Hypothesis Test For A Population Proportion
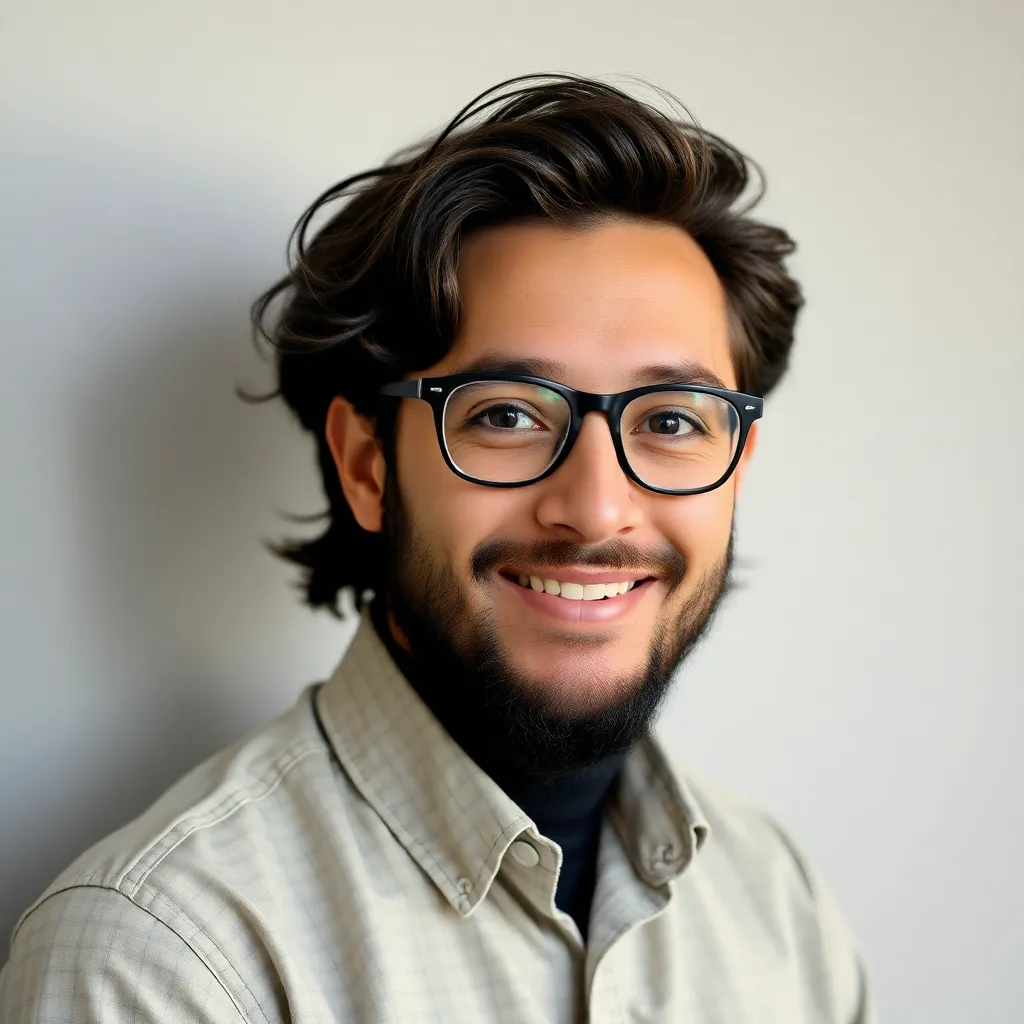
Muz Play
Mar 14, 2025 · 6 min read

Table of Contents
Hypothesis Testing for a Population Proportion: A Comprehensive Guide
Hypothesis testing is a cornerstone of statistical inference, allowing us to draw conclusions about a population based on a sample of data. One common type of hypothesis test involves examining a population proportion – the percentage of individuals in a population possessing a specific characteristic. This guide will delve into the intricacies of hypothesis testing for a population proportion, providing a comprehensive understanding of the process, its assumptions, and its applications.
Understanding Population Proportions
Before diving into hypothesis testing, let's clarify what a population proportion represents. Imagine you're interested in the proportion of voters who support a particular candidate. The population is all eligible voters, and the population proportion (often denoted as p) is the true, but usually unknown, percentage who support that candidate. We rarely have access to data from the entire population; instead, we collect data from a sample and use it to make inferences about the population proportion.
The Hypothesis Testing Framework
Hypothesis testing for a population proportion follows a structured process:
1. State the Hypotheses
We formulate two competing hypotheses:
-
Null Hypothesis (H₀): This is the statement we aim to disprove. It typically asserts that the population proportion is equal to a specific value (often a status quo or a previously established value). For example, H₀: p = 0.5 (50% of voters support the candidate).
-
Alternative Hypothesis (H₁ or Hₐ): This is the statement we are trying to find evidence for. It can be one-sided (directional) or two-sided (non-directional).
- One-sided (directional): H₁: p > 0.5 (more than 50% support the candidate) or H₁: p < 0.5 (less than 50% support the candidate).
- Two-sided (non-directional): H₁: p ≠ 0.5 (the proportion is different from 50%).
The choice between a one-sided and two-sided alternative depends on the research question. A one-sided test is appropriate when we have a specific direction in mind (e.g., we suspect the proportion is greater than a certain value). A two-sided test is more general and appropriate when we are simply interested in whether the proportion is different from a specific value.
