If A Distribution Is Skewed To The Left
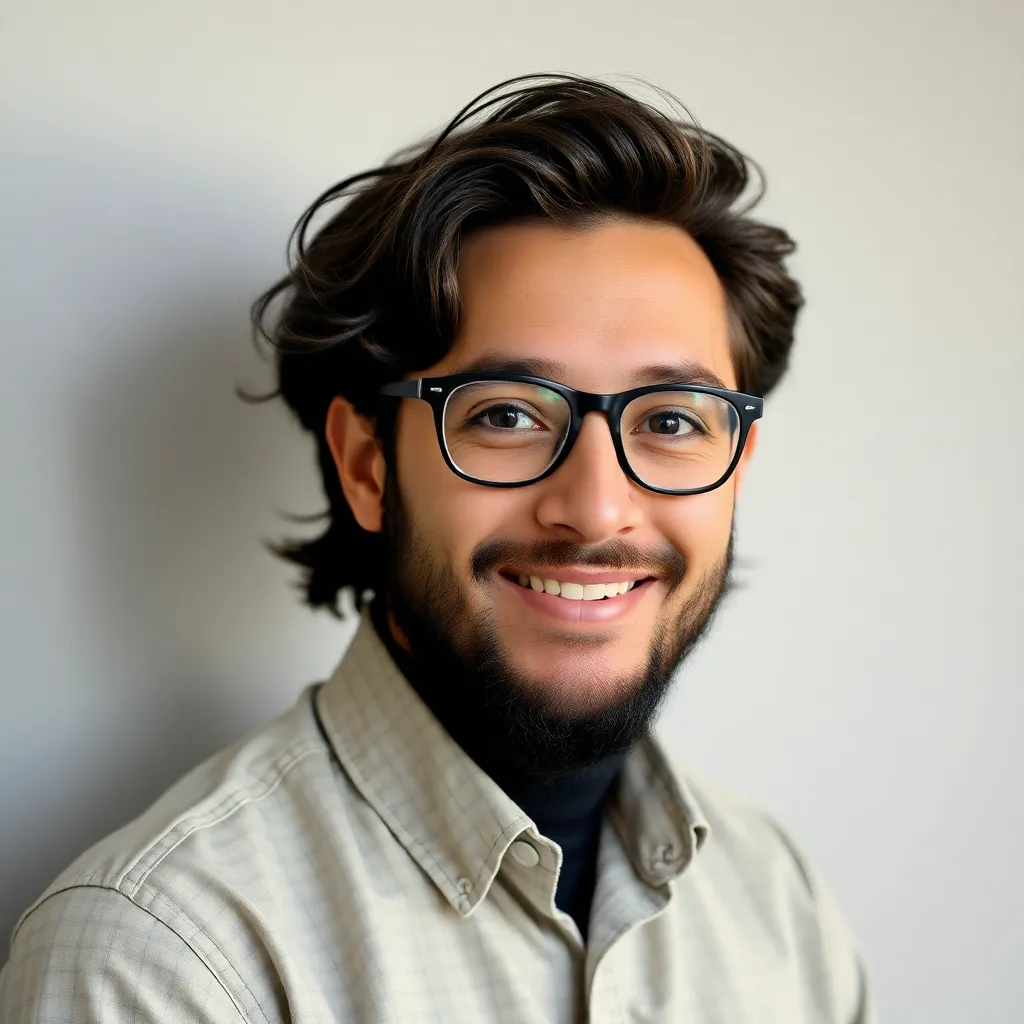
Muz Play
Apr 19, 2025 · 6 min read

Table of Contents
If a Distribution is Skewed to the Left: A Comprehensive Guide
Understanding data distributions is crucial in statistics. A key aspect of describing a distribution is its skewness – a measure of its asymmetry. This article delves deep into left-skewed distributions, also known as negatively skewed distributions, exploring their characteristics, causes, implications, and how to handle them in various statistical analyses.
What is a Left-Skewed Distribution?
A left-skewed distribution, or negatively skewed distribution, is one where the tail on the left-hand side is longer or fatter than the tail on the right-hand side. The majority of the data points cluster towards the higher end of the scale, with a few outliers stretching far to the left. Visually, the distribution's peak (mode) is shifted towards the right, the mean is pulled to the left of the median, and the median is to the left of the mode. This creates a tail that extends to the left, hence the term "left-skewed."
Key Characteristics of a Left-Skewed Distribution:
- Mean < Median < Mode: This is the most distinguishing feature. The mean is pulled downwards by the extreme values in the left tail.
- Asymmetrical: The distribution is not symmetrical around its mean.
- Long Left Tail: A significant number of data points are located far below the mean.
- Skewness Coefficient: A negative value for the skewness coefficient indicates a left-skewed distribution. However, the exact value varies depending on the chosen method of calculation (e.g., Pearson's moment coefficient of skewness, Bowley's coefficient).
Visual Representations of Left Skewness
Understanding the visual representation is key. Imagine a bell curve – a perfectly symmetrical distribution. Now, imagine stretching the left side of that curve, extending the tail far to the left. That's a left-skewed distribution.
Examples of Visualizations:
- Histograms: A histogram of a left-skewed distribution will show a concentration of bars on the right side, with a long tail extending to the left. The bars gradually decrease in height as you move leftward.
- Box Plots: A box plot will reveal the skewness through the position of the median relative to the quartiles and the length of the whiskers extending to the left. A longer left whisker suggests left skewness.
