In Order To Generate Findings About Causation
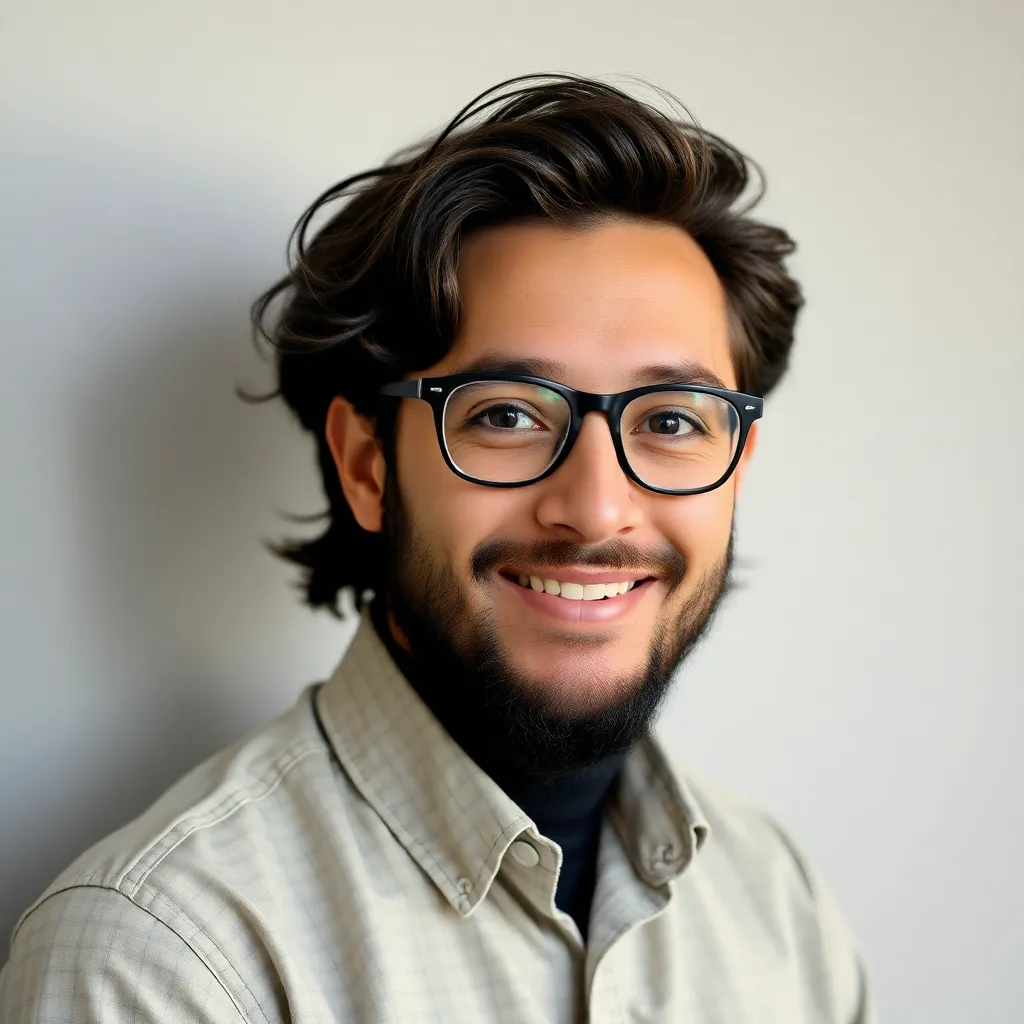
Muz Play
May 09, 2025 · 7 min read
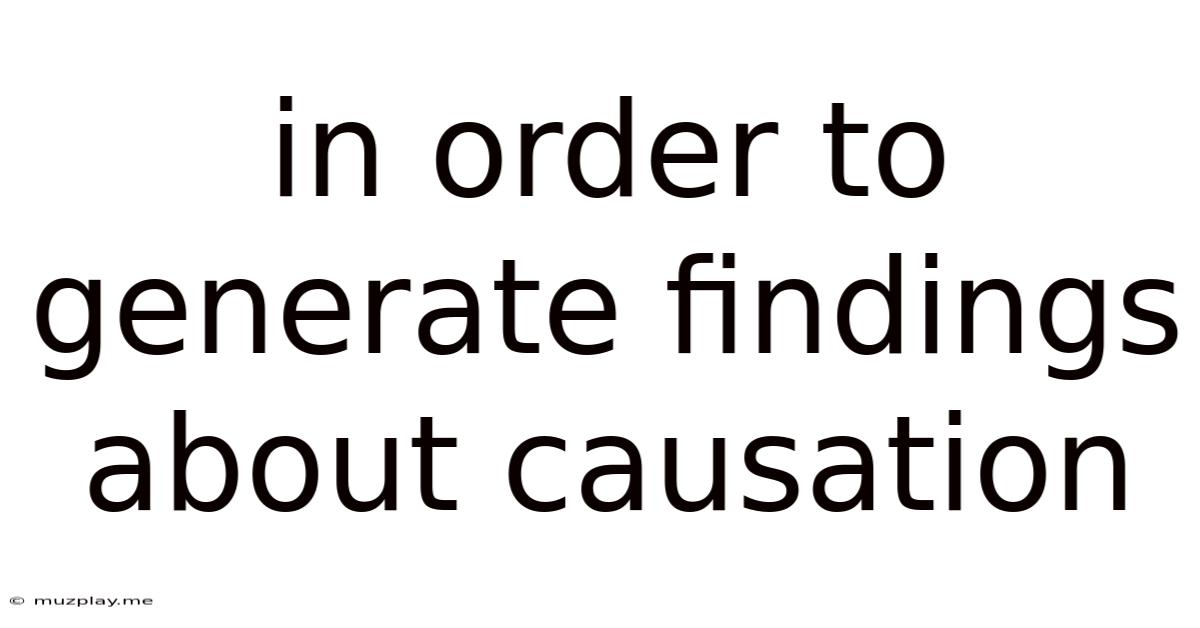
Table of Contents
Generating Findings About Causation: A Comprehensive Guide
Establishing causation is a cornerstone of scientific inquiry and many other fields. It goes beyond simple correlation; it requires demonstrating that one event, X, causes another event, Y. This isn't always straightforward, and numerous methods and considerations are crucial for generating robust findings about causation. This article delves into the intricacies of causal inference, exploring various approaches and highlighting the challenges involved.
Understanding the Difference Between Correlation and Causation
Before diving into methods for establishing causation, it's crucial to understand the fundamental difference between correlation and causation. Correlation simply indicates a statistical relationship between two variables – they tend to change together. However, correlation does not imply causation. Two variables might be correlated due to a third, unobserved variable (a confounding variable), or the relationship might be purely coincidental.
Causation, on the other hand, implies a direct causal link: X directly influences Y. To establish causation, we need to demonstrate not only that X and Y are associated but also that the change in X precedes the change in Y and that this relationship isn't explained by other factors.
Examples Illustrating the Difference
-
Correlation without Causation: Ice cream sales and drowning incidents are positively correlated. However, eating ice cream doesn't cause drowning. Both are linked to a third variable: hot weather. Increased temperatures lead to increased ice cream consumption and more people swimming, thus increasing the risk of drowning.
-
Causation: Regular exercise is causally linked to improved cardiovascular health. Studies have demonstrated that engaging in physical activity directly impacts the heart and circulatory system, leading to positive health outcomes. This isn't simply a correlation; the relationship is supported by biological mechanisms.
Key Methods for Establishing Causation
Several methods are employed to generate findings about causation, each with its strengths and limitations.
1. Randomized Controlled Trials (RCTs)
