Difference Between Chi Square Homogeneity And Independence
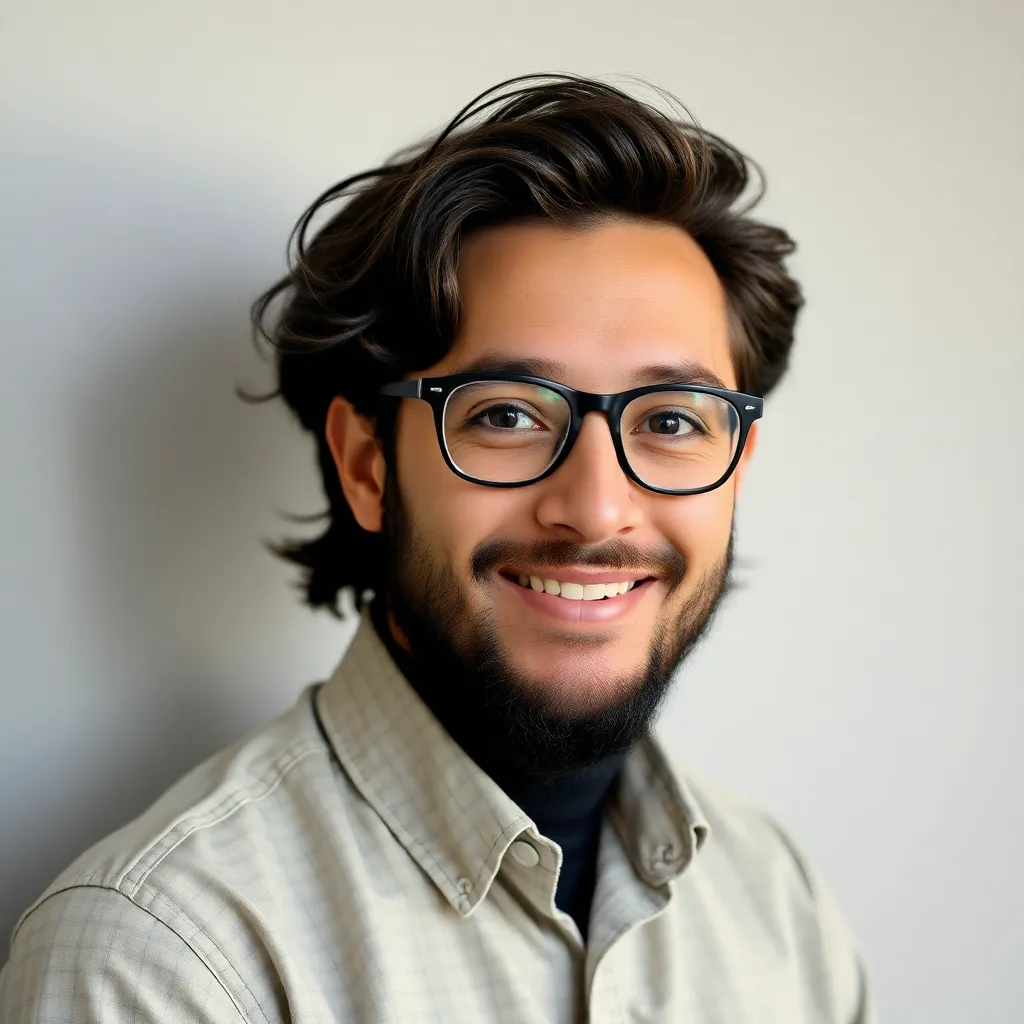
Muz Play
Apr 16, 2025 · 6 min read

Table of Contents
Chi-Square Test of Homogeneity vs. Independence: A Comprehensive Guide
The chi-square test is a powerful statistical tool used to analyze categorical data. However, within the chi-square family, two tests are frequently confused: the chi-square test of homogeneity and the chi-square test of independence. While both use the same underlying chi-square distribution and similar calculations, they address different research questions and involve distinct experimental designs. Understanding their differences is crucial for accurate data analysis and interpretation. This comprehensive guide will delve into the nuances of each test, clarifying their applications, assumptions, and interpretations.
Understanding Categorical Data and the Chi-Square Test
Before diving into the specifics of homogeneity and independence tests, let's briefly review categorical data and the chi-square test's fundamental principles. Categorical data represents qualities or characteristics rather than numerical values. Examples include gender (male/female), eye color (blue, brown, green), or political affiliation (Democrat, Republican, Independent). The chi-square test assesses the relationship between two or more categorical variables by comparing observed frequencies with expected frequencies under a specific hypothesis. A significant chi-square statistic suggests a statistically significant difference between observed and expected frequencies, indicating a relationship between the variables.
Chi-Square Test of Independence: Examining the Relationship Between Two Variables
The chi-square test of independence investigates whether two categorical variables are associated or independent. In essence, it asks: "Is there a relationship between these two variables?" The test analyzes data from a single sample where both variables are measured for each subject. The data are usually presented in a contingency table, showing the frequencies of each combination of categories for the two variables.
