How To Describe Distribution In Statistics
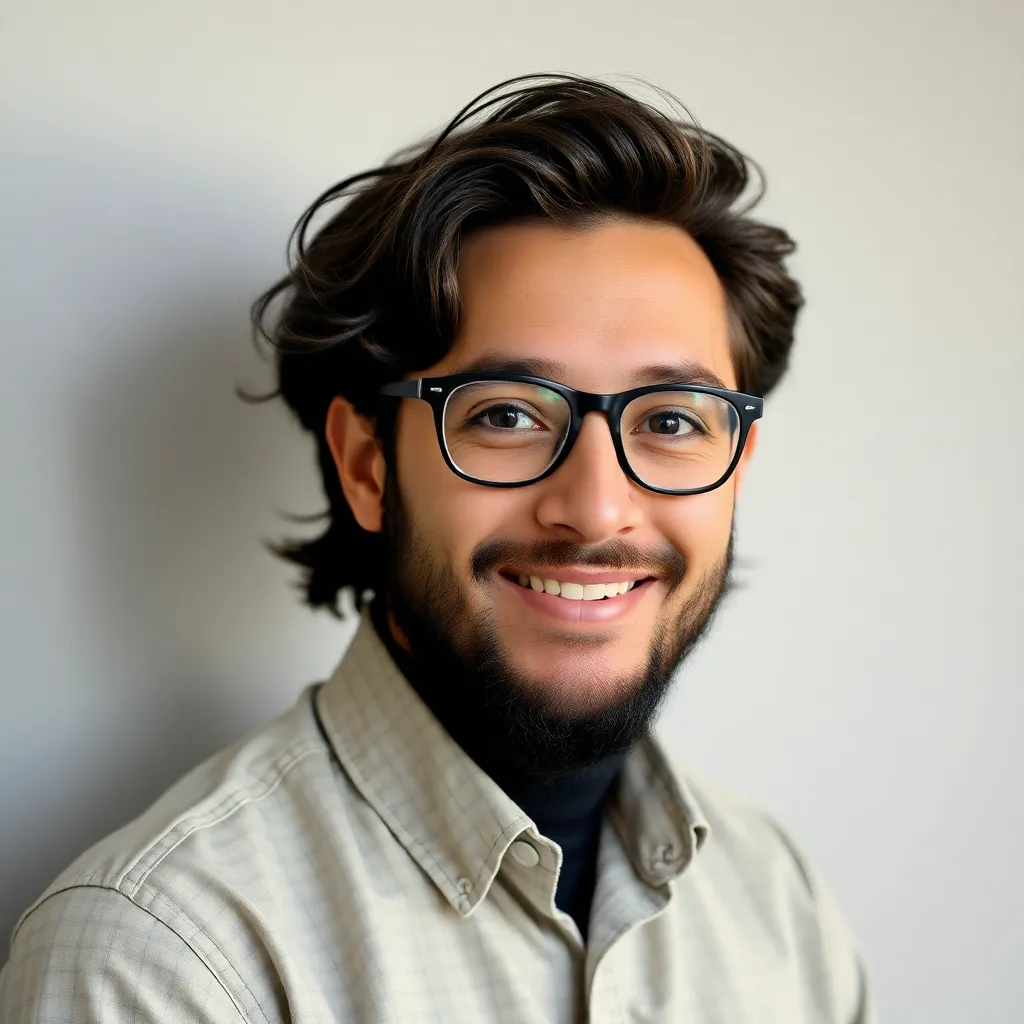
Muz Play
Apr 07, 2025 · 7 min read

Table of Contents
How to Describe Distribution in Statistics: A Comprehensive Guide
Understanding how to describe distribution in statistics is crucial for effectively analyzing and interpreting data. A distribution, in simple terms, describes how data points are spread out across a range of values. This guide will delve into various aspects of describing distributions, covering key concepts, methods, and practical applications. We'll explore both quantitative (numerical) and qualitative (categorical) data, equipping you with the tools to effectively communicate your findings.
Understanding the Fundamentals of Data Distribution
Before we delve into the specifics of describing distributions, let's clarify some foundational concepts. A data distribution summarizes how frequently different values appear in a dataset. Visualizing this is often the first step; common tools include histograms, frequency polygons, and box plots. These visual aids provide a quick understanding of the data's shape, center, and spread.
Key Characteristics of a Distribution
Several characteristics help us fully describe a distribution:
- Center (Central Tendency): This describes the typical or average value. Common measures include the mean, median, and mode.
- Mean: The average of all values. Sensitive to outliers.
- Median: The middle value when data is ordered. Robust to outliers.
- Mode: The most frequent value. Can be multiple modes (multimodal) or no mode.
- Spread (Dispersion or Variability): This describes how much the data values deviate from the center. Measures include range, interquartile range (IQR), variance, and standard deviation.
- Range: The difference between the maximum and minimum values. Highly sensitive to outliers.
- IQR: The difference between the 75th percentile (Q3) and the 25th percentile (Q1). More robust to outliers than the range.
- Variance: The average of the squared differences from the mean. Provides a measure of overall spread.
- Standard Deviation: The square root of the variance. Expressed in the same units as the data, making it easier to interpret.
- Shape: This describes the overall pattern of the distribution. Is it symmetric, skewed, or uniform? Are there any peaks (modes) or gaps? Common shapes include:
- Symmetric: Data is evenly distributed around the center. The mean and median are approximately equal.
- Skewed Right (Positively Skewed): The tail extends to the right, indicating a few high values. The mean is greater than the median.
- Skewed Left (Negatively Skewed): The tail extends to the left, indicating a few low values. The mean is less than the median.
