Find The Mean And Standard Deviation Of The Sampling Distribution
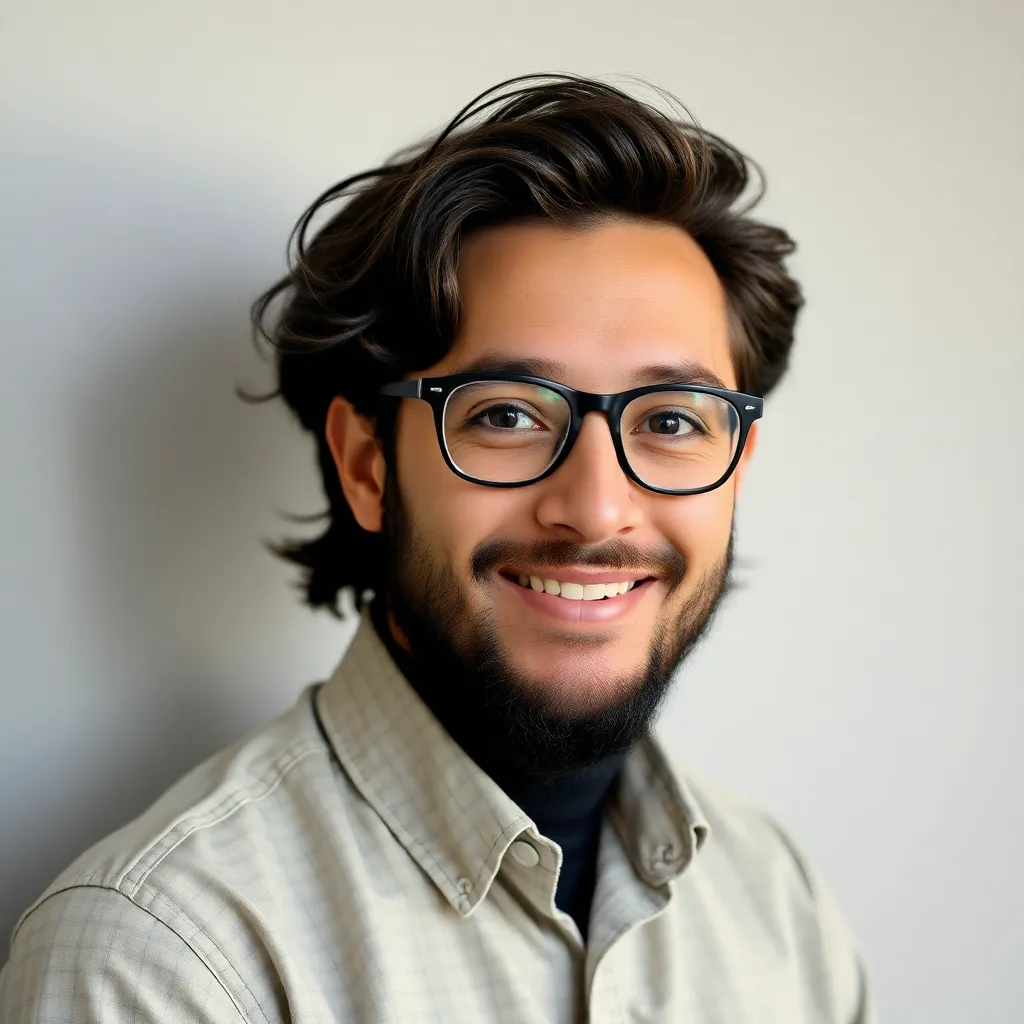
Muz Play
Apr 14, 2025 · 6 min read

Table of Contents
Find the Mean and Standard Deviation of the Sampling Distribution: A Comprehensive Guide
Understanding the sampling distribution of the mean is crucial in inferential statistics. It forms the bedrock of hypothesis testing and confidence interval estimation, allowing us to make inferences about a population based on a sample. This article provides a comprehensive guide to finding the mean and standard deviation (also known as the standard error) of the sampling distribution, explaining the underlying concepts and providing practical examples.
What is a Sampling Distribution?
Before delving into calculations, let's clarify what a sampling distribution actually is. Imagine you're interested in the average height of all adult women in a specific country. Measuring every single woman is impractical; instead, you'd take a random sample. Now, imagine taking many, many random samples (say, 1000 samples) of the same size from the same population. For each sample, you calculate the mean height. The distribution of these sample means is called the sampling distribution of the mean. It's a theoretical distribution, as we rarely take thousands of samples in practice.
Key characteristics:
- It's a distribution of sample means: Not individual data points, but the averages from multiple samples.
- It's theoretical: It represents what would happen if we took countless samples.
- It's central to inferential statistics: It allows us to estimate population parameters from sample statistics.
The Central Limit Theorem (CLT) - The Cornerstone
The Central Limit Theorem is the cornerstone of understanding sampling distributions. It states that, regardless of the shape of the population distribution (provided it has a finite mean and variance), the sampling distribution of the mean will approximate a normal distribution as the sample size (n) increases. This holds true even if the original population isn't normally distributed. The approximation becomes better as 'n' gets larger; generally, a sample size of 30 or more is considered sufficient for a good approximation.
