Example Of A True Experimental Design
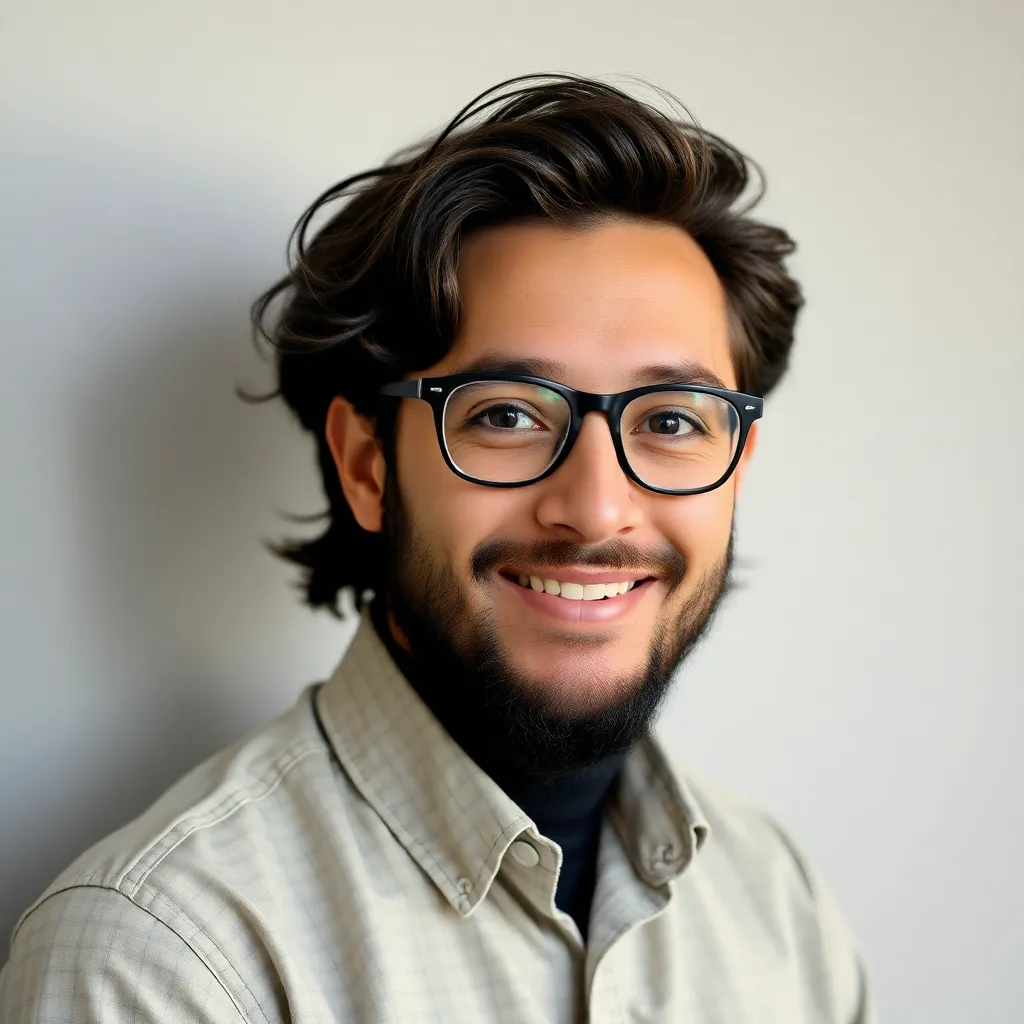
Muz Play
Apr 14, 2025 · 7 min read

Table of Contents
Examples of True Experimental Designs: A Comprehensive Guide
True experimental designs are the gold standard in research methodology, offering the strongest evidence for cause-and-effect relationships. Unlike observational studies, which merely document correlations, true experiments actively manipulate an independent variable to observe its impact on a dependent variable while controlling for extraneous factors. This rigorous approach minimizes confounding variables, allowing researchers to draw more confident conclusions about causality. This article will explore various examples of true experimental designs, highlighting their strengths, weaknesses, and applications.
Understanding the Core Components of a True Experiment
Before diving into specific examples, let's solidify our understanding of the essential elements that define a true experimental design:
- Independent Variable (IV): This is the variable that the researcher manipulates or controls. It's the presumed cause in the cause-and-effect relationship.
- Dependent Variable (DV): This is the variable that is measured or observed. It's the presumed effect resulting from the manipulation of the IV.
- Random Assignment: This crucial step involves randomly assigning participants to different groups (e.g., experimental and control groups). This helps ensure that the groups are comparable at the start of the experiment, minimizing pre-existing differences that could confound the results.
- Control Group: A group that doesn't receive the experimental treatment or intervention. This serves as a baseline for comparison, allowing researchers to isolate the effects of the independent variable.
- Pre-test and Post-test: Measurements taken before and after the manipulation of the independent variable. These provide data to assess the change in the dependent variable.
Classic Examples of True Experimental Designs
Several well-established designs fall under the umbrella of true experimental designs. Let's explore some key examples:
1. Pretest-Posttest Control Group Design
This is perhaps the most common true experimental design. Participants are randomly assigned to either an experimental group or a control group. Both groups are pre-tested on the dependent variable before the experimental manipulation. The experimental group receives the treatment, while the control group does not. Finally, both groups are post-tested on the dependent variable.
Example: A researcher wants to investigate the effectiveness of a new teaching method on student performance in mathematics.
