Example Of Linear Programming In Real Life
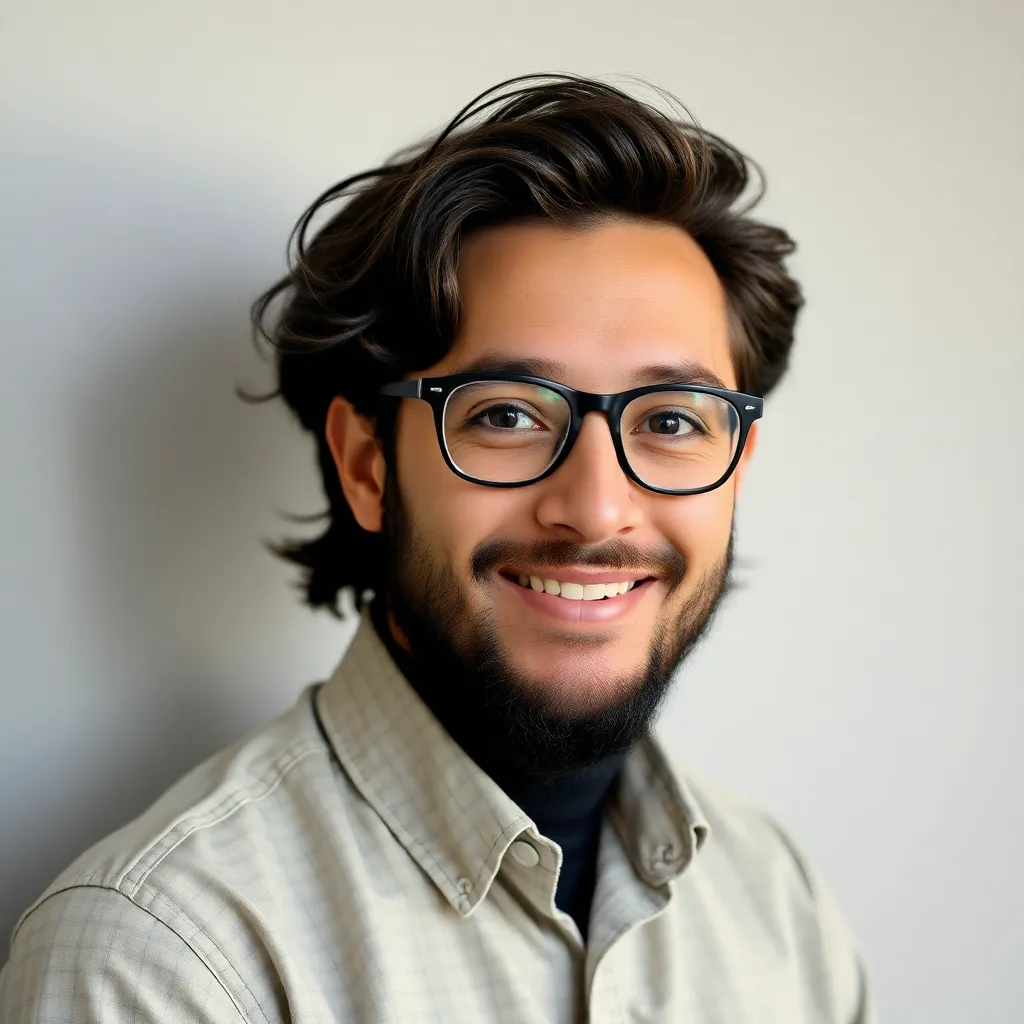
Muz Play
Apr 08, 2025 · 6 min read

Table of Contents
Real-Life Examples of Linear Programming: Optimizing Your World
Linear programming (LP) is a powerful mathematical method used to achieve the best outcome (such as maximum profit or lowest cost) in a mathematical model whose requirements are represented by linear relationships. While it might sound abstract, LP underpins countless decisions in the real world, impacting everything from manufacturing and logistics to finance and healthcare. This article dives deep into several real-life applications of linear programming, illustrating its versatility and practical impact.
Understanding the Core Concepts of Linear Programming
Before delving into examples, let's briefly revisit the fundamental elements of linear programming. At its heart, LP involves:
-
Objective Function: This defines what you're trying to maximize or minimize (e.g., profit, cost, distance). It's expressed as a linear equation.
-
Decision Variables: These are the controllable factors you can adjust to achieve your objective (e.g., production quantity of different products, allocation of resources).
-
Constraints: These are limitations or restrictions imposed on the decision variables (e.g., limited raw materials, available labor hours, production capacity). These are also expressed as linear inequalities or equations.
-
Non-negativity Constraints: Decision variables typically cannot have negative values (e.g., you can't produce a negative number of cars).
Real-World Applications of Linear Programming
Now, let's explore some compelling real-life examples where linear programming finds its application:
1. Production Planning and Optimization
Imagine a furniture manufacturer producing chairs and tables. Each chair requires a certain amount of wood and labor, as does each table. The manufacturer has a limited supply of wood and a fixed number of labor hours available. Using linear programming, the manufacturer can determine the optimal number of chairs and tables to produce to maximize profit, given the constraints of available resources. The objective function would be to maximize profit (profit per chair * number of chairs + profit per table * number of tables), subject to constraints on wood and labor.
Key Variables: Number of chairs produced, number of tables produced.
Objective Function: Maximize Profit
Constraints: Limited wood supply, limited labor hours, non-negativity constraints.
