If Median Is Greater Than Mean
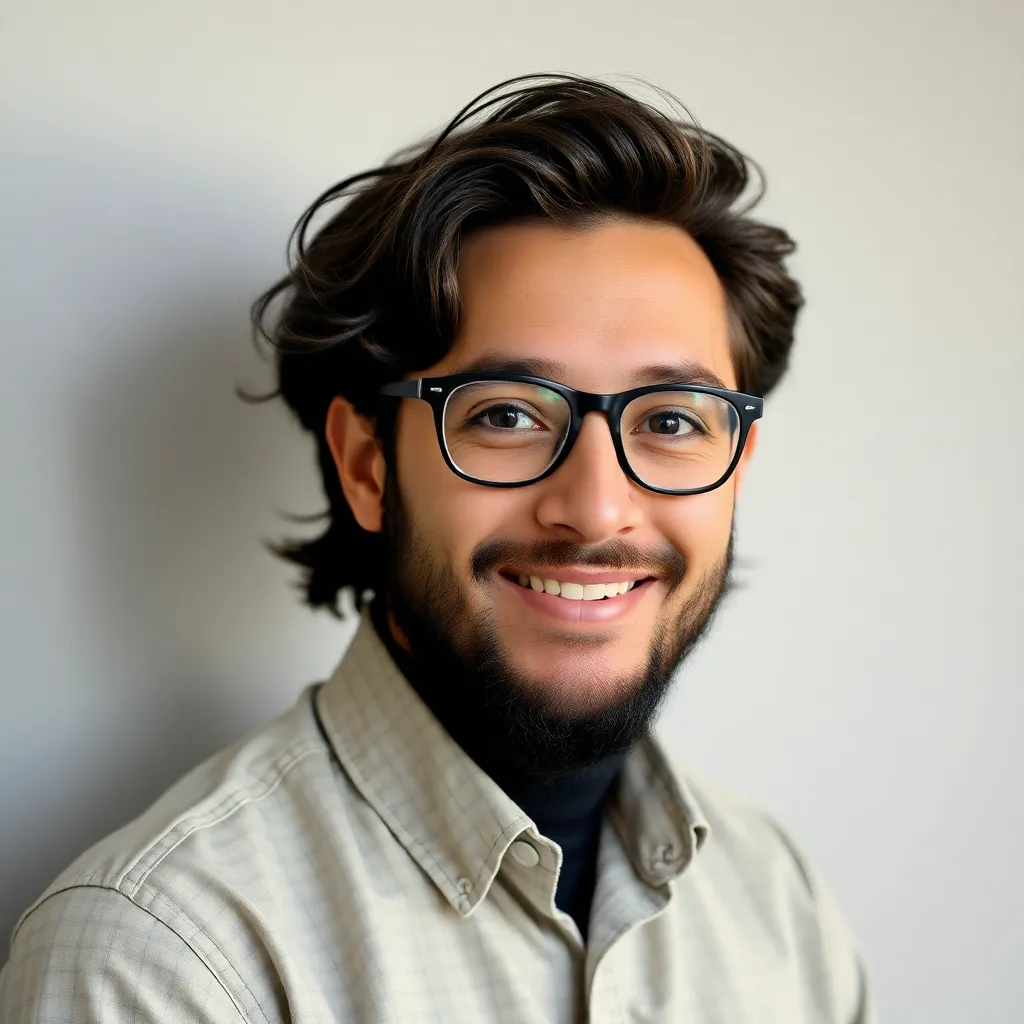
Muz Play
May 11, 2025 · 6 min read
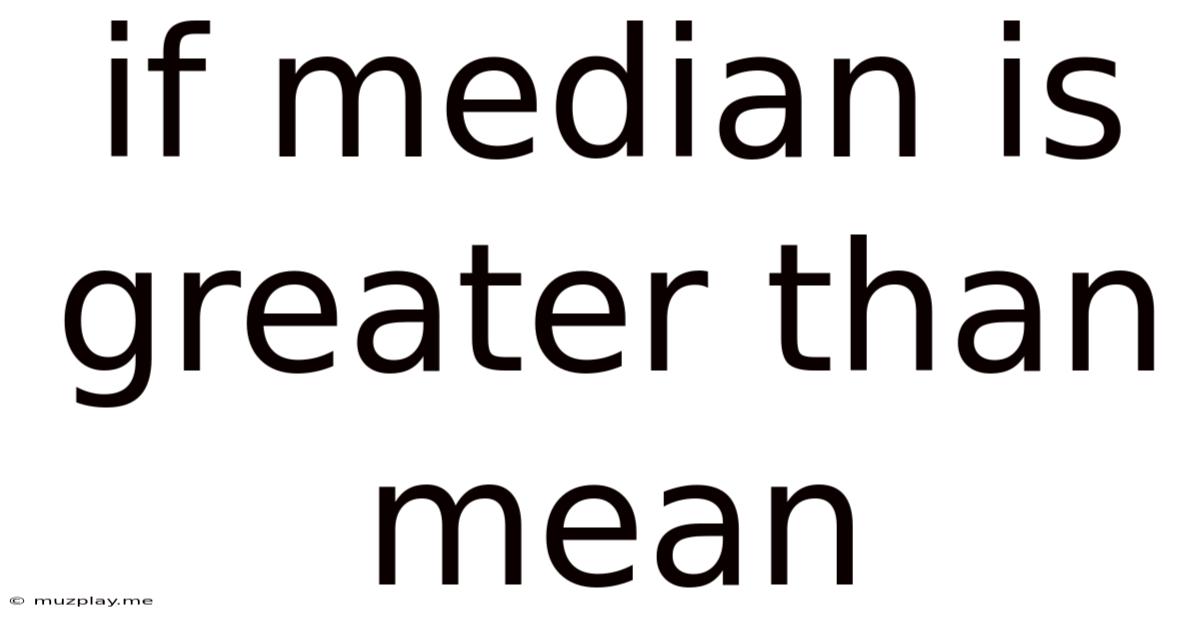
Table of Contents
If the Median is Greater Than the Mean: Understanding Skewed Distributions
The relationship between the mean and median of a dataset provides valuable insights into the data's underlying distribution. While the mean (average) and median (middle value) often provide similar information, discrepancies can reveal important characteristics, particularly when the median is greater than the mean. This situation indicates a left-skewed distribution, also known as a negatively skewed distribution. Understanding this type of skew is crucial for accurate data interpretation and informed decision-making in various fields, from finance and economics to healthcare and social sciences.
What Does it Mean When the Median Exceeds the Mean?
When the median is larger than the mean, it signifies that the data is skewed to the left. This means there's a concentration of data points towards the higher end of the distribution, with a long tail extending towards the lower values. This tail, containing relatively few but exceptionally low data points, pulls the mean downward, causing it to be smaller than the median, which is less sensitive to extreme values.
Imagine a dataset representing the incomes of individuals in a particular community. If the median income is significantly higher than the mean income, it suggests the presence of a few individuals with extremely low incomes. These low incomes, although few in number, disproportionately affect the mean, dragging it down. The median, however, remains relatively unaffected because it only considers the middle value. This scenario perfectly illustrates a left-skewed distribution.
Visualizing Left Skewness
Understanding left skewness is greatly aided by visualizing it. A typical histogram or box plot of a left-skewed distribution shows a longer tail on the left side. The peak of the distribution will be towards the higher values, with a gradual tapering off towards the lower values. The mean will be positioned to the left of the median, reflecting the influence of the lower outliers.
Key Visual Characteristics of a Left-Skewed Distribution:
- Longer left tail: A significant portion of data points are clustered towards the higher end of the scale, with fewer data points spread out over a wider range on the lower end.
- Median greater than mean: This is the defining characteristic of a left-skewed distribution.
- Skewness measure: Statistical measures of skewness will typically yield a negative value, further confirming the left skew.
Causes of Left-Skewed Distributions
Various factors can contribute to the creation of a left-skewed distribution. Understanding these factors is crucial for interpreting the data meaningfully. Some common causes include:
1. Censored Data or Lower Bounds:
Many datasets have inherent lower bounds. For instance, income cannot be negative, test scores cannot be below zero, and ages are always positive. The presence of a hard lower bound can artificially create a left-skewed appearance if a considerable portion of the data is clustered near the upper end of the range.