Implications of the CLT:
- Normality: Allows us to use normal distribution properties for statistical inference.
- Predictability: Even with non-normal populations, we can predict the behavior of sample means.
- Efficiency: We can make accurate estimations about the population mean using relatively smaller samples.
Calculating the Mean of the Sampling Distribution
The mean of the sampling distribution of the mean (often denoted as μ<sub>x̄</sub>) is equal to the population mean (μ). This is a significant result. It means that the average of all possible sample means is equal to the true population mean. This makes intuitive sense: if we averaged all the sample means, we'd expect it to converge towards the true population mean.
Formula:
μ<sub>x̄</sub> = μ
Calculating the Standard Deviation of the Sampling Distribution (Standard Error)
The standard deviation of the sampling distribution is called the standard error (SE) of the mean. It measures the variability or spread of the sample means around the population mean. A smaller standard error indicates that the sample means are clustered closely around the population mean, suggesting more precise estimates. The standard error is always smaller than the population standard deviation (σ).
Formula:
SE = σ / √n
Where:
- σ: The population standard deviation.
- n: The sample size.
The formula reveals a crucial relationship:
- Inverse relationship with sample size: As the sample size (n) increases, the standard error decreases. Larger samples lead to more precise estimates of the population mean.
- Direct relationship with population standard deviation: A larger population standard deviation leads to a larger standard error, implying greater variability in the sample means.
Examples: Finding the Mean and Standard Deviation of the Sampling Distribution
Let's illustrate these concepts with examples.
Example 1: Known Population Parameters
Suppose the average weight of adult male elephants in a specific region is 6000 kg (μ = 6000 kg), with a standard deviation of 500 kg (σ = 500 kg). If we repeatedly took samples of 100 elephants (n = 100), what would be the mean and standard deviation of the sampling distribution of the mean?
- Mean of the sampling distribution (μ<sub>x̄</sub>): μ<sub>x̄</sub> = μ = 6000 kg
- Standard error (SE): SE = σ / √n = 500 kg / √100 = 50 kg
This means the average of all possible sample means would be 6000 kg, and the standard deviation of these sample means would be 50 kg.
Example 2: Unknown Population Standard Deviation – Using Sample Standard Deviation as an Estimate
Often, we don't know the population standard deviation (σ). In this case, we estimate it using the sample standard deviation (s) calculated from our sample. However, this introduces an additional layer of uncertainty. We use the sample standard deviation (s) in place of σ, but we adjust the formula for the standard error slightly, employing the t-distribution instead of the normal distribution. This adjustment accounts for the extra uncertainty introduced by estimating σ from the sample.
Let's say we take a sample of 35 elephants and find the sample mean to be 6100 kg and the sample standard deviation to be 550 kg.
- Estimated Mean of the sampling distribution: The sample mean (6100 kg) is our best estimate of the population mean.
- Estimated Standard error (using t-distribution): The formula for the standard error remains largely the same, but the choice of distribution changes to account for the additional uncertainty. The standard error calculation is: SE = s / √n = 550 kg / √35 ≈ 92.8 kg
In this scenario, because the population standard deviation is unknown, the 92.8kg standard error is an estimate. Further calculations involving confidence intervals or hypothesis testing would involve using the t-distribution and its associated degrees of freedom (n-1 = 34 in this case).
Implications for Statistical Inference
The mean and standard deviation of the sampling distribution are fundamental for hypothesis testing and confidence intervals.
- Hypothesis testing: We compare the sample mean to a hypothesized population mean using the standard error to determine the probability of observing the sample mean if the null hypothesis were true.
- Confidence intervals: We construct confidence intervals around the sample mean, using the standard error to define the margin of error. The interval provides a range of plausible values for the population mean.
Conclusion
Understanding the sampling distribution, its mean, and its standard deviation (standard error) is crucial for conducting valid statistical inferences. The Central Limit Theorem assures us that even with non-normal populations, the sampling distribution of the mean tends towards normality as the sample size increases. Being able to calculate the mean and standard error allows us to accurately estimate population parameters and make reliable conclusions based on sample data. Remember to account for the difference between using the known population standard deviation and having to estimate it from sample data, which involves the t-distribution. This knowledge empowers researchers and data analysts to interpret data effectively and draw meaningful conclusions.
Latest Posts
Latest Posts
-
What Are The Three Most Common Shapes Of Bacterial Cells
May 09, 2025
-
What Is Inertia Measured In Units
May 09, 2025
-
Controls The Movement Of The Slide On The Stage
May 09, 2025
-
What Effect Does An Enzyme Have On Ea
May 09, 2025
-
Is Tap Water A Pure Substance
May 09, 2025
Related Post
Thank you for visiting our website which covers about Find The Mean And Standard Deviation Of The Sampling Distribution . We hope the information provided has been useful to you. Feel free to contact us if you have any questions or need further assistance. See you next time and don't miss to bookmark.