Difference Between P Hat And P
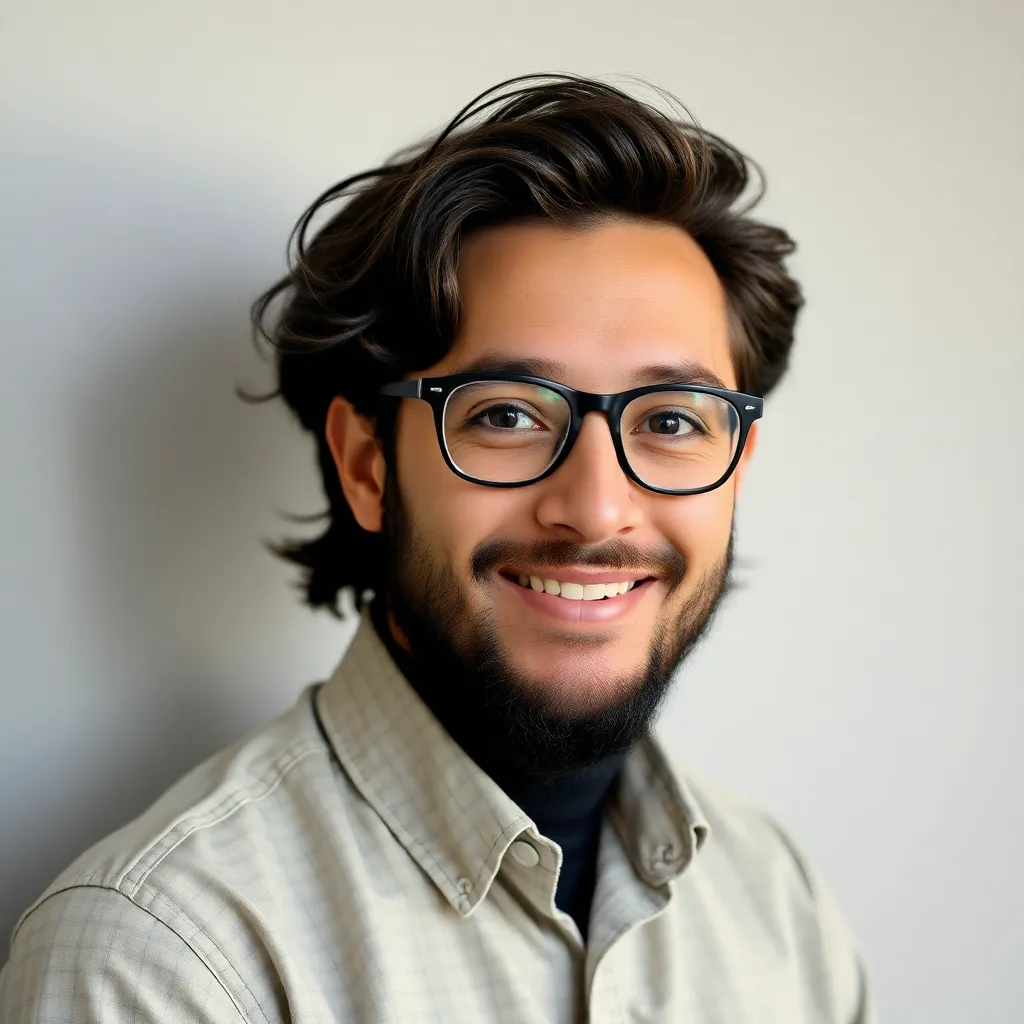
Muz Play
May 09, 2025 · 6 min read
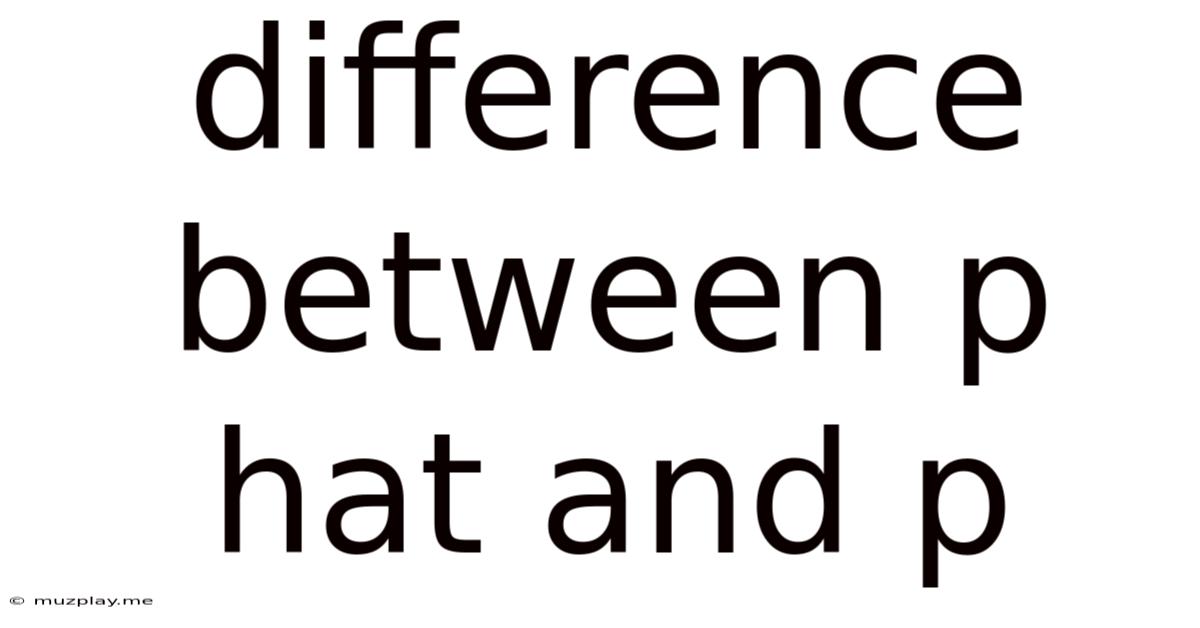
Table of Contents
Understanding the Difference Between p-hat (p̂) and p: A Deep Dive into Statistical Inference
In the realm of statistics, understanding the nuances of notation is crucial for accurate interpretation and analysis. Two symbols frequently encountered, especially in hypothesis testing and confidence intervals, are p and p̂ (p-hat). While both relate to proportions, their meanings and applications differ significantly. This article will delve deep into the distinction between these two, clarifying their roles and providing practical examples to solidify your understanding.
What is p (Population Proportion)?
p represents the population proportion. It's the true, but often unknown, proportion of individuals in a population possessing a specific characteristic or attribute. Think of it as the "big picture" – the actual percentage of the entire group you're interested in. For example:
- p could be the true proportion of voters who will support a particular candidate in an upcoming election.
- p could represent the actual percentage of defective items produced by a manufacturing process.
- p could be the true proportion of individuals in a city who have a specific disease.
Because it's usually impossible to survey every single member of a population (especially large ones!), p remains unknown and needs to be estimated. This is where p̂ comes into play.
The Challenges of Obtaining p
