Fill In The Missing Values For This Anova Summary Table
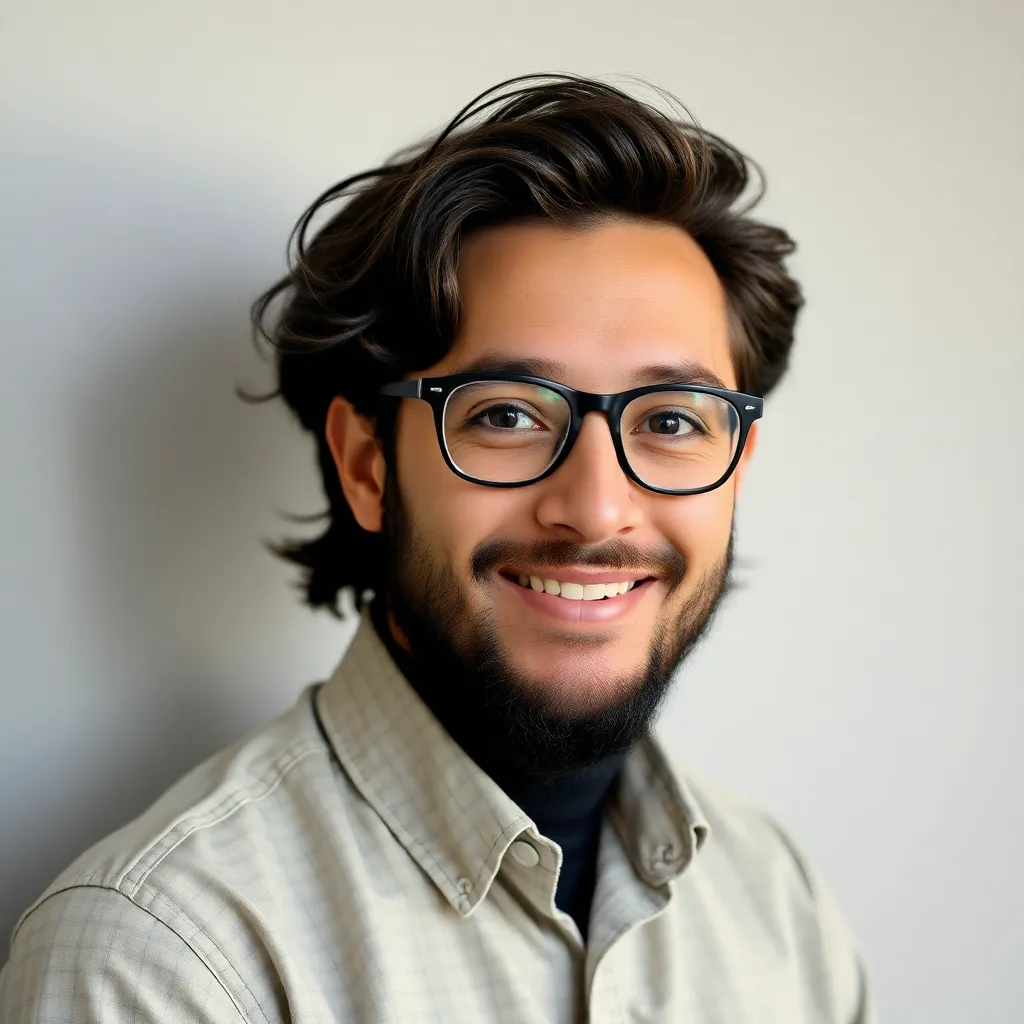
Muz Play
Apr 19, 2025 · 6 min read

Table of Contents
Filling in the Missing Values for an ANOVA Summary Table: A Comprehensive Guide
Analyzing variance (ANOVA) is a crucial statistical tool used to compare means across different groups. The ANOVA summary table is the cornerstone of interpreting these analyses, summarizing key information about the variability within and between groups. However, sometimes you'll encounter an ANOVA table with missing values. This guide will walk you through how to calculate and fill in those missing pieces, ensuring you can fully understand your ANOVA results.
Understanding the ANOVA Summary Table
Before diving into missing values, let's review the structure of a typical ANOVA summary table. It typically includes the following columns:
-
Source of Variation: This column identifies the source of the variability in your data. Key sources include "Between Groups" (or "Treatment"), representing the variation between the different groups you're comparing, and "Within Groups" (or "Error"), representing the variation within each group. A final row typically shows the "Total" variation.
-
Degrees of Freedom (df): This represents the number of independent pieces of information available to estimate a parameter. For "Between Groups," df is the number of groups minus 1 (k-1, where k is the number of groups). For "Within Groups," df is the total number of observations minus the number of groups (N-k, where N is the total number of observations). The total degrees of freedom is simply N-1.
-
Sum of Squares (SS): This represents the sum of squared deviations from the mean. It quantifies the total variation in the data.
SS_Between
measures the variation between group means and the overall mean, whileSS_Within
measures the variation within each group.SS_Total
is the sum ofSS_Between
andSS_Within
. -
Mean Square (MS): This is the average sum of squares. It's calculated by dividing the sum of squares by its corresponding degrees of freedom.
