F Test Two Sample For Variances
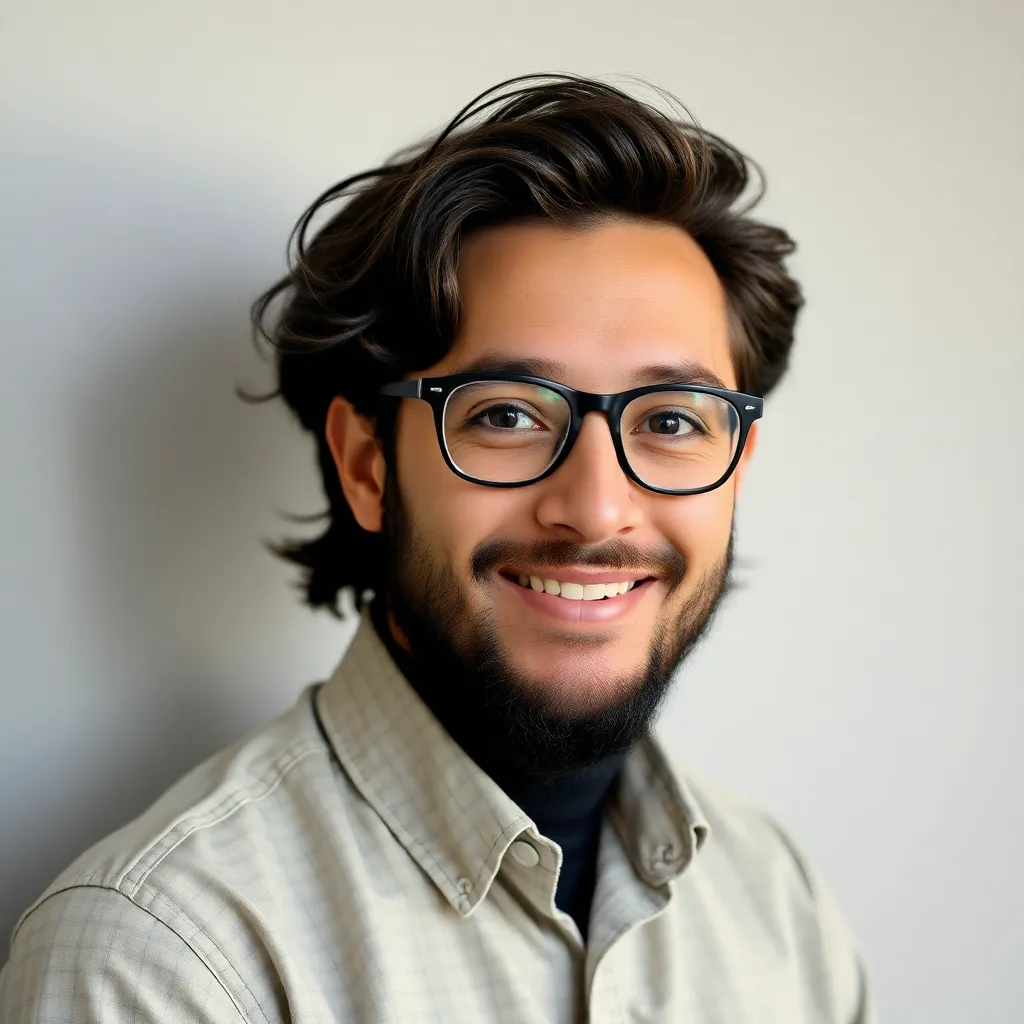
Muz Play
Apr 27, 2025 · 7 min read

Table of Contents
F-Test for Two Sample Variances: A Comprehensive Guide
The F-test for two sample variances is a statistical test used to determine if the variances of two populations are significantly different. Unlike t-tests which focus on means, the F-test focuses on the spread or dispersion of data. This test is crucial in various fields, including engineering, finance, and healthcare, where understanding the variability of data is as important, if not more so, than understanding the average. This comprehensive guide will delve into the intricacies of the F-test, covering its assumptions, calculations, interpretations, and practical applications.
Understanding the F-Distribution
Before diving into the specifics of the F-test, it's crucial to grasp the concept of the F-distribution. The F-distribution is a probability distribution that arises frequently in statistical hypothesis testing, particularly when dealing with ratios of variances. It's characterized by two parameters: degrees of freedom for the numerator (df1) and degrees of freedom for the denominator (df2). These degrees of freedom are related to the sample sizes of the two groups being compared. The shape of the F-distribution is positively skewed, meaning it has a longer tail on the right side. The exact shape is determined by the degrees of freedom. Understanding the F-distribution is pivotal because the F-statistic calculated in the test is compared against this distribution to determine significance.
Assumptions of the F-Test for Two Sample Variances
The validity and reliability of the F-test depend heavily on several crucial assumptions. Violating these assumptions can lead to inaccurate conclusions. These assumptions are:
1. Independence of Samples:
The two samples being compared must be independent. This means that the data points in one sample should not be related to or influence the data points in the other sample. If there's any correlation between the samples, the F-test results will be unreliable.
2. Normality of Data:
The F-test is robust to departures from normality, especially with larger sample sizes. However, ideally, both populations from which the samples are drawn should be approximately normally distributed. Severe deviations from normality, especially with smaller sample sizes, can affect the accuracy of the test. Tests like the Shapiro-Wilk test can be used to assess normality.
