Difference Between Relative And Cumulative Frequency
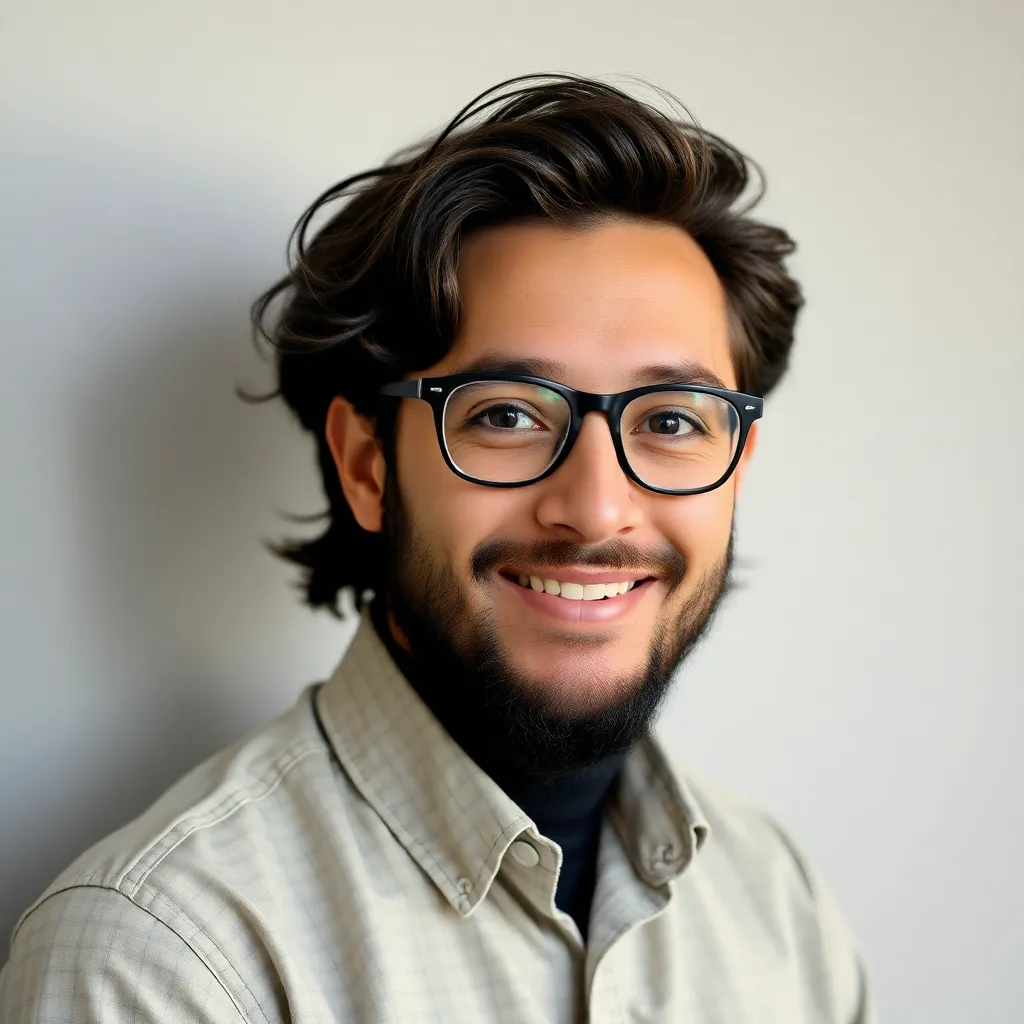
Muz Play
May 10, 2025 · 6 min read
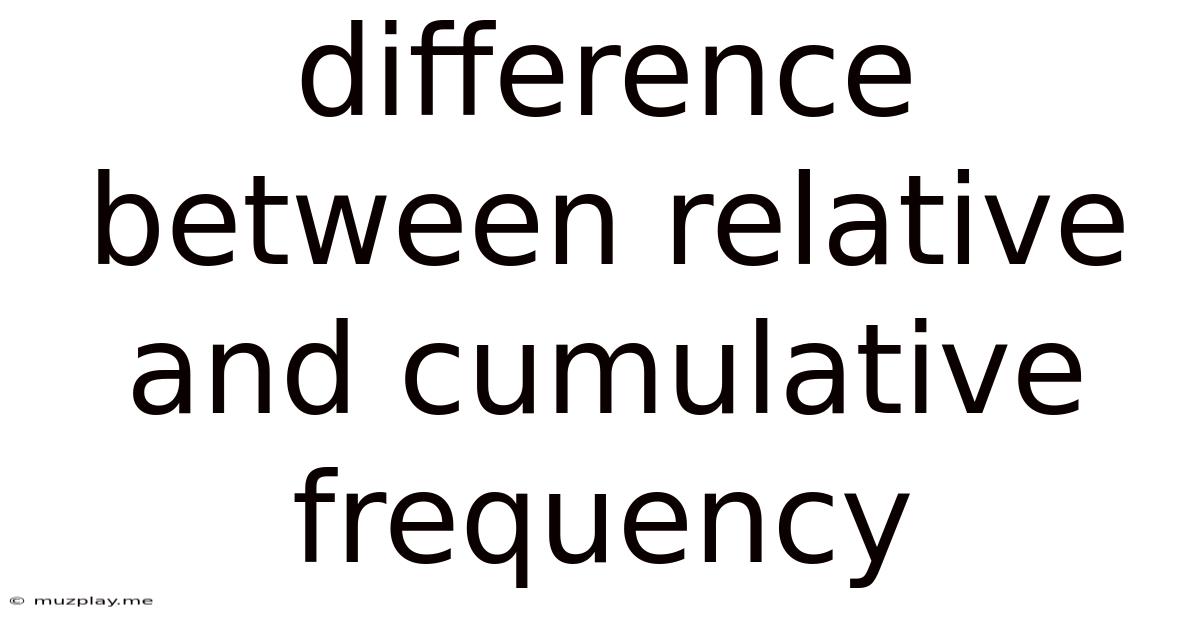
Table of Contents
Understanding the Difference Between Relative and Cumulative Frequency
Understanding frequency distributions is crucial for analyzing data effectively. While simple frequency counts tell us how often each data point appears, relative and cumulative frequencies provide deeper insights into the data's distribution. This article will delve into the distinct meanings, calculations, and applications of relative and cumulative frequencies, highlighting their differences and showcasing their usefulness in various contexts.
What is Frequency?
Before diving into relative and cumulative frequencies, let's establish a clear understanding of the basic concept of frequency. In statistics, frequency refers to the number of times a particular value or observation occurs in a dataset. For instance, if we're analyzing the heights of students in a class, the frequency of a specific height (e.g., 170 cm) represents the number of students who have that exact height. A frequency distribution table neatly organizes this information, listing each value and its corresponding frequency.
Relative Frequency: Proportion within the Whole
Relative frequency represents the proportion or percentage of times a specific value appears within the entire dataset. It's essentially the frequency of a value divided by the total number of observations. This normalization allows for easy comparison across datasets of different sizes. Instead of just knowing the raw count of occurrences, relative frequency expresses each value's contribution to the overall dataset.
Calculating Relative Frequency
The formula for calculating relative frequency is straightforward:
Relative Frequency = (Frequency of a Value) / (Total Number of Observations)