Example: Relationship between Smoking and Lung Cancer
Imagine a study investigating the relationship between smoking and lung cancer. Researchers collect data on a single sample of individuals, recording their smoking status (smoker/non-smoker) and whether they have lung cancer (yes/no). The data are organized into a 2x2 contingency table:
Lung Cancer (Yes) | Lung Cancer (No) | Total | |
---|---|---|---|
Smoker | a | b | a+b |
Non-Smoker | c | d | c+d |
Total | a+c | b+d | N |
The chi-square test of independence would determine if there's a significant association between smoking status and lung cancer. A significant result would suggest that smoking and lung cancer are not independent; that is, smoking status influences the likelihood of developing lung cancer.
Assumptions of the Chi-Square Test of Independence:
- Random Sampling: The sample data should be randomly selected from the population of interest.
- Independence of Observations: Each observation should be independent of the others.
- Expected Cell Frequencies: Expected cell frequencies (calculated based on the assumption of independence) should be sufficiently large. A common rule of thumb is that at least 80% of the cells should have an expected frequency of 5 or more, and no cell should have an expected frequency of less than 1.
Chi-Square Test of Homogeneity: Comparing the Distributions of a Single Variable Across Multiple Groups
The chi-square test of homogeneity examines whether the distribution of a single categorical variable is the same across two or more different populations or groups. It asks: "Are the distributions of this variable similar across these groups?" Unlike the independence test, the homogeneity test involves comparing multiple independent samples, each representing a different population or group.
Example: Comparing Smoking Rates Across Different Age Groups
Consider a study comparing smoking rates among three different age groups: 18-25, 26-40, and 41-55. Researchers collect independent samples from each age group and record the smoking status (smoker/non-smoker) for each individual. The data would be organized into a contingency table:
18-25 | 26-40 | 41-55 | Total | |
---|---|---|---|---|
Smoker | a | b | c | a+b+c |
Non-Smoker | d | e | f | d+e+f |
Total | a+d | b+e | c+f | N |
The chi-square test of homogeneity would determine if the distribution of smoking status is the same across the three age groups. A significant result would indicate that the proportion of smokers differs significantly among the age groups.
Assumptions of the Chi-Square Test of Homogeneity:
- Random Sampling: Each sample should be randomly selected from its respective population.
- Independence of Observations: Observations within each sample should be independent.
- Independence of Samples: The samples from different groups should be independent of each other.
- Expected Cell Frequencies: Similar to the independence test, expected cell frequencies should be sufficiently large.
Key Differences Summarized:
Feature | Chi-Square Test of Independence | Chi-Square Test of Homogeneity |
---|---|---|
Research Question | Is there an association between two variables? | Are the distributions of a variable the same across groups? |
Number of Samples | One sample with two variables measured | Multiple independent samples, one variable measured |
Data Structure | Contingency table (two variables) | Contingency table (one variable across multiple groups) |
Hypothesis | Null: Variables are independent; Alternative: Variables are associated | Null: Distributions are homogeneous; Alternative: Distributions are not homogeneous |
Choosing the Correct Test: A Practical Guide
Selecting the appropriate chi-square test hinges on the research question and the study design. Here's a practical guide to help you choose:
-
Identify your variables: Determine the number of categorical variables you have (two or more).
-
Determine the sampling method: Are you collecting data from one sample or multiple independent samples?
-
Formulate your research question: Are you interested in the relationship between two variables (independence) or the distribution of a variable across different groups (homogeneity)?
-
If you have two variables and one sample: Use the chi-square test of independence.
-
If you have one variable and multiple independent samples: Use the chi-square test of homogeneity.
Beyond the Basics: Interpreting Results and Effect Size
Both tests produce a chi-square statistic and a p-value. The p-value indicates the probability of observing the data (or more extreme data) if the null hypothesis (independence or homogeneity) is true. A p-value less than a predetermined significance level (typically 0.05) leads to the rejection of the null hypothesis. However, a significant p-value only indicates the presence of an association or difference; it doesn't quantify the strength of the relationship or the magnitude of the difference.
To understand the strength of the association or the size of the difference, consider calculating an effect size measure. For chi-square tests, Cramer's V is a common effect size measure. It ranges from 0 (no association) to 1 (perfect association).
Conclusion: Mastering Chi-Square Tests for Powerful Data Analysis
The chi-square test of independence and the chi-square test of homogeneity are valuable tools for analyzing categorical data. Understanding their subtle yet crucial differences is essential for accurate data analysis and meaningful interpretation. By carefully considering the research question, study design, and assumptions of each test, researchers can effectively use these methods to investigate relationships between categorical variables and compare the distributions of variables across different groups. Remember to always consider the effect size to gain a comprehensive understanding of your results. Through careful application and interpretation, you can harness the power of chi-square analysis to extract valuable insights from categorical data.
Latest Posts
Latest Posts
-
Which Of The Following Minerals Is A Ferromagnesian Silicate
Apr 16, 2025
-
How Can Density Be Used To Identify Substances
Apr 16, 2025
-
An Element In Period 2 And Group 5a
Apr 16, 2025
-
What Are The Two Shapes Found In Microscopic Fungi
Apr 16, 2025
-
Examples Of Wilcoxon Signed Rank Test
Apr 16, 2025
Related Post
Thank you for visiting our website which covers about Difference Between Chi Square Homogeneity And Independence . We hope the information provided has been useful to you. Feel free to contact us if you have any questions or need further assistance. See you next time and don't miss to bookmark.