula:
Raw Score = (Z-score * Standard Deviation) + Mean Raw Score = (1.5 * 10) + 75 Raw Score = 15 + 75 Raw Score = 90
Therefore, the student's raw score on the math exam was 90.
Practical Applications of Z-score to Raw Score Conversion
The ability to convert Z-scores back to raw scores is essential in various real-world scenarios. Here are some key applications:
1. Interpreting Standardized Test Scores
Many standardized tests, like the SAT or GRE, report scores as Z-scores or percentiles, which are derived from Z-scores. Converting these standardized scores to raw scores allows for a more intuitive understanding of the performance relative to the original test scale. For example, understanding the raw score on a college entrance exam helps in comparing scores with individual requirements of different universities.
2. Analyzing Research Data
In research studies, data is often standardized using Z-scores for analysis. However, when presenting the results, researchers often need to convert Z-scores back to raw scores to make the findings more accessible and understandable to the audience. This is particularly crucial when presenting results in a less technical format for a broader audience. For instance, in medical research, Z-scores might be used in statistical analysis, but converting to raw scores provides meaningful context regarding blood pressure, weight, or other health measures.
3. Quality Control and Process Improvement
In manufacturing and quality control, Z-scores are often used to monitor process variation. Converting Z-scores to raw scores allows for a direct assessment of how far a particular measurement deviates from the target value in the original units of measurement. For instance, Z-scores can measure deviation from the ideal weight of a product during the manufacturing process. Converting them back to grams or kilograms helps establish the scale of the quality control issue.
4. Financial Analysis
Financial markets frequently use Z-scores to analyze market movements and assess risk. Converting Z-scores back to raw scores – such as stock prices or portfolio values – helps investors comprehend the actual monetary implications of changes in market conditions. The actual financial impact of a market shift is easier to understand when Z-scores are translated to raw values.
5. Educational Assessment
In education, Z-scores can be used to compare student performance across different classes or schools. Converting Z-scores to raw scores helps educators understand the actual student performance in terms of the original grading scale (e.g., percentage scores), facilitating the design of more targeted interventions. This provides a more tangible picture of a student's understanding compared to simply interpreting a standardized score.
Handling Different Distributions
The formula for converting Z-scores to raw scores assumes a normal distribution. However, if the underlying data distribution is significantly non-normal, the conversion might not be entirely accurate. In such cases, more sophisticated statistical techniques might be required for accurate interpretation and conversion.
For instance, data that has significant skewness or outliers might require transformations before applying the Z-score to raw score conversion. Methods like logarithmic transformations or Winsorizing outliers can help mitigate the impact of the non-normality on the conversion process. The choice of method will depend on the nature of the data and the research question.
Importance of Accurate Mean and Standard Deviation
The accuracy of the raw score conversion heavily relies on the accuracy of the mean and standard deviation used in the calculation. Using inaccurate values for the mean and standard deviation will lead to inaccurate raw score conversions. Therefore, using reliable data collection methods and correct statistical techniques to calculate these values are essential for achieving accurate results.
Using Software for Z-score to Raw Score Conversion
Statistical software packages like R, SPSS, and Excel offer functionalities for performing Z-score to raw score conversions. These programs automate the calculation, reducing the likelihood of manual calculation errors and allowing for efficient processing of large datasets. These tools can handle large datasets, reducing the risk of human error and speeding up the analysis process.
Conclusion
Converting Z-scores to raw scores is a critical statistical procedure that allows for the meaningful interpretation of standardized data in its original context. By understanding the underlying formula, applying it correctly, and being aware of potential challenges related to non-normal distributions, researchers, analysts, and students can effectively transform standardized scores into their original units, facilitating deeper insights and informed decision-making across various fields. Remember that accurate values for the mean and standard deviation are essential for accurate conversion. Utilizing statistical software can significantly simplify and improve the efficiency of this process.
Latest Posts
Latest Posts
-
Transcription Begins Near A Site In The Dna Called The
Apr 19, 2025
-
Conventional Current Flows From Positive To Negative
Apr 19, 2025
-
Mass Media Is A Form Of Socialization
Apr 19, 2025
-
An Atom Or Molecule With A Net Electrical Charge
Apr 19, 2025
-
During Glycolysis Atp Is Produced By
Apr 19, 2025
Related Post
Thank you for visiting our website which covers about Convert Z Score To Raw Score . We hope the information provided has been useful to you. Feel free to contact us if you have any questions or need further assistance. See you next time and don't miss to bookmark.