For example, if 5 students out of a class of 25 have a height of 170 cm, the relative frequency of that height is 5/25 = 0.2 or 20%. This tells us that 20% of the students in the class have a height of 170 cm.
Applications of Relative Frequency
Relative frequency is exceptionally useful in several scenarios:
-
Comparing datasets: When dealing with datasets of different sizes, relative frequency allows for meaningful comparisons. For example, comparing the percentage of students who scored above 90% on a test in two classes of different sizes becomes much easier using relative frequencies.
-
Visualizations: Relative frequencies are commonly used in various visualizations, such as pie charts, bar charts, and histograms, making it easy to grasp the proportions of different categories within a dataset.
-
Probability estimations: In situations where the dataset is considered representative of a larger population, relative frequency can serve as an estimate of the probability of observing a particular value.
-
Understanding data distribution: Relative frequency distributions provide a normalized view of how data is spread across different values, offering a clear picture of the overall distribution's shape.
Cumulative Frequency: The Running Total
Cumulative frequency represents the accumulated or running total of frequencies up to a certain point in the data. It tracks the total number of observations less than or equal to a given value. This gives us a sense of the proportion of data that falls below a certain threshold. Unlike relative frequency which focuses on individual values, cumulative frequency emphasizes the accumulation of frequencies.
Calculating Cumulative Frequency
Calculating cumulative frequency involves sequentially adding up the frequencies of values in ascending order (or descending, depending on your chosen arrangement). The cumulative frequency of a particular value is the sum of its frequency and the frequencies of all preceding values.