3. Homogeneity of Variances (for some variations of the test):
While the F-test itself is designed to compare variances, some variations of the test (e.g., when using it as a precursor to ANOVA) assume that the variances are equal if the null hypothesis is true. Violating this assumption can compromise the results. Levene's test can be used to assess the homogeneity of variances before performing the F-test.
Steps in Conducting an F-Test for Two Sample Variances
The F-test involves a series of steps to determine whether the variances are significantly different:
1. State the Hypotheses:
The null hypothesis (H0) states that the variances of the two populations are equal. The alternative hypothesis (H1) states that the variances are not equal. This can be a two-tailed test (variances are unequal) or a one-tailed test (variance of one population is greater than the variance of the other).
- H0: σ1² = σ2²
- H1: σ1² ≠ σ2² (Two-tailed)
- H1: σ1² > σ2² (One-tailed)
- H1: σ1² < σ2² (One-tailed)
2. Calculate the F-Statistic:
The F-statistic is calculated as the ratio of the larger sample variance to the smaller sample variance.
- F = s1²/s2² (where s1² is the larger sample variance and s2² is the smaller sample variance)
3. Determine the Degrees of Freedom:
The degrees of freedom for the numerator (df1) is the sample size of the group with the larger variance minus 1 (n1 - 1). The degrees of freedom for the denominator (df2) is the sample size of the group with the smaller variance minus 1 (n2 - 1).
4. Determine the Critical F-Value:
Using the calculated degrees of freedom (df1 and df2) and the chosen significance level (alpha, typically 0.05), the critical F-value is obtained from an F-distribution table or statistical software. This value defines the rejection region. If the calculated F-statistic exceeds the critical F-value, the null hypothesis is rejected.
5. Make a Decision:
- If the calculated F-statistic is greater than the critical F-value, the null hypothesis is rejected. This indicates that there is a statistically significant difference between the variances of the two populations.
- If the calculated F-statistic is less than or equal to the critical F-value, the null hypothesis is not rejected. This means there is not enough evidence to conclude that the variances are significantly different.
Interpreting the Results
The interpretation of the F-test results depends on whether the null hypothesis is rejected or not. A rejection of the null hypothesis suggests a significant difference in variances, implying that the variability in one population is substantially greater than the other. This information can be crucial in various contexts. For example, in manufacturing, a significant difference in variances might indicate inconsistencies in the production process. In finance, it could highlight differences in the risk associated with two investment options.
Practical Applications of the F-Test for Two Sample Variances
The F-test for two sample variances finds wide application in diverse fields. Some prominent examples include:
- Quality Control: Assessing the consistency of a manufacturing process by comparing the variances of measurements from different batches or machines.
- Experimental Design: Determining whether the variances of treatment groups in an experiment are homogeneous, a prerequisite for many analysis of variance (ANOVA) tests.
- Finance: Comparing the volatility of two different stocks or investment portfolios. Higher variance implies higher risk.
- Healthcare: Evaluating the variability of patient responses to different treatments or the variability of diagnostic measurements across different laboratories.
- Environmental Science: Comparing the variability of pollutant levels at different locations or time periods.
Limitations of the F-Test
While a powerful tool, the F-test has certain limitations:
- Sensitivity to Non-Normality: Although reasonably robust, the F-test can be sensitive to significant deviations from normality, especially with small sample sizes. Transformations of the data or non-parametric alternatives might be considered in such cases.
- Assumption of Equal Variances (in some contexts): Some applications of the F-test assume equal variances under the null hypothesis. Violating this assumption can lead to inaccurate results. Levene's test can help assess this assumption.
- Focus on Variances Only: The F-test solely focuses on the comparison of variances. It doesn't provide information about the means or other aspects of the data distributions.
Alternatives to the F-Test
In situations where the assumptions of the F-test are severely violated, or when a non-parametric approach is preferred, several alternatives exist:
- Levene's Test: Used to assess the equality of variances before performing other tests, like ANOVA, that assume homogeneity of variances.
- Brown-Forsythe Test: A more robust alternative to Levene's test, less sensitive to deviations from normality.
- Non-parametric tests: For non-normal data, non-parametric tests like the Siegel-Tukey test or Mood's median test can be used to compare variances. These tests are less powerful than the F-test if the normality assumption holds.
Conclusion
The F-test for two sample variances is a fundamental statistical tool for comparing the variability of two populations. Its applications span numerous fields, providing valuable insights into data dispersion. Understanding its assumptions, calculations, and interpretations is crucial for accurate and meaningful results. However, researchers should always be mindful of its limitations and consider alternative tests if the assumptions are not met. Remembering that statistical significance does not always imply practical significance is paramount in interpreting the results within the broader context of the research question. Proper application of the F-test, combined with careful consideration of its limitations and alternatives, ensures robust and reliable statistical inference.
Latest Posts
Latest Posts
-
Which Pairs Of Statements Are Logically Equivalent Select Two Options
Apr 28, 2025
-
What Is A Series Of Events That Cells Go Through
Apr 28, 2025
-
An Example Of A Base Is
Apr 28, 2025
-
Calcium Carbonate Is Organic Or Inorganic
Apr 28, 2025
-
What Is True Of All Atoms
Apr 28, 2025
Related Post
Thank you for visiting our website which covers about F Test Two Sample For Variances . We hope the information provided has been useful to you. Feel free to contact us if you have any questions or need further assistance. See you next time and don't miss to bookmark.