How To Interpret Post Hoc Test
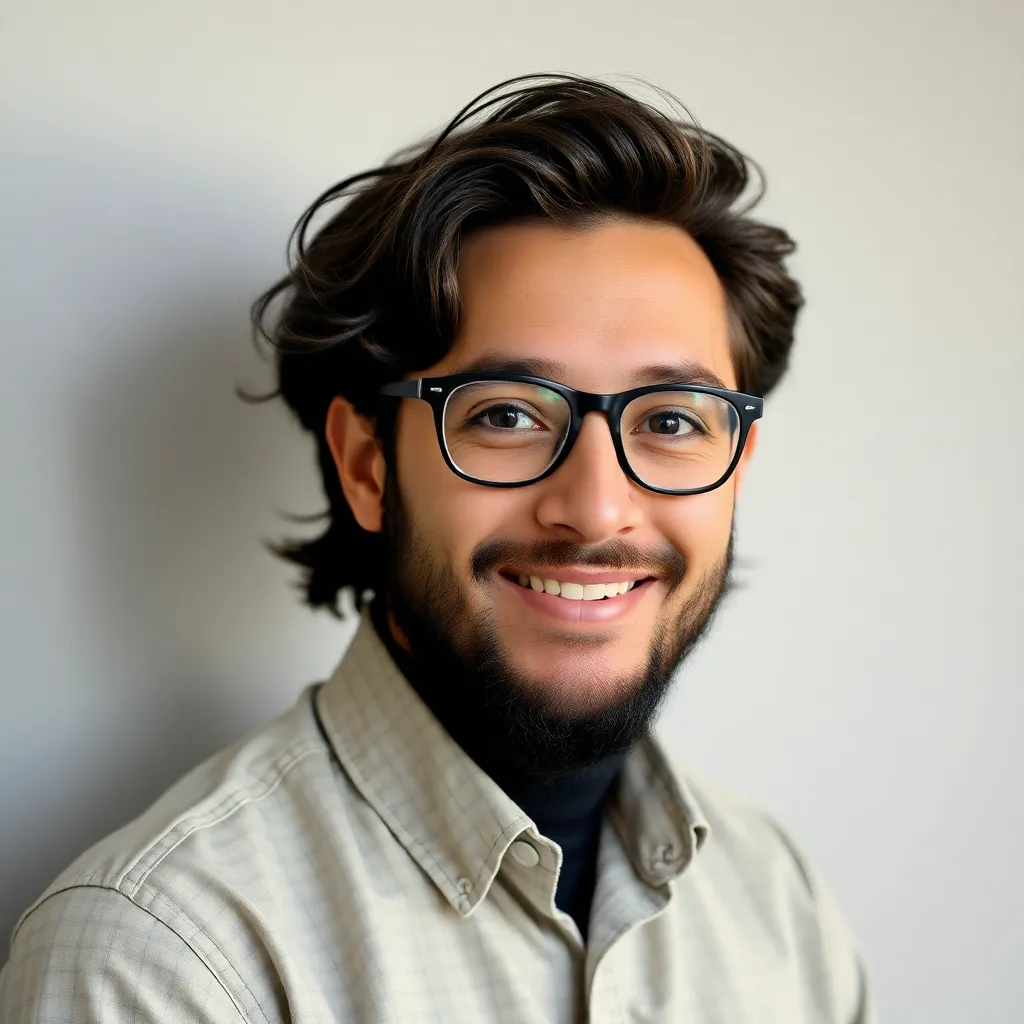
Muz Play
Apr 08, 2025 · 7 min read

Table of Contents
How to Interpret Post Hoc Tests: A Comprehensive Guide
Post hoc tests are crucial in statistical analysis when you've found a significant result in an ANOVA (Analysis of Variance) or similar test, indicating differences exist within your groups. However, ANOVA alone doesn't pinpoint where these differences lie. That's where post hoc tests step in, revealing which specific group means differ significantly from each other. This comprehensive guide will explore the interpretation of these vital tests, demystifying the process and empowering you to draw accurate conclusions from your data.
Understanding the Need for Post Hoc Tests
ANOVA tests whether there's an overall difference between the means of three or more groups. If the ANOVA is significant (p-value < α, your significance level, typically 0.05), it merely signifies that at least one group mean is statistically different from another. It doesn't identify which specific pairs of groups show this difference. Imagine comparing the effectiveness of four different medications. A significant ANOVA result would show that at least one medication is different from another. But which ones? This is where post hoc tests become invaluable.
Common Types of Post Hoc Tests
Several post hoc tests exist, each with its own strengths and weaknesses. The choice of the appropriate test depends on factors like the sample size, the distribution of your data, and whether your groups have equal variances. Here are some of the most frequently used:
1. Tukey's Honestly Significant Difference (HSD) Test
Tukey's HSD is a popular and robust method. It controls the family-wise error rate (FWER), meaning it minimizes the chance of making at least one Type I error (false positive) across all the comparisons. It's best suited for situations with equal group sizes and equal variances. The test uses a critical difference value, which is compared to the difference between each pair of group means. If the difference is greater than the critical difference, the means are considered significantly different.
Interpretation: Tukey's HSD typically presents results in a table, showing the difference between each pair of group means and whether the difference is statistically significant (often indicated by asterisks or letters). For example:
Group Pair | Mean Difference | p-value | Significant? |
---|---|---|---|
Group A - Group B | 5.2 | 0.012* | Yes |
Group A - Group C | 2.1 | 0.345 | No |
Group B - Group C | 3.1 | 0.187 | No |
- denotes statistical significance at a pre-determined alpha level (e.g. 0.05).