2. Transportation and Logistics
Logistics companies use linear programming extensively to optimize their delivery routes and minimize transportation costs. Consider a company with multiple warehouses and numerous delivery locations. Linear programming can determine the most efficient way to transport goods from warehouses to customers, considering factors like distance, transportation costs, and delivery deadlines. The objective function would be to minimize the total transportation cost, subject to constraints such as vehicle capacity and delivery time windows.
Key Variables: Amount of goods transported from each warehouse to each location.
Objective Function: Minimize Total Transportation Cost
Constraints: Warehouse capacity, vehicle capacity, demand at each location, delivery time windows, non-negativity constraints.
3. Portfolio Optimization in Finance
In the world of finance, linear programming plays a crucial role in portfolio optimization. Investors aim to build a portfolio of assets (stocks, bonds, etc.) that maximizes return while minimizing risk. Linear programming can help determine the optimal allocation of funds among different assets, considering factors like expected returns, risk levels, and investment constraints. The objective function could be to maximize expected return, subject to constraints on risk tolerance and available capital.
Key Variables: Amount of investment in each asset.
Objective Function: Maximize Expected Return (while minimizing risk)
Constraints: Total investment budget, risk tolerance limits, diversification requirements, non-negativity constraints.
4. Diet Optimization
Linear programming can even help you plan a healthy and cost-effective diet! By defining the nutritional requirements (e.g., minimum daily intake of vitamins, proteins, carbohydrates) and the cost and nutritional content of various foods, linear programming can determine the optimal combination of foods to meet those requirements at the lowest cost.
Key Variables: Quantity of each food item to consume.
Objective Function: Minimize total cost
Constraints: Minimum daily intake of nutrients, calorie limits, budget constraints, non-negativity constraints.
5. Blending Problems in the Chemical Industry
The chemical industry extensively uses linear programming for blending problems. For instance, a company might need to produce a specific type of gasoline with certain octane ratings and other properties by blending different crude oils. Linear programming can determine the optimal proportions of each crude oil to blend to meet the desired specifications at the minimum cost.
Key Variables: Amount of each crude oil used in the blend.
Objective Function: Minimize blending cost.
Constraints: Desired octane rating, other property requirements, availability of crude oils, non-negativity constraints.
6. Cutting Stock Problem
In industries like textiles and paper, the cutting stock problem is common. Large rolls of material need to be cut into smaller pieces to meet specific customer orders. Linear programming can optimize the cutting process to minimize waste and maximize the utilization of the raw material.
Key Variables: Number of times each cutting pattern is used.
Objective Function: Minimize waste (or maximize material utilization).
Constraints: Customer order requirements, roll dimensions, non-negativity constraints.
7. Workforce Scheduling
Optimizing workforce scheduling is crucial for businesses to ensure adequate staffing levels while minimizing labor costs. Linear programming can be used to create work schedules that meet demand, comply with labor laws (e.g., maximum working hours per week), and consider employee preferences.
Key Variables: Number of employees assigned to each shift.
Objective Function: Minimize labor cost (or maximize employee satisfaction).
Constraints: Demand for employees at different times, employee availability, labor laws, non-negativity constraints.
8. Assignment Problems
Linear programming finds application in assignment problems, where tasks need to be assigned to individuals or machines to optimize efficiency. For example, assigning workers to different jobs based on their skills and availability, minimizing the total time required to complete all tasks.
Key Variables: Assignment of each worker to each task.
Objective Function: Minimize total time to complete all tasks.
Constraints: Each worker can only be assigned to one task, each task must be assigned to one worker, worker skills and availability, non-negativity constraints.
9. Network Flow Problems
Network flow problems involve optimizing the flow of goods or information through a network. Examples include routing traffic in a communication network, managing water flow in a pipeline system, or scheduling flights in an airline network. Linear programming helps find the optimal flow to minimize congestion, maximize throughput, or minimize costs.
Key Variables: Flow along each arc in the network.
Objective Function: Maximize flow (or minimize cost).
Constraints: Capacity constraints on arcs, flow conservation at nodes, non-negativity constraints.
10. Facility Location Problems
Businesses often use linear programming to determine the optimal location for new facilities, considering factors such as proximity to customers, transportation costs, and the availability of resources. The goal is to minimize overall costs or maximize service coverage.
Key Variables: Location of facilities.
Objective Function: Minimize total cost (or maximize coverage).
Constraints: Distance to customers, resource availability, capacity constraints, non-negativity constraints.
Conclusion: The Expanding Reach of Linear Programming
Linear programming is a remarkably versatile tool with applications far exceeding those listed above. Its ability to optimize complex systems under constraints makes it an indispensable technique in various fields. As computational power continues to increase and algorithms improve, the scope of linear programming's application will only continue to expand, helping businesses and individuals make better, more informed decisions. Understanding the fundamental principles and appreciating the diverse real-world applications of linear programming provides valuable insights into its importance in shaping our modern world. From optimizing production lines to managing financial portfolios, LP is a silent but powerful force driving efficiency and innovation across multiple sectors.
Latest Posts
Latest Posts
-
Where Do Organisms Get The Energy They Need To Survive
Apr 16, 2025
-
Organic Compounds Composed Of Carbon Hydrogen And Oxygen
Apr 16, 2025
-
How Enzymes Function Effect Of Concentration
Apr 16, 2025
-
Determine Four Ways Of Expressing A Relation
Apr 16, 2025
-
What Makes Amino Acids Different From Each Other
Apr 16, 2025
Related Post
Thank you for visiting our website which covers about Example Of Linear Programming In Real Life . We hope the information provided has been useful to you. Feel free to contact us if you have any questions or need further assistance. See you next time and don't miss to bookmark.