For The Binomial Distribution Which Formula Finds The Standard Deviation
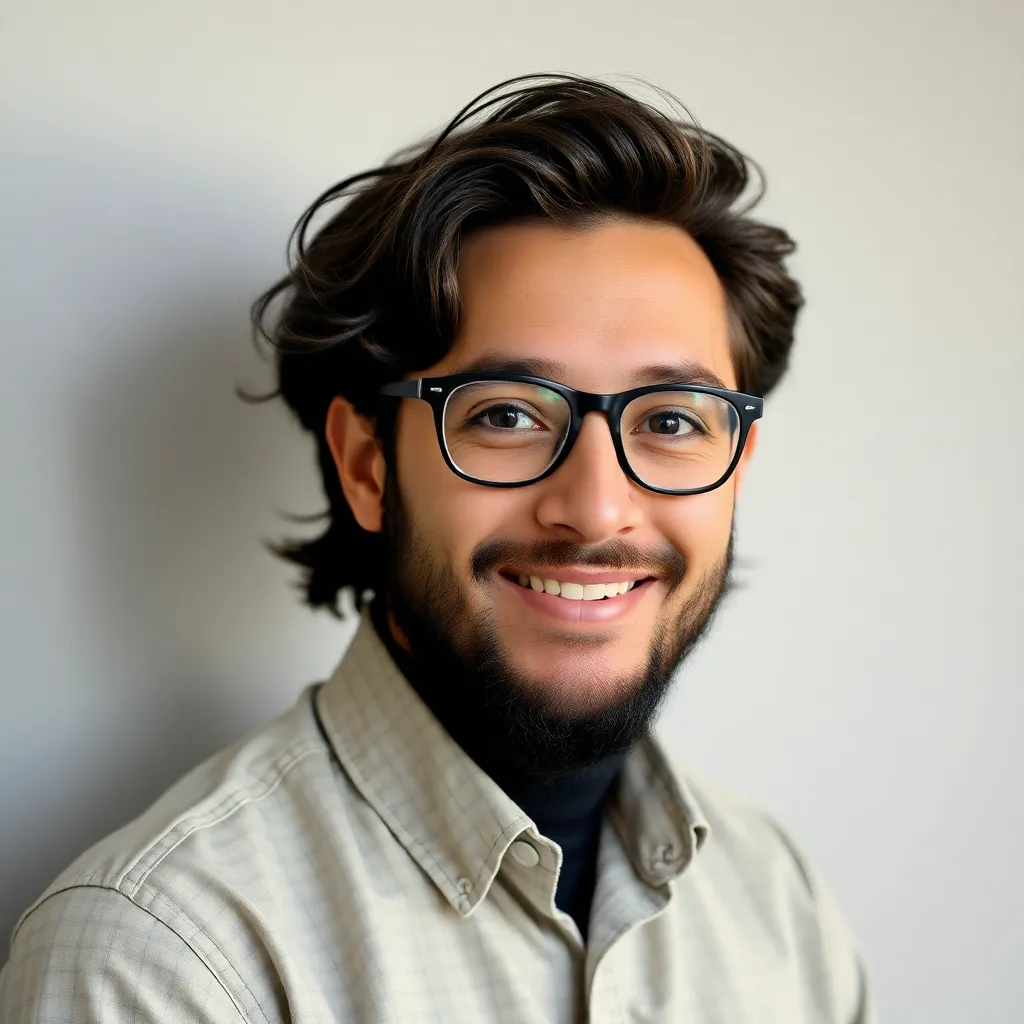
Muz Play
Apr 21, 2025 · 5 min read

Table of Contents
Decoding the Standard Deviation of the Binomial Distribution: A Comprehensive Guide
The binomial distribution, a cornerstone of probability and statistics, describes the probability of obtaining a certain number of successes in a fixed number of independent Bernoulli trials. Understanding its standard deviation is crucial for interpreting the variability inherent in binomial experiments. This comprehensive guide will delve into the formula for calculating the standard deviation of a binomial distribution, exploring its derivation, practical applications, and nuances.
Understanding the Binomial Distribution
Before diving into the standard deviation formula, let's solidify our understanding of the binomial distribution itself. A binomial experiment is characterized by:
- Fixed number of trials (n): The experiment consists of a predetermined number of independent trials.
- Independent trials: The outcome of one trial does not affect the outcome of any other trial.
- Two possible outcomes: Each trial results in one of two mutually exclusive outcomes, typically labeled "success" and "failure."
- Constant probability of success (p): The probability of success remains constant across all trials. The probability of failure is therefore (1-p), often denoted as 'q'.
The probability of obtaining exactly k successes in n trials is given by the binomial probability mass function:
P(X = k) = (nCk) * p^k * q^(n-k)
where:
- nCk represents the number of combinations of n items taken k at a time (also written as ⁿCₖ or ⍙(n,k)). This is calculated as: n! / (k! * (n-k)!)
- p is the probability of success in a single trial.
