Example Of Dependent Samples T Test
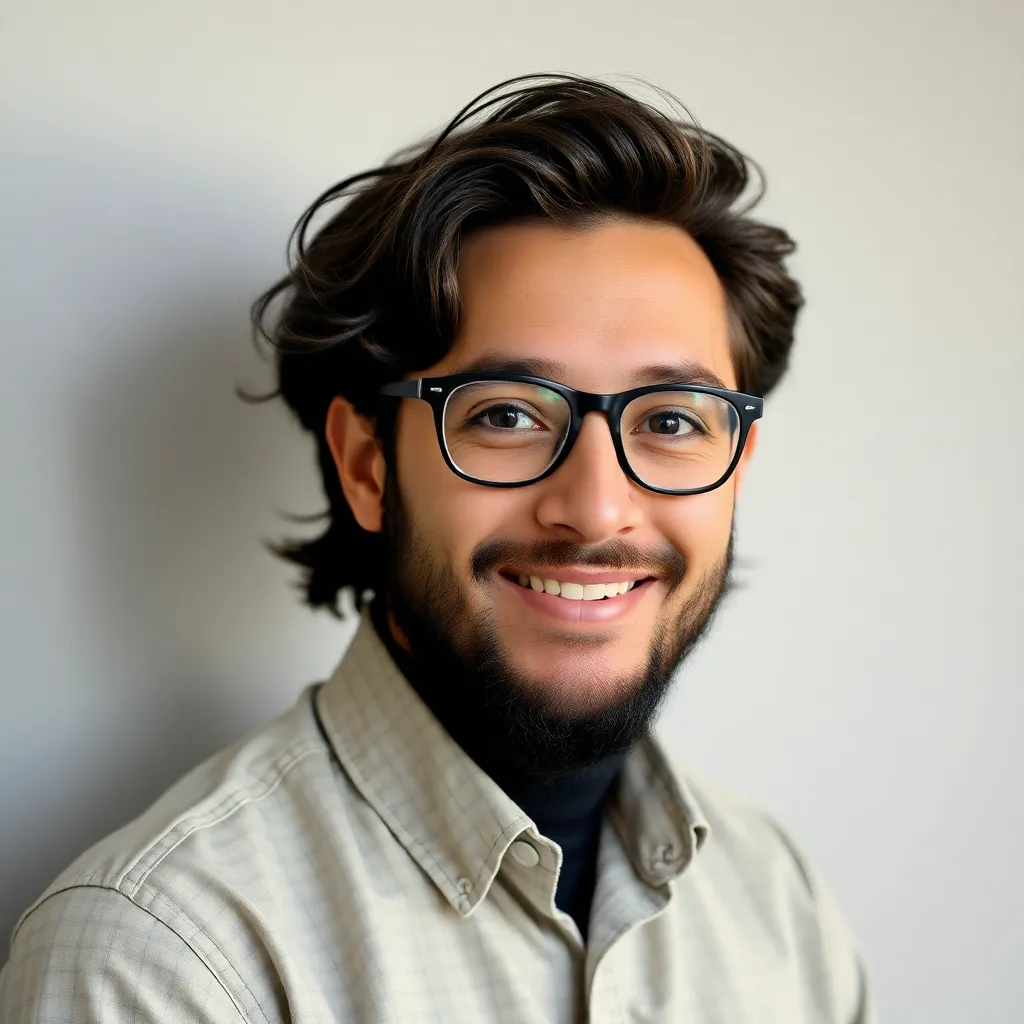
Muz Play
Apr 06, 2025 · 7 min read
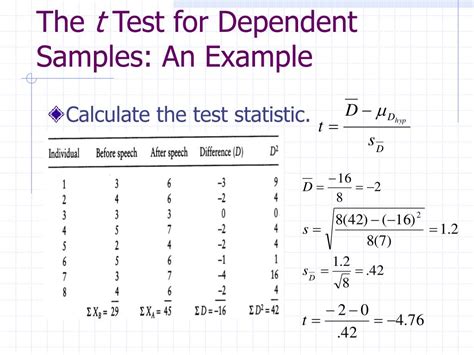
Table of Contents
Understanding and Applying the Dependent Samples t-test: Examples and Interpretations
The dependent samples t-test, also known as the paired samples t-test, is a statistical procedure used to determine if there's a significant difference between the means of two related groups. Unlike the independent samples t-test, which compares two unrelated groups, the dependent samples t-test analyzes data from the same subjects or matched pairs, measured under two different conditions or at two different time points. This makes it a powerful tool for analyzing pre- and post-intervention effects, longitudinal studies, and matched-pairs designs. This article will delve into the core concepts of the dependent samples t-test, providing clear examples and interpretations to solidify your understanding.