RCTs are considered the gold standard for establishing causal relationships. In an RCT, participants are randomly assigned to either an experimental group (receiving the treatment or intervention) or a control group (receiving a placebo or standard treatment). Randomization minimizes the influence of confounding variables, ensuring that the observed differences between groups are likely due to the intervention.
Strengths: RCTs offer strong internal validity, meaning the observed effects are likely due to the intervention being studied.
Limitations: RCTs can be expensive, time-consuming, and ethically challenging in some cases. They may also have limited external validity (generalizability to other populations or settings). Furthermore, certain interventions, such as exposure to environmental toxins, cannot be ethically randomized.
2. Observational Studies
When RCTs are not feasible or ethical, observational studies can provide valuable insights into causal relationships. These studies observe and analyze existing data without manipulating variables. Several types of observational studies exist:
-
Cohort Studies: Follow a group of individuals over time to observe the development of a particular outcome.
-
Case-Control Studies: Compare individuals with a particular outcome (cases) to those without the outcome (controls) to identify potential risk factors.
-
Cross-sectional Studies: Examine the relationship between variables at a single point in time.
Strengths: Observational studies can be less expensive and time-consuming than RCTs and are often more feasible for studying long-term effects or rare outcomes.
Limitations: Observational studies are susceptible to confounding variables, making it difficult to establish clear causal links. Establishing causality requires careful consideration of potential confounders and the use of statistical techniques to adjust for their influence.
3. Instrumental Variables
Instrumental variables (IV) are used to address endogeneity, a situation where the independent variable is correlated with the error term in a regression model. An IV is a variable that influences the independent variable but does not directly affect the dependent variable except through its influence on the independent variable. It essentially acts as a proxy for the independent variable, allowing researchers to isolate its causal effect.
