Does The Mean Represent The Center Of The Data
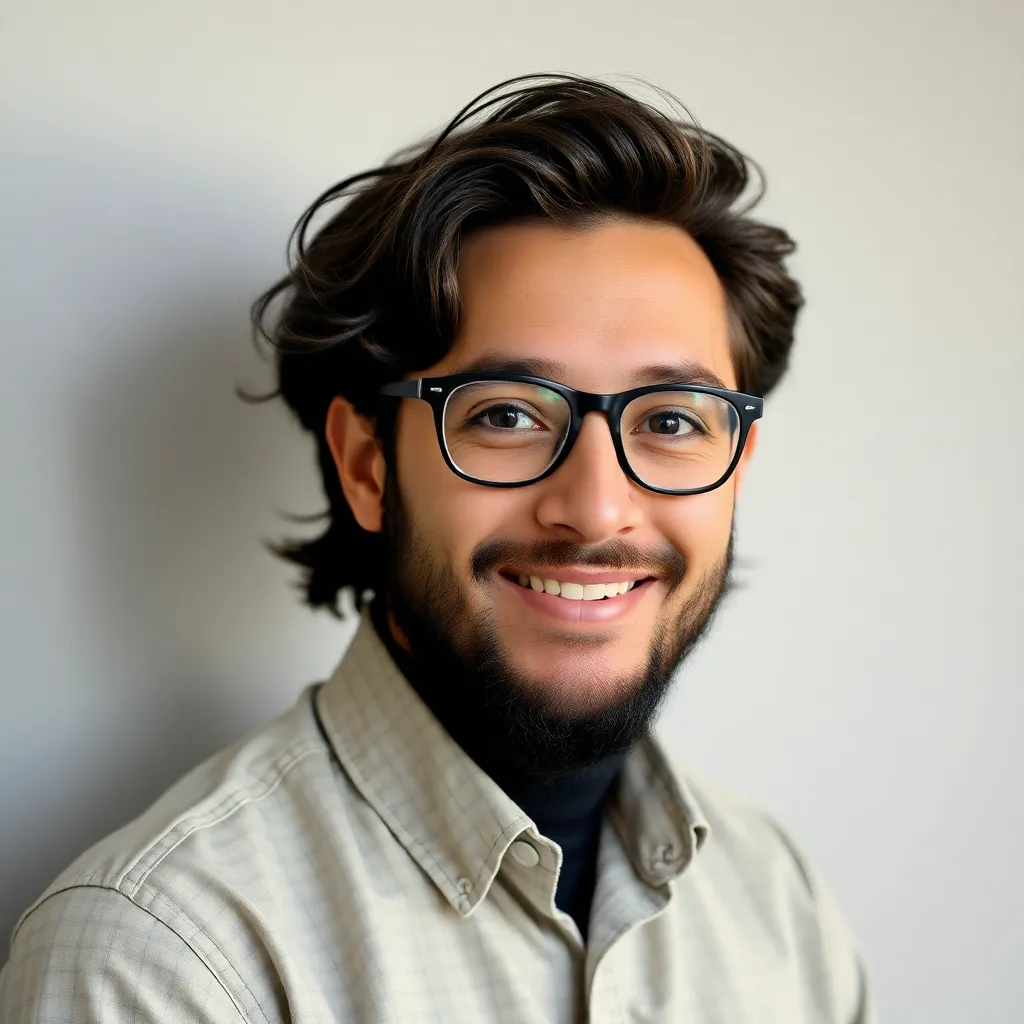
Muz Play
Apr 06, 2025 · 6 min read
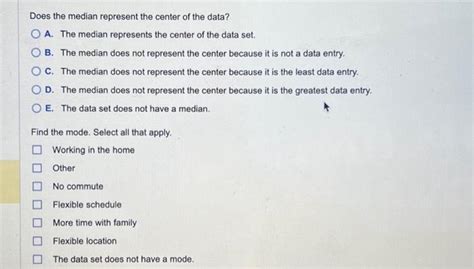
Table of Contents
Does the Mean Represent the Center of the Data? A Deep Dive into Central Tendency
The mean, often casually referred to as the "average," is a fundamental concept in statistics. It's a measure of central tendency, aiming to represent the typical or central value within a dataset. However, the question of whether the mean truly represents the center of the data is far more nuanced than a simple yes or no. While it's a widely used and often useful measure, the mean's effectiveness hinges critically on the nature of the data itself. This article will delve into the intricacies of the mean, exploring its strengths and limitations in accurately reflecting the center of a dataset. We'll examine scenarios where the mean is a reliable indicator and situations where it can be misleading, highlighting the importance of understanding the context and choosing the most appropriate measure of central tendency.
Understanding the Mean: Calculation and Interpretation
The mean is calculated by summing all the values in a dataset and then dividing by the number of values. This simple formula, while straightforward, masks the complexities involved in interpreting its meaning.
Formula:
Mean (x̄) = Σx / n
Where:
- Σx represents the sum of all values in the dataset.
- n represents the number of values in the dataset.
For example, consider the dataset: {2, 4, 6, 8, 10}. The mean is (2 + 4 + 6 + 8 + 10) / 5 = 6. In this simple case, the mean of 6 appears to accurately represent the center of the data. However, this is not always the case.
When the Mean Accurately Represents the Center: Symmetrical Distributions
The mean functions best as a measure of central tendency when the data follows a symmetrical distribution. A symmetrical distribution is one where the data is evenly balanced around the center. Think of a bell curve (normal distribution) – a classic example of a symmetrical distribution. In a perfectly symmetrical distribution, the mean, median, and mode are all equal and located at the exact center.