Several factors make determining the exact value of p practically impossible:
- Population Size: Many populations are extremely large, making a complete census extremely time-consuming and expensive.
- Accessibility: Not all members of a population are easily accessible or willing to participate in a study.
- Resources: Conducting a complete census often requires substantial resources (time, money, personnel).
- Dynamic Nature: Population characteristics can change over time. By the time a complete census is finished, the true proportion p might already be different.
What is p̂ (Sample Proportion)?
p̂ (p-hat) represents the sample proportion. It's the proportion of individuals in a sample who possess the characteristic of interest. A sample is a smaller, manageable subset of the population selected for study. p̂ is calculated from the sample data and serves as an estimate of the true population proportion, p.
For example:
- If we survey 1000 voters (our sample) and 600 say they will vote for a particular candidate, then p̂ = 600/1000 = 0.6 (or 60%).
- If we inspect 500 items from a production line and find 10 defective items, then p̂ = 10/500 = 0.02 (or 2%).
The Importance of Random Sampling
The accuracy of p̂ as an estimator of p heavily relies on the method used to select the sample. Random sampling is crucial. A random sample ensures that every member of the population has an equal chance of being selected, minimizing bias and improving the reliability of the estimate. Biased sampling methods can lead to p̂ being a poor representation of p, resulting in inaccurate conclusions.
