Formula For 2 Proportion Z Test
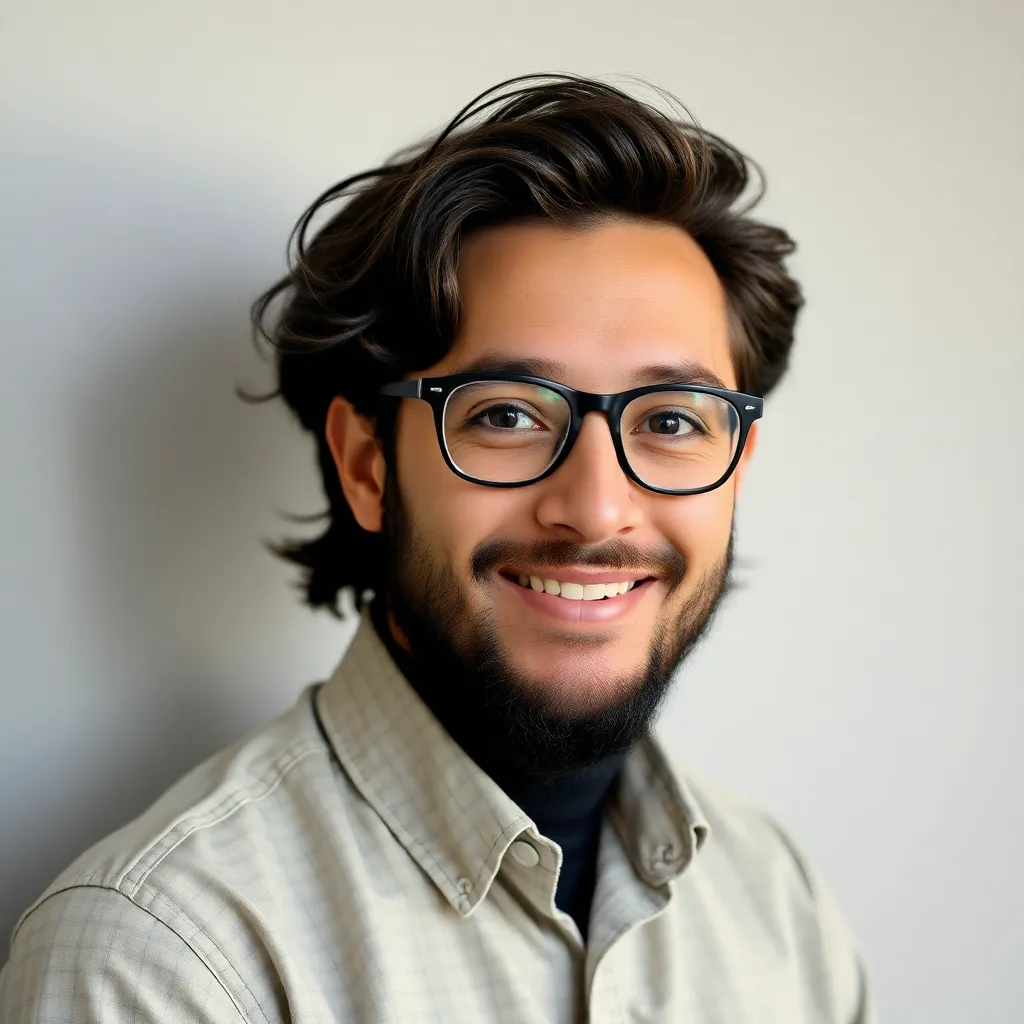
Muz Play
Mar 10, 2025 · 6 min read

Table of Contents
The Formula for a 2-Proportion Z-Test: A Deep Dive
The two-proportion z-test is a powerful statistical tool used to determine if there's a significant difference between the proportions of two independent populations. Understanding its formula and application is crucial for researchers across various fields, from healthcare and marketing to social sciences and engineering. This comprehensive guide will delve into the intricacies of the two-proportion z-test, exploring its underlying assumptions, step-by-step calculations, and practical applications.
Understanding the Core Concepts
Before diving into the formula, let's clarify some fundamental concepts:
1. Proportions:
A proportion represents the fraction of a population possessing a specific characteristic. For example, the proportion of women in a particular city, the proportion of customers preferring a certain product, or the proportion of patients responding positively to a treatment. We typically represent proportions as p.
2. Sample Proportions:
In reality, we rarely have access to the entire population. Instead, we work with sample proportions (denoted as p̂, pronounced "p-hat"). These are estimates of the population proportions, calculated from a representative sample drawn from the population.
3. Independent Samples:
The two-proportion z-test requires the samples from the two populations to be independent. This means that the selection of individuals in one sample doesn't influence the selection of individuals in the other sample. For instance, if we are comparing the effectiveness of two drugs, we need to ensure that the patients in each treatment group are distinct.
4. Null and Alternative Hypotheses:
The test revolves around two hypotheses:
- Null Hypothesis (H₀): This hypothesis assumes there's no significant difference between the two population proportions (p₁ = p₂).
- Alternative Hypothesis (H₁ or Hₐ): This hypothesis proposes a significant difference between the two population proportions (p₁ ≠ p₂, p₁ > p₂, or p₁ < p₂). The direction of the difference (≠, >, or <) dictates whether it's a two-tailed, right-tailed, or left-tailed test, respectively.