- Probability Density Functions (PDFs): These curves provide a more continuous representation, visually highlighting the asymmetry and the long left tail.
Causes of Left-Skewed Distributions
Several factors can contribute to left skewness in your data. Understanding these underlying causes can be crucial for interpreting the results and drawing meaningful conclusions.
Common Causes:
- Natural Limits: When a variable has a lower bound (e.g., zero), and the majority of values cluster close to that lower bound, it can lead to a left-skewed distribution. Examples include income (many people earn modest amounts while a few earn extremely high salaries), exam scores (a large number of students score around the average or below, with a few high scores), and age at death.
- Censoring: Data might be censored, meaning certain values are not observable. For example, measuring the time until an event occurs (e.g., equipment failure) might result in left skewness if the observation period is limited and some events happen after the observation ends.
- Measurement Errors: Systematic errors in measuring a variable toward the lower end of the range can create a left skew.
- Sampling Bias: If the sampling method systematically under-represents the higher values or over-represents the lower values, it can lead to a left-skewed sample.
Implications of Left Skewness
The presence of left skewness influences various statistical analyses and interpretations. Ignoring it can lead to inaccurate conclusions.
Implications for Statistical Analysis:
- Mean is not a representative measure of central tendency: Because the mean is heavily influenced by outliers in the left tail, the median becomes a more robust measure of central tendency in left-skewed distributions.
- Effect on Standard Deviation: The standard deviation, a measure of dispersion, can be inflated by extreme values in the left tail.
- Statistical Tests: Certain statistical tests assume normality (symmetrical distribution). If the data is severely left-skewed, these tests might not be valid, leading to inaccurate results. Transformations may be necessary to address this.
- Interpretation of Results: The presence of left skewness needs to be considered when interpreting statistical results. For instance, a high average score on an exam but a left-skewed distribution suggests the difficulty of the exam and a clustering of low scores.
Handling Left-Skewed Data
Several techniques can be used to address left skewness, depending on the severity and the goal of the analysis.
Methods for Handling Left Skewness:
- Data Transformation: This involves applying mathematical functions to the data to make it more symmetrical. Common transformations include:
- Log Transformation: Taking the natural logarithm of the data often helps reduce left skewness. It is particularly useful when dealing with positively valued data.
- Square Root Transformation: Applying the square root transformation can also reduce skewness.
- Box-Cox Transformation: A more flexible transformation family that includes logarithmic and square root as special cases.
- Non-parametric Methods: These methods do not assume a specific distribution shape and are less sensitive to outliers. Examples include non-parametric tests (e.g., Wilcoxon rank-sum test, Kruskal-Wallis test) and robust statistical measures (e.g., median, interquartile range).
- Winsorizing or Trimming: Winsorizing replaces extreme values with less extreme values, while trimming removes extreme values entirely. This reduces the influence of outliers.
- Robust Regression: Instead of ordinary least squares regression, robust regression techniques are less sensitive to outliers.
Examples of Left-Skewed Distributions in Real-World Applications
Numerous real-world phenomena exhibit left-skewed distributions. Recognizing these examples enhances the practical application of understanding left skewness.
Real-World Applications:
- Income Distribution: In most countries, the income distribution is typically left-skewed, with a large number of people earning lower incomes and a smaller number earning very high incomes.
- Test Scores: Exam scores often follow a left-skewed pattern, with many students achieving average or below-average scores and a few students scoring exceptionally high.
- Age at Death: The age at death distribution is usually left-skewed, as most people die at older ages, with fewer dying at much younger ages.
- Wealth Distribution: Similar to income, wealth distribution usually presents a left-skewed nature, with a vast majority possessing relatively modest wealth and a small number holding significant assets.
- Time to Failure (Reliability Analysis): In certain reliability studies, the distribution of time until failure of a system or component can be left-skewed, especially if the system has a strong initial period of failure.
Advanced Considerations
Further exploration into the nuances of left-skewed distributions can deepen understanding and refine analytical approaches.
Advanced Topics:
- Mixture Models: These models allow for the representation of a data set as a mixture of several different distributions, possibly including a component with a left skew.
- Copulas: For multivariate data exhibiting left skewness, copulas can model the dependence structure without specifying the individual marginal distributions.
- Simulation Studies: Simulations can help assess the impact of left skewness on various statistical inferences and inform decisions regarding data transformation or robust methods.
Conclusion
Left-skewed distributions are common occurrences in various fields. Understanding their characteristics, causes, and implications is critical for accurate data analysis and interpretation. By employing appropriate techniques for handling skewness, researchers and analysts can draw valid conclusions and make informed decisions. Remember that choosing the appropriate method depends heavily on the context, the severity of the skewness, and the specific goals of the analysis. Always visually inspect your data and consider the underlying processes generating it to choose the best approach.
Latest Posts
Latest Posts
-
Does Forming A Bond Require Energy
Apr 19, 2025
-
Why Does Volume Increase When Temperature Increases
Apr 19, 2025
-
Combination Of Chemical Symbols And Numbers To Represent A Substance
Apr 19, 2025
-
The Chemical Level Of An Organization Is
Apr 19, 2025
-
The Combination Of Plasma And Formed Elements Is Called
Apr 19, 2025
Related Post
Thank you for visiting our website which covers about If A Distribution Is Skewed To The Left . We hope the information provided has been useful to you. Feel free to contact us if you have any questions or need further assistance. See you next time and don't miss to bookmark.