Properties of p̂ as an Estimator
p̂ possesses several important properties as an estimator of p:
- Unbiasedness: On average, p̂ will equal p. Over many repeated samples, the average of all sample proportions will converge to the true population proportion.
- Consistency: As the sample size increases, p̂ will become increasingly closer to p. Larger samples generally yield more precise estimates.
- Efficiency: Among unbiased estimators, p̂ is relatively efficient, meaning it has a smaller variance than many other estimators of p.
The Relationship Between p and p̂
The core relationship between p and p̂ is that p̂ is an estimate of p. We use the sample proportion, p̂, to make inferences about the unknown population proportion, p. This is the foundation of statistical inference, which allows us to draw conclusions about a population based on a sample.
Confidence Intervals: Bridging p and p̂
Confidence intervals are a powerful tool that quantifies the uncertainty associated with estimating p using p̂. A 95% confidence interval, for instance, provides a range of values within which we are 95% confident that the true population proportion, p, lies. The confidence interval is centered around p̂ and its width depends on the sample size and the desired level of confidence. A larger sample size generally leads to a narrower confidence interval, reflecting greater precision in the estimation.
Hypothesis Testing: Using p̂ to Test Claims About p
Hypothesis testing allows us to test claims or hypotheses about the population proportion, p. We use the sample proportion, p̂, as evidence to either reject or fail to reject the null hypothesis. For example, we might test the hypothesis that the population proportion of voters supporting a candidate is equal to 50% (H₀: p = 0.5). We would calculate the sample proportion, p̂, from a survey, and use statistical tests (like a z-test) to determine whether the observed p̂ provides sufficient evidence to reject the null hypothesis.
Illustrative Examples
Let's solidify our understanding with a few concrete examples:
Example 1: Quality Control
A manufacturing company produces light bulbs. They want to estimate the proportion of defective bulbs (p). They randomly sample 1000 bulbs and find 20 defective bulbs.
- p̂ (sample proportion): 20/1000 = 0.02 (or 2%)
- p (population proportion): Unknown. p̂ = 0.02 serves as an estimate of p.
Example 2: Election Polling
A polling company wants to estimate the proportion of voters who support Candidate A (p). They randomly survey 500 registered voters and find that 300 support Candidate A.
- p̂ (sample proportion): 300/500 = 0.6 (or 60%)
- p (population proportion): Unknown. p̂ = 0.6 serves as an estimate of p.
Example 3: Medical Research
Researchers want to determine the proportion of individuals in a population with a particular disease (p). They collect data from a random sample of 200 individuals and find that 30 have the disease.
- p̂ (sample proportion): 30/200 = 0.15 (or 15%)
- p (population proportion): Unknown. p̂ = 0.15 serves as an estimate of p.
In all these scenarios, p̂ provides a valuable estimate of the unknown p, but it's crucial to remember that it is only an estimate. The accuracy of this estimate depends on the sample size and the sampling method.
Conclusion: p and p̂ – Two Sides of the Same Coin
In conclusion, while both p and p̂ relate to proportions, their roles are distinct. p represents the true, but often unknown, population proportion, while p̂ is the sample proportion, an estimate of p calculated from a sample. Understanding this fundamental difference is vital for accurate interpretation of statistical analyses, particularly in hypothesis testing and confidence interval construction. Remember that p̂ provides valuable insight into p, but always acknowledge the inherent uncertainty and limitations associated with using sample data to infer population characteristics. Appropriate statistical methods and careful consideration of sampling techniques are essential for drawing meaningful conclusions.
Latest Posts
Latest Posts
-
How To Find The Focal Length Of A Parabola
May 11, 2025
-
Symptoms Of Ptsd Include All Of The Following Except
May 11, 2025
-
Use Symmetry To Graph The Inverse Of The Function
May 11, 2025
-
Describe How You Would Prepare A Supersaturated Solution
May 11, 2025
-
Cross Section Of Monocot And Dicot Stem
May 11, 2025
Related Post
Thank you for visiting our website which covers about Difference Between P Hat And P . We hope the information provided has been useful to you. Feel free to contact us if you have any questions or need further assistance. See you next time and don't miss to bookmark.