2. Outliers:
Extreme low values, which are often outliers, can significantly impact the mean, causing it to be lower than the median. These outliers can be genuine data points or the result of errors in data collection or measurement. Identifying and handling outliers appropriately is essential for accurate analysis.
3. Natural Phenomena:
Some natural phenomena exhibit inherent left skewness. For example, the distribution of lifespan might be left-skewed due to factors like infant mortality, where a small percentage of individuals die at a very young age, affecting the overall average lifespan.
4. Grading Systems:
In certain grading systems, a large majority of students may score highly, resulting in a left-skewed distribution of grades. A few students scoring exceptionally low can disproportionately affect the mean grade.
Implications of Left Skewness
The presence of left skewness has important implications for data analysis and interpretation. It's crucial to consider the following:
1. Misleading Averages:
The mean can be misleading in left-skewed distributions as it's heavily influenced by the extreme low values. The median provides a more robust measure of central tendency in such cases, offering a better representation of the typical value.
2. Data Interpretation:
Left skewness suggests a concentration of data points at the higher end of the scale. Understanding this concentration is vital for making accurate inferences and informed decisions. For example, in assessing the effectiveness of a medical treatment, a left-skewed distribution of patient recovery times might indicate a highly effective treatment with only a few patients experiencing prolonged recovery.
3. Statistical Modeling:
Choosing appropriate statistical models is essential when dealing with left-skewed data. Standard statistical tests often assume a normal distribution; violating this assumption can lead to inaccurate results. Transformations such as logarithmic transformations can be applied to normalize the data and improve the validity of statistical analysis.
4. Real-World Applications:
Left-skewness appears in many real-world applications. Understanding its implications is vital across diverse fields. Examples include:
- Finance: Analyzing investment returns, where a few extremely low returns can heavily influence the average return.
- Healthcare: Studying patient recovery times or survival rates, where a small number of patients experiencing adverse outcomes can affect the overall average.
- Environmental Science: Examining pollution levels, where a few extreme pollution events can distort the average level.
Handling Left-Skewed Data
Handling left-skewed data effectively involves several strategies:
1. Data Transformation:
Transforming the data using mathematical functions, like logarithmic or square root transformations, can often normalize the distribution, making it more symmetrical and amenable to standard statistical methods.
2. Robust Statistical Measures:
Using robust statistical measures like the median and interquartile range, which are less sensitive to outliers, provides a more accurate representation of the central tendency and variability in the data.
3. Non-parametric Methods:
Non-parametric statistical methods, which do not assume a specific data distribution, are suitable for analyzing left-skewed data without the need for transformations.
4. Outlier Treatment:
Investigate and address outliers appropriately. Outliers may be genuine data points or errors. If they are errors, correct them. If they are genuine, consider whether they should be included in the analysis or if a different analytical approach is more appropriate.
Conclusion: The Significance of Understanding Skewness
The relationship between the mean and median, particularly when the median is greater than the mean, is a powerful indicator of left skewness. Understanding this type of distribution is crucial for accurate data interpretation and informed decision-making across various fields. By recognizing the causes and implications of left skewness, and by employing appropriate handling techniques, data analysts can extract valuable insights and avoid misleading conclusions. This knowledge empowers informed choices, robust analyses, and a more nuanced understanding of the underlying data. Remember always to consider the context of the data and the potential impact of outliers when interpreting a left-skewed distribution. Careful consideration of these factors ensures the reliability and validity of your analysis, leading to more accurate and useful conclusions.
Latest Posts
Latest Posts
-
Excretion Is Best Described As The Removal Of
May 12, 2025
-
What Is The Number Of Protons In Silicon
May 12, 2025
-
Crossing Over Begins To Occur During
May 12, 2025
-
Grignard Synthesis Of Triphenylmethanol Lab Report
May 12, 2025
-
Which Describes An Interaction Within The Musculoskeletal System
May 12, 2025
Related Post
Thank you for visiting our website which covers about If Median Is Greater Than Mean . We hope the information provided has been useful to you. Feel free to contact us if you have any questions or need further assistance. See you next time and don't miss to bookmark.