MS_Between
=SS_Between
/df_Between
, andMS_Within
=SS_Within
/df_Within
.MS_Within
is also known as the mean squared error (MSE). -
F-statistic: This is the ratio of
MS_Between
toMS_Within
. It tests the null hypothesis that there is no difference between the group means. A larger F-statistic indicates stronger evidence against the null hypothesis. -
p-value: This is the probability of observing the obtained F-statistic (or a more extreme value) if the null hypothesis is true. A small p-value (typically less than 0.05) leads to the rejection of the null hypothesis, suggesting statistically significant differences between the group means.
Common Scenarios with Missing Values
Let's explore common scenarios where you might have incomplete ANOVA tables and how to fill in the gaps:
Scenario 1: Missing Sum of Squares (SS)
If you're missing one of the Sum of Squares values (SS_Between
, SS_Within
, or SS_Total
), you can calculate it using the relationship:
SS_Total = SS_Between + SS_Within
Therefore, if you know any two of these values, you can easily calculate the third.
Scenario 2: Missing Degrees of Freedom (df)
Degrees of freedom are usually calculated directly from the experimental design. However, if you have a partial ANOVA table and the number of groups (k) or total observations (N) is unknown, you may need more information.
-
If
df_Between
is known, you can find the number of groups using:k = df_Between + 1
-
If
df_Within
andk
are known, you can find the total number of observations using:N = df_Within + k
-
If
df_Total
is known, you can use:df_Total = df_Between + df_Within
. This equation is always true, irrespective of the other values.
Scenario 3: Missing Mean Square (MS)
Mean squares are straightforward to calculate. If either SS
or df
is known, you can calculate MS
:
MS = SS / df
This applies to both MS_Between
and MS_Within
.
Scenario 4: Missing F-statistic
The F-statistic is the ratio of the mean squares:
F = MS_Between / MS_Within
If you have both MS_Between
and MS_Within
, calculating the F-statistic is straightforward.
Scenario 5: Missing p-value
The p-value requires a statistical software or table to determine, based on the calculated F-statistic and the degrees of freedom. You need both df_Between
and df_Within
to find the p-value. Many statistical software packages (like R, SPSS, SAS, Python's SciPy) can directly provide the p-value given the F-statistic and degrees of freedom. Online calculators are also readily available.
Example: Filling in a Partially Completed ANOVA Table
Let's illustrate with an example. Suppose you have the following partial ANOVA table:
Source of Variation | df | SS | MS | F | p-value |
---|---|---|---|---|---|
Between Groups | 2 | 60 | |||
Within Groups | 12 | ||||
Total | 14 |
Step 1: Calculate missing SS values
We know that SS_Total = SS_Between + SS_Within
. We don't have the value for SS_Within or SS_Total. However, from our df values, we know there are 14 - 2 = 12 degrees of freedom for the within-groups variation. Let's assume we are given that SS_Total = 100.
Then, SS_Within = SS_Total - SS_Between = 100 - 60 = 40
Step 2: Calculate missing MS values
Now, we calculate the mean squares:
MS_Between = SS_Between / df_Between = 60 / 2 = 30
MS_Within = SS_Within / df_Within = 40 / 12 = 3.33
Step 3: Calculate the F-statistic
F = MS_Between / MS_Within = 30 / 3.33 ≈ 9.01
Step 4: Find the p-value
To find the p-value, you would consult an F-distribution table or use statistical software. Using an F-distribution table or software with F(2, 12) = 9.01, you would find that the p-value is less than 0.01.
The completed ANOVA table would look like this:
Source of Variation | df | SS | MS | F | p-value |
---|---|---|---|---|---|
Between Groups | 2 | 60 | 30 | 9.01 | <0.01 |
Within Groups | 12 | 40 | 3.33 | ||
Total | 14 | 100 |
Advanced Scenarios and Considerations
In more complex ANOVA designs (like factorial ANOVA or repeated measures ANOVA), the calculations become more intricate. However, the fundamental principles remain the same. You'll still rely on the relationships between the sums of squares, degrees of freedom, mean squares, and the F-statistic. Always carefully consider the specific design of your ANOVA to ensure you're using the appropriate formulas. Statistical software is essential for complex ANOVA analyses; manual calculation is not feasible in these cases.
Conclusion
Filling in missing values in an ANOVA summary table is a valuable skill for any researcher or data analyst. By understanding the relationships between the different components of the table and using appropriate formulas, you can complete the table and draw valid conclusions from your ANOVA results. Remember to use statistical software for complex scenarios to ensure accuracy and efficiency. Always double-check your calculations to maintain the integrity of your analysis. The application of these methods will help in interpreting your ANOVA results effectively and accurately. Remember that the interpretation of your results should always be done in context with your research question and design.
Latest Posts
Latest Posts
-
Who Determined The Charge Of An Electron
Apr 19, 2025
-
What Do Detritus Feeders Contribute To The Carbon Cycle
Apr 19, 2025
-
What Are The Most Active Nonmetals
Apr 19, 2025
-
Identify The Axial And Equatorial Atoms In The Three Dimensional Structure
Apr 19, 2025
-
How To Find Electrons In An Isotope
Apr 19, 2025
Related Post
Thank you for visiting our website which covers about Fill In The Missing Values For This Anova Summary Table . We hope the information provided has been useful to you. Feel free to contact us if you have any questions or need further assistance. See you next time and don't miss to bookmark.