Examples of Symmetrical Distributions:
- Height of adult women: The distribution of heights for adult women generally approximates a normal distribution, exhibiting symmetry.
- Test scores in a large class: If the test is well-designed, the scores might cluster around the average, resulting in a relatively symmetrical distribution.
- Measurements from a well-calibrated instrument: Repeated measurements of a constant physical quantity should ideally be symmetrically distributed around the true value.
In such symmetrical scenarios, the mean provides a reliable and accurate representation of the central tendency. It effectively summarizes the typical value within the dataset.
When the Mean Fails: The Influence of Outliers
The significant weakness of the mean lies in its susceptibility to outliers. Outliers are extreme values that lie far outside the typical range of the data. Even a single extreme outlier can drastically skew the mean, pulling it away from the true center of the majority of the data.
Example:
Consider the dataset: {2, 4, 6, 8, 10, 100}. The mean is (2 + 4 + 6 + 8 + 10 + 100) / 6 = 21.67. Notice how the outlier, 100, significantly inflates the mean. The majority of the data points cluster around the lower values, yet the mean gives the impression of a much higher typical value. In this case, the mean poorly represents the center of the data. The median, in this case 7, provides a more accurate representation of the central tendency.
Real-World Examples of Outlier Influence:
- Income data: A few individuals with extremely high incomes can significantly inflate the mean income, masking the lower incomes experienced by the majority of the population.
- House prices: A few extremely expensive houses in a neighborhood can skew the average house price, making it appear higher than the typical value for most houses.
- Stock prices: A few volatile days with exceptionally high or low prices can skew the average daily stock price.
Skewed Distributions and the Mean's Limitations
Skewed distributions are characterized by an asymmetry in the data. In a positively skewed distribution, the tail stretches out to the right (higher values), while in a negatively skewed distribution, the tail stretches out to the left (lower values). The mean is particularly sensitive to skewness.
Positively Skewed Distributions: The mean will be pulled towards the right tail, resulting in a value higher than the median and mode.
Negatively Skewed Distributions: The mean will be pulled towards the left tail, resulting in a value lower than the median and mode.
Examples of Skewed Distributions:
- House prices (again): A neighborhood with a few very expensive homes will exhibit a positively skewed distribution.
- Insurance claims: Most claims will be relatively small, but a few large claims can create a positively skewed distribution.
- Survival times: In a study of survival times after a diagnosis of a serious illness, the data may be positively skewed due to some individuals surviving for a much longer time than others.
In skewed distributions, the mean often provides a misleading representation of the center because it's disproportionately influenced by the extreme values in the tail. The median, which represents the middle value when the data is ordered, often proves to be a more robust and accurate measure of central tendency in such scenarios.
Robustness: A Comparison of Mean, Median, and Mode
The term "robustness" in statistics refers to a statistic's resistance to outliers and deviations from assumptions. The mean is not robust, while the median is considerably more robust. The mode, representing the most frequent value, can also be useful, but it's less informative in continuous data.
- Mean: Highly sensitive to outliers and skewness.
- Median: Relatively resistant to outliers and skewness.
- Mode: Can be useful for categorical data or identifying the most frequent value, but less informative about the center for continuous data.
The choice of which measure to use depends on the specific dataset and the research question. If the data is symmetrical and free from outliers, the mean is a good choice. However, if the data is skewed or contains outliers, the median often provides a more accurate and robust representation of the center.
Beyond the Mean: Other Measures of Central Tendency
While the mean is widely used, it's crucial to remember that it's just one of several measures of central tendency. The choice of the appropriate measure should always be informed by the characteristics of the data.
- Median: The middle value when data is ordered. Less sensitive to outliers than the mean.
- Mode: The most frequent value. Useful for categorical data and identifying the most common outcome.
- Trimmed Mean: A modified mean that discards a certain percentage of the highest and lowest values, thus reducing the influence of outliers.
- Winsorized Mean: Similar to the trimmed mean, but instead of discarding extreme values, it replaces them with less extreme values (typically the highest and lowest remaining values).
Conclusion: Context Matters Most
In conclusion, the mean doesn't always represent the center of the data. Its effectiveness depends heavily on the distribution of the data. For symmetrical distributions without outliers, the mean is a reliable indicator of the center. However, for skewed distributions or datasets containing outliers, the mean can be misleading, and more robust measures like the median or trimmed mean may be more appropriate. The choice of the best measure of central tendency is not a one-size-fits-all solution. Understanding the nature of your data and the specific research question is paramount in selecting the most accurate and meaningful representation of the center. Always consider the context before relying solely on the mean as a definitive indicator of central tendency. A deeper understanding of data distribution and statistical properties will lead to a more accurate and insightful interpretation of your data.
Latest Posts
Latest Posts
-
What Is The Cortex In Plants
Apr 08, 2025
-
Method Of Joints And Method Of Sections
Apr 08, 2025
-
How Is Artificial Selection Different From Genetic Engineering
Apr 08, 2025
-
Which Chamber Initially Receives Blood From The Systemic Circuit
Apr 08, 2025
-
What Does The Term Nonrepresentational Art Mean
Apr 08, 2025
Related Post
Thank you for visiting our website which covers about Does The Mean Represent The Center Of The Data . We hope the information provided has been useful to you. Feel free to contact us if you have any questions or need further assistance. See you next time and don't miss to bookmark.