- IV: New teaching method (present or absent)
- DV: Student scores on a mathematics test
- Groups: Experimental group (receives the new teaching method), Control group (receives traditional teaching)
- Procedure: Both groups take a pre-test, then the experimental group receives the new method, followed by a post-test for both groups. The difference in post-test scores between the two groups indicates the effect of the new teaching method.
Strengths: Provides strong evidence of causality due to random assignment and the inclusion of a control group. Pre-test data allows researchers to assess changes over time and ensure groups were comparable at the outset.
Weaknesses: Pre-testing can sensitize participants to the experimental manipulation, potentially affecting their responses on the post-test (testing effect).
2. Posttest-Only Control Group Design
This design omits the pre-test. Participants are randomly assigned to either the experimental or control group, the experimental group receives the treatment, and both groups are post-tested.
Example: A researcher wants to test the effectiveness of a new drug on blood pressure.
- IV: New drug (administered or not)
- DV: Blood pressure
- Groups: Experimental group (receives the new drug), Control group (receives a placebo)
- Procedure: Both groups receive their respective treatments, and then blood pressure is measured.
Strengths: Avoids the testing effect associated with pre-testing. Simpler and less time-consuming than the pretest-posttest design.
Weaknesses: Cannot assess changes in the dependent variable over time, making it more difficult to rule out pre-existing differences between groups.
3. Solomon Four-Group Design
This design combines the pretest-posttest and posttest-only control group designs. It involves four groups: two receive the treatment (one with a pre-test, one without), and two serve as control groups (one with a pre-test, one without).
Example: Investigating the effect of a new advertising campaign on consumer buying behavior.
- IV: Exposure to the new advertising campaign
- DV: Purchase of the advertised product
- Groups: Four groups - two exposed to the campaign (one with pre-campaign purchase data, one without), two control groups (one with pre-campaign data, one without).
- Procedure: Data is collected on purchasing behavior before and after the campaign, comparing the differences across all four groups.
Strengths: Allows researchers to assess the impact of pre-testing by comparing the results of the two control groups and the two experimental groups. Provides the most robust evidence for causality.
Weaknesses: Requires a larger sample size and more resources than other designs.
4. Factorial Designs
Factorial designs involve manipulating two or more independent variables simultaneously to examine their individual and combined effects on the dependent variable. They allow researchers to investigate interactions between variables – how the effect of one variable changes depending on the level of another.
Example: A study investigating the effects of different teaching styles (lecture vs. active learning) and levels of student motivation (high vs. low) on academic performance.
- IVs: Teaching style, student motivation (both with two levels each)
- DV: Academic performance
- Procedure: Students are randomly assigned to four groups representing all combinations of teaching style and motivation levels. Academic performance is measured after the intervention.
Strengths: Allows for investigation of interactions between variables and a more comprehensive understanding of the phenomenon.
Weaknesses: Can become complex to analyze with increasing numbers of independent variables and levels. Requires a larger sample size.
Choosing the Right Design
The selection of an appropriate true experimental design depends on several factors:
- Research question: The nature of the research question dictates the necessary design elements.
- Resources: The availability of time, participants, and funding influences the feasibility of different designs.
- Ethical considerations: The chosen design should minimize risks to participants and ensure their well-being.
- Practical limitations: Constraints like participant availability or the difficulty of manipulating the independent variable might restrict design choices.
Threats to Internal and External Validity in True Experiments
Even with rigorous designs, true experiments are susceptible to threats to validity:
Internal Validity: Refers to the confidence that the independent variable caused the observed changes in the dependent variable. Threats include:
- History: External events occurring during the experiment that could affect the results.
- Maturation: Natural changes in participants over time that could influence the dependent variable.
- Testing: The pre-test affecting performance on the post-test.
- Instrumentation: Changes in the measurement instruments or procedures during the experiment.
- Regression to the mean: Extreme scores on a pre-test tending to become less extreme on a post-test.
- Selection bias: Non-random assignment of participants to groups.
- Mortality: Participants dropping out of the study.
External Validity: Refers to the generalizability of the findings to other populations and settings. Threats include:
- Sample characteristics: The sample may not be representative of the broader population.
- Setting: The experimental setting may not be generalizable to real-world situations.
- Time: The findings may not hold true over time.
Careful planning, rigorous methodology, and appropriate statistical analysis are crucial for minimizing these threats and strengthening the internal and external validity of true experimental studies.
Conclusion
True experimental designs provide the strongest evidence for causality, making them invaluable for understanding cause-and-effect relationships. By carefully considering the research question, selecting the appropriate design, and addressing potential threats to validity, researchers can produce high-quality, impactful research that contributes meaningfully to our understanding of the world. The examples discussed above showcase the versatility and power of true experimental designs across various fields of study. The choice of a specific design will always depend on the specific research question and available resources, but the underlying principle of manipulating an independent variable to observe its effect on a dependent variable while controlling for extraneous factors remains consistent. This commitment to methodological rigor ensures the validity and reliability of the findings, contributing to a robust body of scientific knowledge.
Latest Posts
Latest Posts
-
Hormones Are Derived From All Of The Following Macromolecules Except
May 09, 2025
-
The Main Purpose Of Cellular Respiration Is To
May 09, 2025
-
How Do The Terms Enzyme Substrate And Active Site Relate
May 09, 2025
-
Elements That Are Poor Conductors Of Heat And Electricity
May 09, 2025
-
Which Of The Following Bacterial Structures Is Necessary For Chemotaxis
May 09, 2025
Related Post
Thank you for visiting our website which covers about Example Of A True Experimental Design . We hope the information provided has been useful to you. Feel free to contact us if you have any questions or need further assistance. See you next time and don't miss to bookmark.