Finding Area Under A Normal Curve
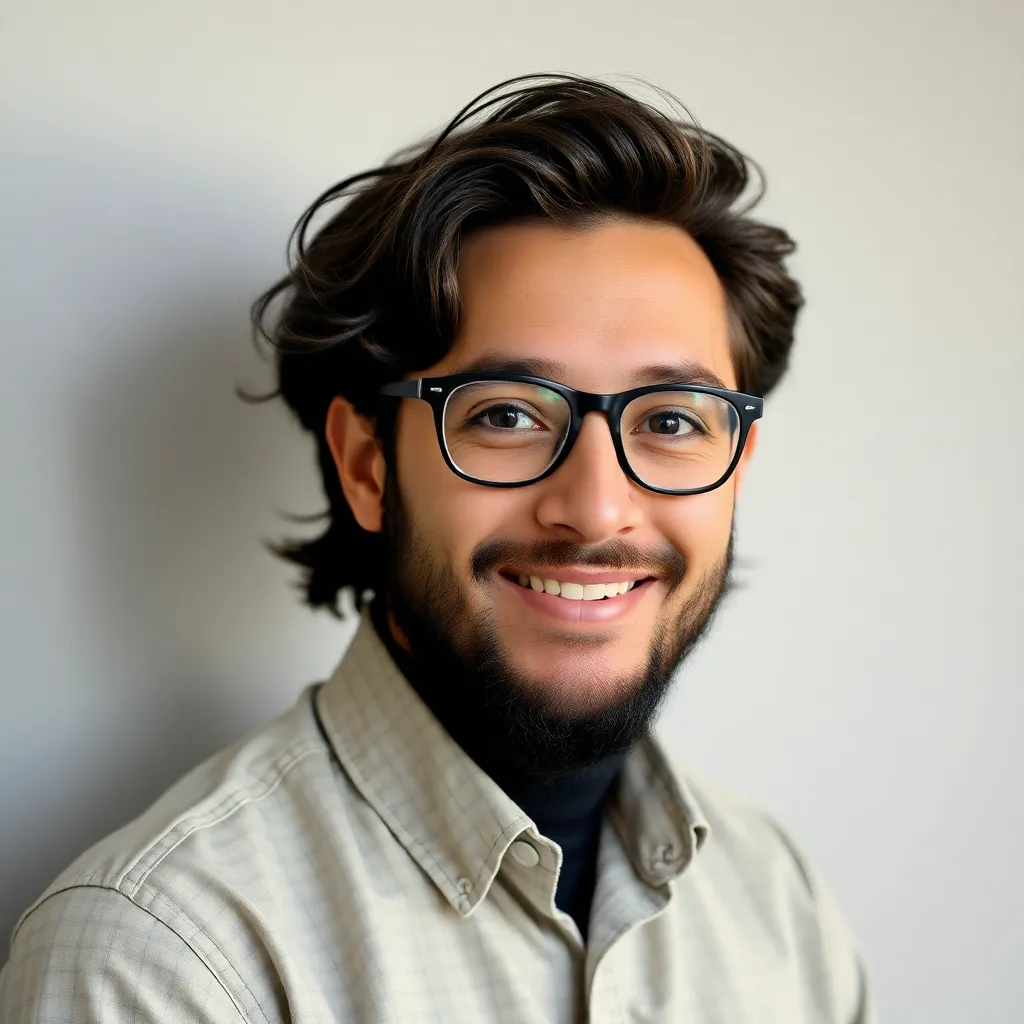
Muz Play
Apr 24, 2025 · 6 min read

Table of Contents
Finding the Area Under a Normal Curve: A Comprehensive Guide
The normal distribution, often called the Gaussian distribution or bell curve, is a fundamental concept in statistics. Understanding how to find the area under a normal curve is crucial for a wide range of applications, from hypothesis testing and confidence intervals to understanding probability distributions in various fields like finance, engineering, and the natural sciences. This comprehensive guide will delve into the methods and techniques used to calculate this crucial area, catering to both beginners and those seeking a more advanced understanding.
Understanding the Normal Distribution
Before we dive into the calculations, let's solidify our understanding of the normal distribution itself. Characterized by its symmetrical, bell-shaped curve, the normal distribution is defined by two parameters:
- Mean (μ): This represents the average or central tendency of the distribution. It's the point at the peak of the bell curve.
- Standard Deviation (σ): This measures the spread or dispersion of the data. A larger standard deviation indicates a wider, flatter curve, while a smaller standard deviation results in a taller, narrower curve.
The area under the entire normal curve always equals 1, representing 100% probability. This is a key property that underpins all calculations related to finding probabilities using the normal distribution.
Methods for Finding the Area Under the Curve
There are several methods for calculating the area under a normal curve, each with its own strengths and limitations:
1. Using the Z-Score and Z-Table
This is the most common and perhaps the most fundamental method. The z-score standardizes a data point by indicating how many standard deviations it is away from the mean. The formula for calculating the z-score is:
z = (x - μ) / σ
Where:
- x is the data point
- μ is the population mean
- σ is the population standard deviation
Once you have the z-score, you can consult a z-table (also known as a standard normal table) to find the corresponding area under the curve to the left of that z-score. The z-table provides the cumulative probability, which is the probability of observing a value less than or equal to the given z-score.