Strengths: IV methods can help mitigate the effects of confounding and endogeneity, leading to more accurate estimates of causal effects.
Limitations: Finding a valid instrumental variable can be challenging. The IV must satisfy strict conditions, including relevance (it must be correlated with the independent variable) and exogeneity (it must not directly affect the dependent variable).
4. Regression Discontinuity Design (RDD)
RDD is a quasi-experimental design used when assignment to treatment is not entirely random but based on a continuous variable that has a cutoff point. For example, students might be assigned to a special program based on their test scores, with a cutoff score determining eligibility. RDD examines the discontinuity in the outcome variable around the cutoff point to estimate the causal effect of the treatment.
Strengths: RDD offers a powerful approach for estimating causal effects when randomization is not possible, leveraging the natural experiment created by the cutoff point.
Limitations: The validity of RDD relies on the assumption that individuals near the cutoff point are similar, except for their treatment status. This assumption can be violated if individuals manipulate their scores to qualify for the treatment.
5. Mendelian Randomization
Mendelian randomization (MR) is a powerful approach for investigating causal relationships using genetic variants as instrumental variables. It leverages the random assignment of genes during meiosis, allowing researchers to analyze the association between genetic predisposition and an outcome, effectively mitigating confounding.
Strengths: MR provides a unique opportunity to address confounding issues, offering a stronger basis for causal inference compared to traditional observational studies.
Limitations: MR relies on several assumptions, including that the genetic variant is strongly associated with the exposure, the genetic variant does not affect the outcome through other pathways, and there is no pleiotropy (the genetic variant does not affect other traits that influence the outcome).
Challenges in Establishing Causation
Generating robust findings about causation often presents significant challenges:
-
Confounding Variables: These are extraneous variables that influence both the independent and dependent variables, making it difficult to isolate the effect of the independent variable. Careful study design and statistical adjustments are crucial for minimizing the influence of confounding variables.
-
Reverse Causality: This occurs when the direction of the causal relationship is misinterpreted. For example, a study might find that people with higher levels of stress also have higher blood pressure. However, it's possible that high blood pressure leads to increased stress, rather than the other way around.
-
Temporal Precedence: To establish causation, it must be demonstrated that the cause precedes the effect. This often requires longitudinal studies that follow participants over time.
-
Statistical Power: A study needs sufficient statistical power to detect a causal relationship if it exists. Low power can lead to false negative results (failing to detect a true effect).
-
Generalizability: Findings from one study might not generalize to other populations or settings. Factors such as age, gender, ethnicity, and socioeconomic status can influence the effect of an intervention.
Strengthening Causal Inference
To enhance the robustness of causal inferences, researchers should employ a range of strategies:
-
Careful Study Design: This includes selecting appropriate study designs (RCTs when feasible, well-designed observational studies when not), carefully defining variables, and developing rigorous data collection protocols.
-
Controlling for Confounders: Statistical techniques such as regression analysis, propensity score matching, and instrumental variable methods can be used to control for the influence of confounding variables.
-
Sensitivity Analyses: These analyses assess the robustness of findings by examining how results change when assumptions are relaxed or when different models are used.
-
Replication: Replicating findings in independent studies strengthens confidence in the validity of the causal relationship.
-
Mechanism Exploration: Understanding the underlying mechanisms linking the cause and effect enhances causal inference. This can involve biological, psychological, or social mechanisms.
-
Transparency and Replicability: Researchers should ensure that their study design, data, and analysis methods are transparent and easily replicable, allowing other researchers to verify the findings.
Conclusion
Establishing causal relationships is a complex and challenging endeavor. While RCTs provide the strongest evidence, various methods exist for generating causal inferences when RCTs are not feasible. By employing robust study designs, carefully controlling for confounding variables, and utilizing appropriate statistical techniques, researchers can make substantial progress in understanding causal relationships across a wide range of disciplines. Remember, however, that absolute certainty in establishing causation is rarely achievable; instead, we aim to build strong evidence supporting a causal link based on multiple lines of evidence and rigorous methodology. Continuous critical evaluation and refinement of methods are crucial in the ongoing quest for understanding the causal structures underlying complex phenomena.
Latest Posts
Latest Posts
-
How To Find A Vector Orthogonal To A Plane
May 09, 2025
-
Acids Increase The Concentration Of What In Water
May 09, 2025
-
Both Protozoans And Algae Are Found In The Kingdom
May 09, 2025
-
What Is Body Mechanics In Nursing
May 09, 2025
-
Describe The Relationship Between Population Density And Population Distribution
May 09, 2025
Related Post
Thank you for visiting our website which covers about In Order To Generate Findings About Causation . We hope the information provided has been useful to you. Feel free to contact us if you have any questions or need further assistance. See you next time and don't miss to bookmark.