What is a Dependent Samples t-test?
At its core, the dependent samples t-test assesses whether the mean difference between paired observations is significantly different from zero. This "mean difference" represents the average change observed between the two conditions. A significant result suggests that the intervention or change in condition has a statistically significant impact. The test's power stems from its ability to control for individual differences between subjects, leading to a more precise estimate of the treatment effect. This is in stark contrast to the independent samples t-test, which struggles with this individual variation.
Key Assumptions of the Dependent Samples t-test
Before applying the dependent samples t-test, it's crucial to ensure the following assumptions are met:
- Data is paired: The observations must be paired or matched in some way. This could be the same subjects measured twice (e.g., before and after treatment) or matched pairs of subjects (e.g., twins, matched on relevant characteristics).
- Data is approximately normally distributed: While the t-test is relatively robust to violations of normality, especially with larger sample sizes, significant deviations from normality can affect the results. Visual inspection of the distribution (histograms, Q-Q plots) or formal normality tests (Shapiro-Wilk test) can be used to assess this.
- Data is continuous: The dependent variable must be measured on a continuous scale (e.g., weight, blood pressure, test scores).
- Independence of differences: The differences between paired observations should be independent of each other. This assumption is usually met if the sampling procedure is appropriate.
Examples of Dependent Samples t-test Applications
Let's explore several examples to illustrate the diverse applications of this powerful statistical tool:
Example 1: Pre- and Post-Treatment Comparison
Imagine a researcher studying the effectiveness of a new weight loss program. They measure the weight of 20 participants before the program begins (pre-treatment) and again after eight weeks (post-treatment). The dependent samples t-test can determine if there's a statistically significant difference in weight between the pre- and post-treatment measurements. A significant result would suggest the program is effective in promoting weight loss.
Data Structure:
Participant | Pre-Treatment Weight (kg) | Post-Treatment Weight (kg) | Difference (Post - Pre) |
---|---|---|---|
1 | 85 | 78 | -7 |
2 | 92 | 85 | -7 |
3 | 78 | 72 | -6 |
... | ... | ... | ... |
20 | 100 | 90 | -10 |
The analysis focuses on the "Difference" column. The t-test assesses if the average difference is significantly different from zero.
Example 2: Comparing Two Different Treatments on the Same Subjects
A clinical trial is evaluating two different pain-relieving medications. Participants receive both medications, with a washout period between treatments to minimize carryover effects. Pain levels are measured after each medication. The dependent samples t-test compares the average pain relief achieved by the two medications within the same participants.
Data Structure:
Participant | Medication A Pain Score | Medication B Pain Score | Difference (B - A) |
---|---|---|---|
1 | 7 | 5 | -2 |
2 | 8 | 6 | -2 |
3 | 6 | 4 | -2 |
... | ... | ... | ... |
20 | 9 | 7 | -2 |
Again, the focus is on the difference scores to determine if one medication provides significantly more pain relief than the other.