Example: Let's say you have a normal distribution with μ = 50 and σ = 10. You want to find the probability of observing a value less than 60.
- Calculate the z-score: z = (60 - 50) / 10 = 1
- Consult the z-table: Look up the z-score of 1.00 in the z-table. You'll find a value close to 0.8413.
- Interpretation: This means there is an 84.13% probability of observing a value less than 60 in this distribution.
To find the area between two points, you simply subtract the cumulative probabilities obtained from the z-table for each point. For example, to find the area between 40 and 60, you would find the cumulative probability for z = (40 - 50) / 10 = -1 and subtract it from the cumulative probability for z = 1.
2. Utilizing Statistical Software
Statistical software packages like R, SPSS, Python (with libraries like SciPy), and Excel provide powerful functions for calculating probabilities related to the normal distribution. These tools often offer greater precision and efficiency than using a z-table, particularly when dealing with complex calculations or a large number of data points. They can directly calculate the area under the curve for a given range or probability.
3. Employing the Empirical Rule (68-95-99.7 Rule)
This rule provides a quick approximation of the area under the curve for specific ranges around the mean. It states that:
- Approximately 68% of the data falls within one standard deviation of the mean (μ ± σ).
- Approximately 95% of the data falls within two standard deviations of the mean (μ ± 2σ).
- Approximately 99.7% of the data falls within three standard deviations of the mean (μ ± 3σ).
This rule is useful for quick estimations, but it's less precise than using z-tables or software for more specific calculations.
4. Numerical Integration Techniques
For more advanced scenarios or when dealing with non-standard normal distributions, numerical integration techniques can be employed. These methods approximate the area under the curve using numerical algorithms. Methods like the trapezoidal rule or Simpson's rule can be used to achieve a high degree of accuracy. However, these methods are generally more complex and require a strong understanding of numerical analysis.
Applications of Finding the Area Under the Normal Curve
The ability to calculate the area under a normal curve is essential in numerous statistical applications:
- Hypothesis Testing: Determining the p-value, which represents the probability of observing the obtained results if the null hypothesis is true.
- Confidence Intervals: Constructing intervals that are likely to contain the true population parameter with a specified level of confidence.
- Probability Calculations: Assessing the likelihood of events falling within specific ranges of a normal distribution.
- Process Control: Monitoring and controlling industrial processes by analyzing the variability of measurements.
- Risk Management: Evaluating and managing financial risks using probability distributions.
- Quality Control: Assessing the quality of products or services by examining the distribution of their characteristics.
- Medical Research: Analyzing the distribution of biological measurements, such as blood pressure or height.
- Predictive Modeling: Building models that predict future outcomes based on normally distributed variables.
Dealing with Non-Standard Normal Distributions
While the z-table and many software functions focus on the standard normal distribution (μ = 0, σ = 1), real-world data often follows a non-standard normal distribution. The key to working with these distributions is to standardize the data using the z-score transformation before looking up probabilities in the z-table or using statistical software. This standardization allows us to use the same principles and tables regardless of the mean and standard deviation of the specific normal distribution being considered.
Advanced Concepts and Considerations
- Central Limit Theorem: This theorem states that the distribution of sample means approaches a normal distribution as the sample size increases, regardless of the underlying population distribution. This is crucial for inferential statistics.
- Normal Approximation to the Binomial Distribution: For large sample sizes and probabilities not too close to 0 or 1, the binomial distribution can be approximated by a normal distribution.
- Goodness-of-Fit Tests: Tests like the chi-squared test can be used to determine whether a dataset follows a normal distribution.
Conclusion
Finding the area under a normal curve is a fundamental skill in statistics with far-reaching applications across numerous disciplines. While the z-table and the empirical rule offer valuable tools for quick estimations and understanding, statistical software packages provide greater accuracy and efficiency for complex calculations. Mastering these techniques empowers individuals to analyze data, draw meaningful conclusions, and make informed decisions in a vast array of contexts. Understanding the nuances of the normal distribution, including its parameters and its relationship to other distributions, is essential for effective data analysis and interpretation in any quantitative field. Remember to always consider the context of your data and select the appropriate method for calculating the area under the curve based on the required precision and complexity of your analysis.
Latest Posts
Latest Posts
-
How Much Of An Iceberg Is Below Water
Apr 24, 2025
-
How Is A Chemical Symbol Of An Element Determined
Apr 24, 2025
-
Everything That Is Visible When Looking Through The Eyepiece
Apr 24, 2025
-
Is This Bacterial Strain Positive Or Negative For Mannitol Fermentation
Apr 24, 2025
-
Which Pair Of Properties Describes The Elements In Group 18
Apr 24, 2025
Related Post
Thank you for visiting our website which covers about Finding Area Under A Normal Curve . We hope the information provided has been useful to you. Feel free to contact us if you have any questions or need further assistance. See you next time and don't miss to bookmark.