2. Determine the Significance Level (α)
The significance level (alpha) represents the probability of rejecting the null hypothesis when it is actually true (Type I error). Common significance levels are 0.05 (5%) and 0.01 (1%). A lower significance level reduces the risk of a Type I error but increases the chance of failing to reject a false null hypothesis (Type II error).
3. Collect Data and Calculate the Sample Proportion
A random sample is drawn from the population, and the sample proportion (often denoted as p̂) is calculated. This is the proportion of individuals in the sample who possess the characteristic of interest. For example, if we survey 100 voters and 60 support the candidate, then p̂ = 60/100 = 0.6.
4. Calculate the Test Statistic
The test statistic measures how far the sample proportion deviates from the null hypothesis value. For testing a population proportion, we use the z-statistic:
z = (p̂ - p₀) / √(p₀(1-p₀) / n)
where:
- p̂ is the sample proportion
- p₀ is the population proportion under the null hypothesis
- n is the sample size
5. Determine the p-value
The p-value is the probability of observing a sample proportion as extreme as (or more extreme than) the one obtained, assuming the null hypothesis is true. A small p-value suggests that the observed result is unlikely under the null hypothesis, providing evidence against it.
We find the p-value using the standard normal distribution (z-distribution). For a two-sided test, we double the probability in one tail. Statistical software or z-tables can be used to determine the p-value.
6. Make a Decision
We compare the p-value to the significance level (α).
- If p-value ≤ α: Reject the null hypothesis. There is sufficient evidence to support the alternative hypothesis.
- If p-value > α: Fail to reject the null hypothesis. There is not enough evidence to support the alternative hypothesis.
Important Note: Failing to reject the null hypothesis does not mean we accept it as true. It simply means we don't have enough evidence to reject it based on the available data.
Assumptions of the Hypothesis Test
The validity of the hypothesis test relies on certain assumptions:
- Random Sampling: The sample should be randomly selected from the population to ensure it's representative.
- Independence: Observations in the sample should be independent of each other. This is often satisfied if the sample size is less than 10% of the population size.
- Sample Size: The sample size should be large enough to ensure the sampling distribution of the sample proportion is approximately normal. A common rule of thumb is that both np₀ and n(1-p₀) should be greater than or equal to 10. This ensures the central limit theorem applies, guaranteeing a normal distribution for the sample proportion.
Example: Testing Voter Support
Let's illustrate the process with an example. Suppose we want to test whether more than 50% of voters support a candidate.
-
Hypotheses:
- H₀: p = 0.5
- H₁: p > 0.5 (one-sided test)
-
Significance Level: α = 0.05
-
Data: We survey 400 voters, and 230 support the candidate. p̂ = 230/400 = 0.575
-
Test Statistic: z = (0.575 - 0.5) / √(0.5(1-0.5) / 400) ≈ 2.92
-
p-value: Using a z-table or statistical software, the p-value for z = 2.92 (one-tailed) is approximately 0.0018.
-
Decision: Since the p-value (0.0018) is less than α (0.05), we reject the null hypothesis. There is sufficient evidence to conclude that more than 50% of voters support the candidate.
Interpreting Results and Potential Errors
The conclusion of a hypothesis test should be carefully interpreted. Remember the potential for errors:
-
Type I Error: Rejecting the null hypothesis when it is actually true (false positive). The probability of this error is equal to the significance level (α).
-
Type II Error: Failing to reject the null hypothesis when it is actually false (false negative). The probability of this error is denoted by β and depends on factors like the true population proportion, the sample size, and the significance level. The power of a test (1-β) represents the probability of correctly rejecting a false null hypothesis.
Power Analysis and Sample Size Determination
Before conducting a hypothesis test, it's crucial to consider the power of the test. A low-power test might fail to detect a true difference, leading to a Type II error. Power analysis helps determine the required sample size to achieve a desired level of power (e.g., 80% or 90%). Several factors influence power, including:
- Significance level (α): Lowering α reduces power.
- Effect size: A larger difference between the null hypothesis value and the true population proportion increases power.
- Sample size (n): Increasing the sample size increases power.
Conclusion
Hypothesis testing for a population proportion is a powerful tool for making inferences about population characteristics based on sample data. Understanding the underlying principles, assumptions, potential errors, and the importance of power analysis is crucial for conducting valid and reliable statistical analyses. Remember that careful interpretation of results, acknowledging limitations, and considering the practical implications are essential components of successful hypothesis testing. This comprehensive guide provides a strong foundation for applying these techniques in various fields requiring statistical inference. Further exploration into advanced statistical concepts and software packages can enhance your expertise in this area.
Latest Posts
Latest Posts
-
How To Solve A Function With A Square Root
May 09, 2025
-
Which Three Elements Have The Most Similar Chemical Properties
May 09, 2025
-
What Is The Optimal Ph For Gastric Protease
May 09, 2025
-
What Is A Solution Set Of An Inequality
May 09, 2025
-
Basic Building Blocks Of The Nervous System
May 09, 2025
Related Post
Thank you for visiting our website which covers about Hypothesis Test For A Population Proportion . We hope the information provided has been useful to you. Feel free to contact us if you have any questions or need further assistance. See you next time and don't miss to bookmark.