- q = 1 - p is the probability of failure in a single trial.
- k is the number of successes.
- n is the total number of trials.
The Formula for the Standard Deviation
The standard deviation (σ) of a binomial distribution is a measure of the spread or dispersion of the possible outcomes around the mean (μ). It quantifies the typical deviation of the number of successes from the expected number of successes. The formula for the standard deviation of a binomial distribution is:
σ = √(n * p * q)
or, equivalently:
σ = √(n * p * (1 - p))
Derivation of the Standard Deviation Formula
The derivation of this formula involves understanding the variance (σ²) first. Variance is the average of the squared differences from the mean. For a binomial distribution, the variance is given by:
σ² = n * p * q
This formula can be derived using the properties of expected value and variance for a sum of independent random variables. Each Bernoulli trial is a random variable with a variance of p(1-p). Since the trials are independent, the variance of the sum (which is the number of successes) is simply the sum of the variances of the individual trials, resulting in n * p * q.
The standard deviation is simply the square root of the variance, hence:
σ = √(n * p * q)
Interpreting the Standard Deviation
The standard deviation provides a crucial insight into the variability of a binomial distribution. A larger standard deviation indicates greater variability, meaning the number of successes is likely to deviate more significantly from the expected value (mean). Conversely, a smaller standard deviation signifies less variability, implying the number of successes will tend to cluster closer to the expected value.
For instance, consider two binomial distributions:
- Distribution A: n = 100, p = 0.5 => σ = √(100 * 0.5 * 0.5) = 5
- Distribution B: n = 100, p = 0.1 => σ = √(100 * 0.1 * 0.9) = 3
Distribution A has a larger standard deviation, indicating greater variability in the number of successes compared to Distribution B. This makes intuitive sense; with a probability of success of 0.5, the outcomes are more evenly spread, resulting in higher variability.
Applications of the Standard Deviation in Binomial Distributions
The standard deviation of a binomial distribution finds extensive applications in diverse fields, including:
-
Quality Control: In manufacturing, the binomial distribution can model the number of defective items in a batch. The standard deviation helps determine the acceptable range of defects. A larger standard deviation indicates a less consistent manufacturing process.
-
Medical Research: In clinical trials, the binomial distribution might model the number of patients responding positively to a treatment. The standard deviation helps assess the variability in treatment effectiveness.
-
Market Research: Surveys often rely on binomial distributions to model the proportion of people holding a particular opinion. The standard deviation quantifies the uncertainty associated with the survey results.
-
Genetics: The binomial distribution can model the probability of inheriting a specific gene. The standard deviation can help understand the variability in gene inheritance patterns within a population.
-
Risk Assessment: In finance, the binomial distribution can model the probability of success or failure of an investment. The standard deviation helps assess the risk associated with the investment.
Limitations and Considerations
While the binomial distribution is a powerful tool, it's essential to acknowledge its limitations:
-
Independence Assumption: The assumption of independent trials is crucial. If the outcome of one trial influences another, the binomial distribution is not applicable.
-
Constant Probability: The probability of success must remain constant across all trials. This assumption can be violated in certain scenarios, such as sampling without replacement from a small population.
-
Large Sample Size: The binomial distribution's approximation by the normal distribution (using the central limit theorem) becomes more accurate as the sample size increases. For small sample sizes, the approximation might be less precise.
Relationship with the Normal Approximation
For sufficiently large values of n, and when p is not too close to 0 or 1, the binomial distribution can be approximated by the normal distribution. This approximation simplifies calculations and allows us to use the properties of the normal distribution, such as the empirical rule (68-95-99.7 rule). The rule of thumb often used is that both np and n(1-p) should be greater than or equal to 5. This approximation is crucial for hypothesis testing and confidence interval estimation related to binomial proportions.
Conclusion
Understanding the standard deviation of a binomial distribution is essential for interpreting and utilizing this fundamental probability model effectively. The formula, its derivation, and its implications across various fields have been explored in this detailed guide. By grasping this concept and its limitations, you can confidently analyze and interpret data arising from binomial experiments, drawing meaningful conclusions and making informed decisions in diverse contexts. Remember to always consider the assumptions and limitations of the binomial distribution before applying it to your specific data. Proper application requires a thorough understanding of the underlying principles and the context in which the data is generated.
Latest Posts
Latest Posts
-
Factors Affecting Rate Of Chemical Reaction Lab Report
Apr 21, 2025
-
Which Rule For Assigning Oxidation Numbers Is Correct
Apr 21, 2025
-
Which Of These Characteristics First Developed In Reptiles
Apr 21, 2025
-
Open The Borders And Shading Dialog
Apr 21, 2025
-
Malate Aspartate Shuttle Vs Glycerol Phosphate Shuttle
Apr 21, 2025
Related Post
Thank you for visiting our website which covers about For The Binomial Distribution Which Formula Finds The Standard Deviation . We hope the information provided has been useful to you. Feel free to contact us if you have any questions or need further assistance. See you next time and don't miss to bookmark.