2. Bonferroni Correction
The Bonferroni correction is a simple yet conservative method. It adjusts the alpha level (significance level) to control the FWER. It divides the original alpha level by the number of comparisons made. While simple, it can be overly conservative, particularly with many comparisons, leading to a higher risk of Type II errors (false negatives). It's less powerful than Tukey's HSD but is relatively easy to understand and implement.
Interpretation: The Bonferroni-corrected p-values are compared to the adjusted alpha level. If a corrected p-value is less than the adjusted alpha, the difference between the group means is considered statistically significant.
3. Scheffé's Test
Scheffé's test is a very conservative method that controls the FWER for all possible comparisons, including complex contrasts (comparing more than just two group means at a time). It's highly robust but less powerful than other tests. It's suitable when you have unequal group sizes or want to explore more complex contrasts between group means beyond simple pairwise comparisons.
Interpretation: Similar to the Bonferroni correction, the p-values are compared against the adjusted alpha level. However, Scheffé's test often involves more complex calculations and might be less straightforward to interpret in standard statistical software output.
4. Games-Howell Test
Games-Howell is a powerful method particularly useful when your groups have unequal variances. It's a more robust alternative to Tukey's HSD in situations where the assumption of equal variances is violated.
Interpretation: Games-Howell, like other post hoc tests, usually provides a table with mean differences, p-values, and indicators of significance. Focus on the p-values to determine if the differences between group means are statistically significant.
5. Dunnett's Test
Dunnett's test is specifically designed for comparing several treatment groups to a single control group. This test is often used in experimental designs where you want to see if experimental treatments differ significantly from a baseline control condition.
Interpretation: Dunnett's test presents results showing whether each treatment group's mean is significantly different from the control group's mean.
Choosing the Right Post Hoc Test
The selection of the most appropriate post hoc test is critical for accurate interpretation. Consider the following factors:
- Equal Variances: If your groups have equal variances (tested using Levene's test or Bartlett's test), Tukey's HSD is generally preferred for its power and control of the FWER. If variances are unequal, Games-Howell is a robust option.
- Equal Sample Sizes: While many tests can handle unequal sample sizes, Tukey's HSD is most efficient with equal sample sizes.
- Number of Comparisons: With a large number of comparisons, Bonferroni and Scheffé's test can become overly conservative, leading to a reduced power to detect real differences.
- Specific Research Question: If your research focuses on comparing all groups to a specific control group, Dunnett's test is more efficient than the other tests.
Interpreting Post Hoc Test Results: A Step-by-Step Guide
-
Check the ANOVA Results: Before interpreting post hoc tests, ensure your ANOVA result is statistically significant. If the ANOVA is not significant, there's no need to perform post hoc tests.
-
Examine the Post Hoc Test Output: Most statistical software packages (like SPSS, R, SAS) present the results in a table format, showing pairwise comparisons of group means, the differences between means, and associated p-values (or adjusted p-values, depending on the test).
-
Identify Significant Differences: Compare the p-values to your alpha level (usually 0.05). If a p-value is less than your alpha level, the difference between the two groups being compared is statistically significant.
-
Consider Effect Sizes: While statistical significance is important, also consider the effect size. A statistically significant difference might be small and practically insignificant. Effect size measures (like Cohen's d) can help quantify the magnitude of the difference between groups.
-
Visualize the Results: Create graphs (e.g., bar charts with error bars) to visually represent the group means and significant differences. This enhances the clarity and understanding of your findings.
-
Write Clear Interpretations: In your report, clearly state which groups differ significantly from each other and provide a concise summary of your findings, emphasizing the practical implications of your results. Avoid over-interpreting non-significant results.
Common Pitfalls to Avoid
- Ignoring Assumptions: Ensure you meet the assumptions of your chosen post hoc test (e.g., normality, homogeneity of variances). Violating these assumptions can lead to inaccurate conclusions.
- Focusing Only on p-values: Do not solely rely on p-values. Consider effect sizes and the practical significance of your results.
- Performing Post Hoc Tests Without a Significant ANOVA: It's inappropriate to perform post hoc tests if your ANOVA is not significant.
- Misinterpreting Non-significant Results: A non-significant result doesn't necessarily mean there's no difference between groups; it simply means the difference wasn't large enough to be statistically significant with your sample size and chosen test.
- Ignoring Multiple Comparisons: Failure to address multiple comparisons (performing multiple post hoc tests without adjusting alpha) can inflate the Type I error rate.
Conclusion
Post hoc tests are an essential part of statistical analysis following a significant ANOVA. Selecting and interpreting the appropriate post hoc test is crucial for drawing accurate and meaningful conclusions from your data. Careful consideration of your data's characteristics, the choice of test, and the interpretation of results will lead to more robust and reliable findings, improving the overall quality of your research. Always remember that statistical significance should be considered alongside effect sizes and the practical implications of your results to provide a comprehensive understanding of your research question.
Latest Posts
Latest Posts
-
For A Certain Substitution Reaction The Rate Of Substitution
Apr 17, 2025
-
Is Boiling Point Chemical Or Physical
Apr 17, 2025
-
Variables Can Take On Any Value In Some Interval
Apr 17, 2025
-
What Happens When Rocks Oxidize Near Earths Surface
Apr 17, 2025
-
Label The Following Fatty Acids As Saturated Or Unsaturated
Apr 17, 2025
Related Post
Thank you for visiting our website which covers about How To Interpret Post Hoc Test . We hope the information provided has been useful to you. Feel free to contact us if you have any questions or need further assistance. See you next time and don't miss to bookmark.