The Formula Unveiled
The core of the two-proportion z-test lies in calculating the z-statistic, which measures the difference between the sample proportions relative to the expected variation. The formula is as follows:
z = (p̂₁ - p̂₂) / √[p̂(1 - p̂)(1/n₁ + 1/n₂)]
Where:
- p̂₁: Sample proportion from population 1
- p̂₂: Sample proportion from population 2
- n₁: Sample size from population 1
- n₂: Sample size from population 2
- p̂: Pooled sample proportion, calculated as: p̂ = (x₁ + x₂) / (n₁ + n₂)
- Where x₁ is the number of successes in sample 1 and x₂ is the number of successes in sample 2.
Step-by-Step Calculation
Let's illustrate the calculation with a concrete example:
Suppose we want to compare the effectiveness of two different marketing campaigns. Campaign A resulted in 60 conversions out of 200 leads (p̂₁ = 60/200 = 0.3), while Campaign B yielded 80 conversions out of 250 leads (p̂₂ = 80/250 = 0.32). We'll perform a two-tailed test to see if there's a significant difference between the conversion rates.
Step 1: Calculate the pooled sample proportion (p̂):
p̂ = (60 + 80) / (200 + 250) = 140 / 450 ≈ 0.311
Step 2: Calculate the standard error:
Standard Error = √[p̂(1 - p̂)(1/n₁ + 1/n₂)] = √[0.311(1 - 0.311)(1/200 + 1/250)] ≈ 0.040
Step 3: Calculate the z-statistic:
z = (0.3 - 0.32) / 0.040 = -0.02 / 0.040 = -0.5
Step 4: Determine the p-value:
Using a z-table or statistical software, we find the p-value associated with a z-score of -0.5. For a two-tailed test, we double the one-tailed p-value. In this case, the p-value would be approximately 0.62.
Step 5: Draw a conclusion:
We compare the p-value to a pre-determined significance level (alpha), often set at 0.05. Since our p-value (0.62) is greater than alpha (0.05), we fail to reject the null hypothesis. This means there's not enough evidence to conclude a significant difference between the conversion rates of Campaign A and Campaign B.
Assumptions of the Two-Proportion Z-Test
The validity of the two-proportion z-test relies on several key assumptions:
- Random Sampling: Both samples must be randomly selected from their respective populations to ensure representativeness.
- Independence: The observations within each sample and between the two samples must be independent.
- Large Sample Sizes: The sample sizes should be large enough to ensure that the sampling distribution of the sample proportions is approximately normal. A common rule of thumb is that n₁p̂₁, n₁(1-p̂₁), n₂p̂₂, and n₂(1-p̂₂) should all be greater than 5. This ensures the Central Limit Theorem applies.
When to Use (and Not Use) the Two-Proportion Z-Test
The two-proportion z-test is appropriate when:
- You want to compare the proportions of two independent groups.
- Your data meets the assumptions of random sampling, independence, and large sample sizes.
- You are interested in testing for a significant difference between the two proportions.
The test is not appropriate when:
- Your samples are dependent (e.g., before-and-after measurements on the same subjects). In such cases, a paired t-test or McNemar's test would be more suitable.
- Your sample sizes are small, violating the large sample size assumption. In this scenario, Fisher's exact test might be more appropriate.
- Your data is not binary (i.e., doesn't consist of successes and failures).
Interpreting the Results
The outcome of the two-proportion z-test depends on the p-value and the chosen significance level.
- p-value ≤ alpha: Reject the null hypothesis. There is statistically significant evidence to suggest a difference between the two population proportions.
- p-value > alpha: Fail to reject the null hypothesis. There is not enough statistically significant evidence to suggest a difference between the two population proportions.
Remember that statistical significance doesn't necessarily imply practical significance. A statistically significant result might not be meaningful in a real-world context. Always consider the effect size (the magnitude of the difference between the proportions) alongside statistical significance when interpreting your findings.
Beyond the Basics: Confidence Intervals
While the z-test focuses on hypothesis testing, calculating confidence intervals provides a range of plausible values for the difference between the two population proportions. A 95% confidence interval, for example, means that we are 95% confident that the true difference between the proportions lies within that range. The formula for the confidence interval is:
(p̂₁ - p̂₂) ± Z<sub>α/2</sub> * √[p̂₁(1 - p̂₁)/n₁ + p̂₂(1 - p̂₂)/n₂]
Where Z<sub>α/2</sub> is the critical z-value corresponding to the desired confidence level (e.g., 1.96 for a 95% confidence interval).
Conclusion: A Powerful Tool for Comparison
The two-proportion z-test is a valuable tool for comparing proportions across two independent groups. By carefully following the formula and understanding its assumptions, researchers can draw meaningful conclusions from their data, informing decision-making in various fields. Remember that proper interpretation requires considering both statistical and practical significance, along with the potential limitations of the test. Always strive for rigorous data collection and analysis to ensure the validity and reliability of your findings. The understanding of this test allows for powerful statistical analysis enabling informed choices across various fields of study. Remember to always critically evaluate your results in the broader context of your research question and its practical implications.
Latest Posts
Latest Posts
-
Do Enzymes Oscillate Between Active And Inactive Forms
May 09, 2025
-
During An Isotonic Concentric Contraction The
May 09, 2025
-
Match Each Organism With The Correct Type Of Body Cavity
May 09, 2025
-
Do Enzymes Interact With Many Different Substrates
May 09, 2025
-
Can You See Bacteria With A Light Microscope
May 09, 2025
Related Post
Thank you for visiting our website which covers about Formula For 2 Proportion Z Test . We hope the information provided has been useful to you. Feel free to contact us if you have any questions or need further assistance. See you next time and don't miss to bookmark.