- Uniform: All values have approximately equal frequency.
- Bimodal: Two distinct peaks are present.
- Multimodal: More than two distinct peaks are present.
Describing Distributions: Visual and Numerical Methods
The most effective way to describe a distribution often involves a combination of visual representations and numerical summaries.
Visual Methods: Creating Effective Data Visualizations
-
Histograms: These show the frequency distribution of a continuous variable. Data is grouped into bins (intervals), and the height of each bar represents the frequency of data points within that bin. Histograms are excellent for visualizing the shape and spread of the data.
-
Frequency Polygons: Similar to histograms, but instead of bars, points representing the frequency of each bin are connected by lines. This allows for a smoother representation of the distribution, particularly useful when comparing multiple distributions.
-
Box Plots (Box-and-Whisker Plots): These summarize the distribution using five key statistics: minimum, Q1 (25th percentile), median (Q2), Q3 (75th percentile), and maximum. The box represents the interquartile range (IQR), while whiskers extend to the minimum and maximum values (or sometimes to 1.5*IQR from Q1 and Q3 to highlight outliers). Box plots are excellent for comparing distributions across different groups or samples.
-
Stem-and-Leaf Plots: A less commonly used but still useful method, especially for smaller datasets. It displays the data values in a way that preserves the individual data points while also showing the distribution's shape.
Numerical Methods: Calculating Descriptive Statistics
Calculating descriptive statistics provides numerical summaries to complement the visual representations.
-
Measures of Central Tendency: Calculate the mean, median, and mode to identify the central location of the data. The choice of which measure is most appropriate depends on the data's shape and the presence of outliers. For symmetric distributions, the mean is a good representative. For skewed distributions, the median is often preferred as it is less influenced by outliers. The mode is useful for identifying the most frequent value(s).
-
Measures of Dispersion: Calculate the range, IQR, variance, and standard deviation to quantify the data's spread. The range provides a quick overview, but the IQR and standard deviation are generally more robust to outliers and provide a more comprehensive measure of variability. The standard deviation is particularly valuable when working with normally distributed data, as it provides a standardized way to interpret the spread relative to the mean.
Interpreting and Communicating Results
Once you have analyzed the distribution using both visual and numerical methods, the next crucial step is effectively communicating your findings.
-
Clear and Concise Language: Avoid technical jargon whenever possible. Explain your findings in a way that is easily understandable to your audience.
-
Context is Key: Always provide context for your analysis. What does the distribution tell us about the phenomenon being studied?
-
Highlight Key Findings: Focus on the most important aspects of the distribution, such as the shape, center, and spread. Are there any unusual features or outliers that warrant further investigation?
-
Use Appropriate Visualizations: Select the most appropriate visualization method based on the type of data and the audience. A histogram might be best for continuous data, while a box plot might be better for comparing distributions across different groups.
-
Relate Findings to the Research Question: Ensure that your description of the distribution directly addresses the research question or objective.
Advanced Concepts in Describing Distributions
For more advanced analyses, several additional concepts are vital:
-
Probability Distributions: These describe the probability of different outcomes in a random variable. Common examples include the normal distribution, binomial distribution, and Poisson distribution. Understanding these distributions is crucial for statistical inference and hypothesis testing.
-
Empirical Rule (68-95-99.7 Rule): This rule applies to normally distributed data, stating that approximately 68% of the data falls within one standard deviation of the mean, 95% within two standard deviations, and 99.7% within three standard deviations.
-
Z-Scores: These standardize data values by expressing them in terms of standard deviations from the mean. Z-scores allow for comparisons between different datasets with different units and scales.
-
Quantiles and Percentiles: These divide the data into equal parts. For example, quartiles divide the data into four equal parts, while percentiles divide it into 100 equal parts.
-
Kurtosis: This measures the "tailedness" of the probability distribution of a real-valued random variable. High kurtosis indicates heavy tails, while low kurtosis indicates light tails.
-
Skewness: A measure of the asymmetry of the probability distribution of a real-valued random variable about its mean.
Describing Distributions of Categorical Data
The methods for describing distributions differ slightly when dealing with categorical data (data that can be divided into groups or categories).
-
Frequency Tables: These show the count (frequency) of observations in each category.
-
Bar Charts: These visualize the frequencies of each category using bars, similar to histograms but for categorical data.
-
Pie Charts: These represent the proportion of each category using slices of a circle.
Conclusion: Mastering the Art of Describing Distributions
Describing distributions is a fundamental skill in statistics. By mastering the techniques outlined in this guide, you can effectively analyze, interpret, and communicate your data findings. Remember to use a combination of visual and numerical methods, choose appropriate measures of central tendency and dispersion based on your data's characteristics, and always present your findings in a clear and concise manner. The ability to clearly describe distributions is essential for making informed decisions based on data analysis and for effectively communicating those insights to others. Continuous practice and exploration of various datasets will solidify your understanding and refine your skills in this critical aspect of statistical analysis.
Latest Posts
Latest Posts
-
A Plane And A Line Can Intersect In A Point
Apr 09, 2025
-
Magnetic Field Of A Moving Charge
Apr 09, 2025
-
Describe The Relationship Between Temperature And Kinetic Energy
Apr 09, 2025
-
What Are The Formulas For Photosynthesis And Cellular Respiration
Apr 09, 2025
-
Equilibrium Constant Of Fescn2 Lab Answers
Apr 09, 2025
Related Post
Thank you for visiting our website which covers about How To Describe Distribution In Statistics . We hope the information provided has been useful to you. Feel free to contact us if you have any questions or need further assistance. See you next time and don't miss to bookmark.