Example 3: Matched-Pairs Design
Researchers are interested in comparing the effectiveness of two different teaching methods. They create matched pairs of students based on their prior academic performance. One student from each pair is randomly assigned to method A, and the other to method B. Their final exam scores are then compared using a dependent samples t-test. The matching controls for pre-existing differences in student abilities, making the comparison more precise.
Data Structure:
Pair | Student 1 (Method A) Score | Student 2 (Method B) Score | Difference (A - B) |
---|---|---|---|
1 | 85 | 80 | 5 |
2 | 92 | 88 | 4 |
3 | 78 | 75 | 3 |
... | ... | ... | ... |
10 | 95 | 90 | 5 |
The analysis focuses on the difference in scores between students within each matched pair.
Interpreting the Results of a Dependent Samples t-test
The output of a dependent samples t-test typically includes:
- t-statistic: This value indicates the magnitude of the difference between the means relative to the variability within the data.
- Degrees of freedom (df): This is calculated as n - 1, where n is the number of pairs.
- p-value: This probability indicates the likelihood of observing the obtained results (or more extreme results) if there were no actual difference between the means.
- Confidence interval: This provides a range of values within which the true population mean difference is likely to fall.
Interpreting the p-value:
- p-value ≤ alpha (typically 0.05): The results are statistically significant. This means there is sufficient evidence to reject the null hypothesis (that there is no difference between the means) and conclude that a significant difference exists between the paired observations.
- p-value > alpha: The results are not statistically significant. This means there is insufficient evidence to reject the null hypothesis. It's important to note that this doesn't necessarily mean there is no difference; it simply means the study didn't find enough evidence to conclude a significant difference exists.
Choosing Between Dependent and Independent Samples t-tests
It's crucial to select the appropriate t-test based on the nature of the data. The choice hinges on whether the samples are independent or dependent:
- Independent samples t-test: Use this test when comparing the means of two unrelated groups. For example, comparing the average height of men and women.
- Dependent samples t-test: Use this test when comparing the means of two related groups, such as pre- and post-treatment measurements on the same subjects or matched pairs.
Choosing the incorrect test can lead to inaccurate conclusions. The dependent samples t-test's advantage lies in its ability to control for individual variability, which increases statistical power and the precision of results.
Beyond the Basics: Effect Size and Power Analysis
While statistical significance (p-value) is important, it's not the whole story. Consider these additional aspects for a complete interpretation:
-
Effect Size: This quantifies the magnitude of the difference between the means. Common effect size measures include Cohen's d, which expresses the difference in standard deviation units. A larger effect size indicates a more substantial practical significance, regardless of the p-value.
-
Power Analysis: Before conducting the study, a power analysis can determine the necessary sample size to detect a meaningful effect size with a desired level of confidence. This helps avoid underpowered studies that may fail to detect a real effect.
Conclusion
The dependent samples t-test is a versatile and valuable tool for analyzing paired data. By understanding its assumptions, applications, and interpretation, researchers can effectively evaluate the impact of interventions, treatments, or changes in conditions. Remember to always consider effect size and power analysis to enhance the robustness and comprehensiveness of your findings. Proper application of the dependent samples t-test contributes significantly to sound scientific conclusions and evidence-based decision-making across various disciplines. Mastering this test is crucial for anyone working with paired data and seeking reliable insights.
Latest Posts
Latest Posts
-
What Does Composition Mean In Chemistry
Apr 07, 2025
-
Adolf Hitler Was A Great Leader
Apr 07, 2025
-
Identify The Resulting Resonance Structure Indicated By The Curved Arrows
Apr 07, 2025
-
How Do You Determine If A Function Has An Inverse
Apr 07, 2025
-
Can P Orbitals Form Sigma Bonds
Apr 07, 2025
Related Post
Thank you for visiting our website which covers about Example Of Dependent Samples T Test . We hope the information provided has been useful to you. Feel free to contact us if you have any questions or need further assistance. See you next time and don't miss to bookmark.