For instance, consider the following frequency distribution for student heights:
Height (cm) | Frequency | Cumulative Frequency |
---|---|---|
160 | 3 | 3 |
165 | 5 | 8 (3+5) |
170 | 7 | 15 (8+7) |
175 | 5 | 20 (15+5) |
180 | 2 | 22 (20+2) |
The cumulative frequency column shows the total number of students with a height less than or equal to the height in the corresponding row. For example, 15 students have heights of 170 cm or less.
Applications of Cumulative Frequency
Cumulative frequency is invaluable in various applications:
-
Percentile calculations: Cumulative frequency is fundamental to calculating percentiles, which describe the value below which a certain percentage of data falls. For example, the median (50th percentile) can be easily determined from the cumulative frequency distribution.
-
Identifying data thresholds: Cumulative frequency helps identify thresholds or critical points in the data. For example, in sales data, you might use cumulative frequency to find the point at which 80% of sales have been achieved.
-
Analyzing data trends: By observing how rapidly or slowly the cumulative frequency increases, insights can be gained into the overall pattern and trends within the data.
-
Creating cumulative frequency curves (ogives): Ogives are graphical representations of cumulative frequency distributions, enabling a visual understanding of data accumulation. This visualization is beneficial for quick interpretation of data distribution and percentile estimations.
Key Differences Between Relative and Cumulative Frequency
Feature | Relative Frequency | Cumulative Frequency |
---|---|---|
Focus | Proportion of individual values in the dataset | Accumulated frequency up to a given value |
Calculation | Frequency / Total Number of Observations | Sum of frequencies up to a given value |
Interpretation | Percentage or proportion of a specific value | Total number of observations less than or equal to a value |
Applications | Comparisons, visualizations, probability estimations | Percentile calculations, threshold identification, ogives |
Normalization | Yes (expressed as a proportion or percentage) | No (expressed as raw counts) |
Illustrative Example: Analyzing Exam Scores
Let's consider an example to solidify the differences. Imagine we have the following exam scores for a class of 20 students:
70, 80, 85, 90, 75, 80, 95, 100, 85, 80, 75, 90, 85, 70, 90, 85, 75, 80, 95, 90
First, let's create a frequency distribution table:
Score | Frequency | Relative Frequency | Cumulative Frequency |
---|---|---|---|
70 | 2 | 2/20 = 0.1 (10%) | 2 |
75 | 3 | 3/20 = 0.15 (15%) | 5 |
80 | 4 | 4/20 = 0.2 (20%) | 9 |
85 | 4 | 4/20 = 0.2 (20%) | 13 |
90 | 4 | 4/20 = 0.2 (20%) | 17 |
95 | 2 | 2/20 = 0.1 (10%) | 19 |
100 | 1 | 1/20 = 0.05 (5%) | 20 |
Relative frequency analysis: The table shows that the score of 80 and 85 each represents 20% of the scores, highlighting their prevalence.
Cumulative frequency analysis: We can see that 9 students scored 80 or less, and 17 students scored 90 or less. This helps determine percentiles: for example, a score of 85 represents the approximate 65th percentile (13/20).
This example clearly illustrates how relative and cumulative frequencies offer complementary perspectives on the same dataset, revealing different but equally valuable insights.
Conclusion
Relative and cumulative frequencies are powerful tools for data analysis. While relative frequency focuses on the proportion of individual values, cumulative frequency emphasizes the accumulation of frequencies up to a specific point. Understanding their distinct meanings, calculations, and applications allows for a comprehensive interpretation of data distributions and supports informed decision-making across various fields. By utilizing both approaches, we can gain a richer understanding of data patterns, trends, and distributions, leading to more effective data analysis and interpretation.
Latest Posts
Latest Posts
-
What Type Of Chlorophyll Does The Reaction Center Contain
May 10, 2025
-
Primary Source Of Energy For Cells
May 10, 2025
-
Formula For Cumulative Frequency In Excel
May 10, 2025
-
Animals Can Move As A Result Of What Energy Conversion
May 10, 2025
-
A Polar Covalent Bond Can Best Be Described As
May 10, 2025
Related Post
Thank you for visiting our website which covers about Difference Between Relative And Cumulative Frequency . We hope the information provided has been useful to you. Feel free to contact us if you have any questions or need further assistance. See you next time and don't miss to bookmark.