Is P Value The Same As Critical Value
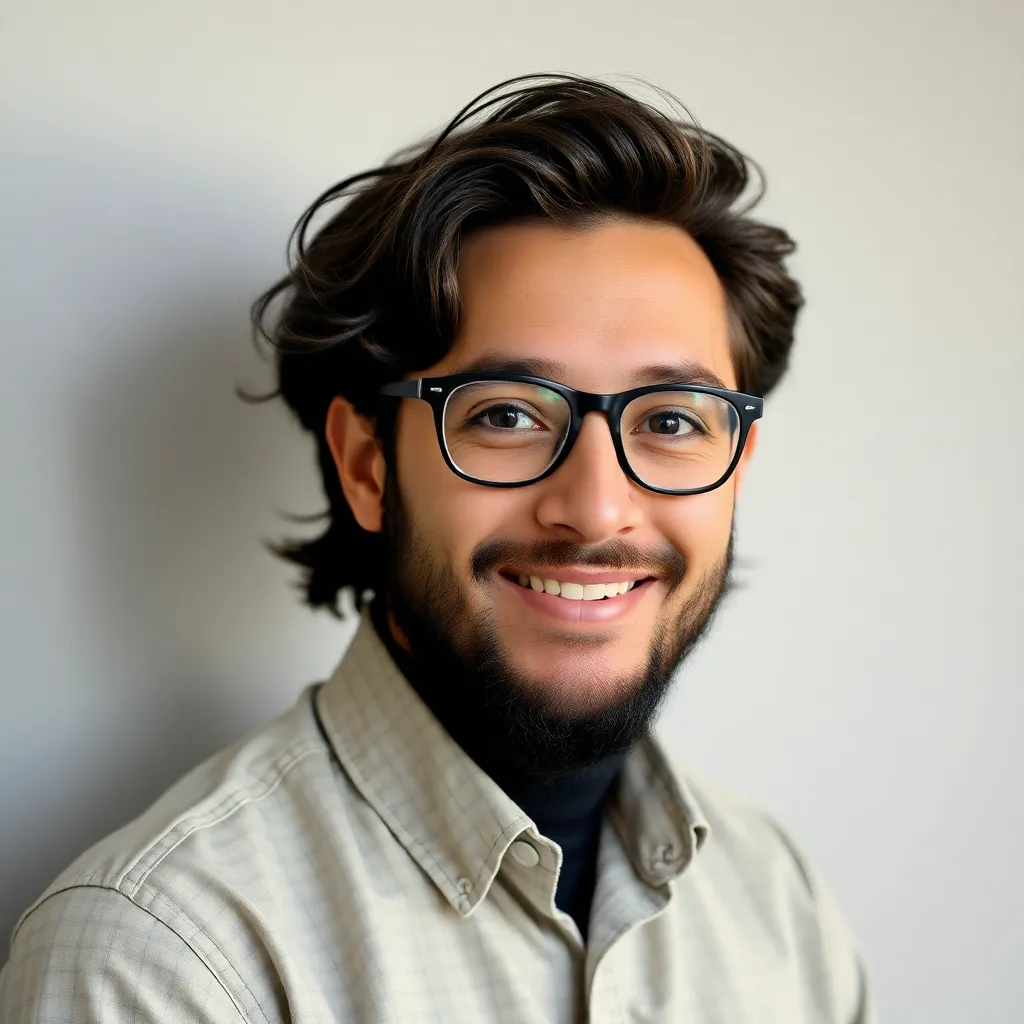
Muz Play
Apr 08, 2025 · 6 min read

Table of Contents
Is P-Value the Same as Critical Value? A Deep Dive into Statistical Significance
The concepts of p-value and critical value are fundamental in hypothesis testing, a cornerstone of statistical inference. While both are used to determine whether to reject a null hypothesis, they represent distinct approaches to the same problem, leading to frequent confusion. This article aims to clarify the differences and similarities between p-values and critical values, providing a comprehensive understanding for researchers and students alike.
Understanding Hypothesis Testing: A Quick Recap
Before diving into the nuances of p-values and critical values, let's briefly revisit the core components of hypothesis testing. Hypothesis testing involves formulating a null hypothesis (H₀), representing the status quo, and an alternative hypothesis (H₁), representing the claim we're trying to support. We collect data and use statistical tests to assess the evidence against the null hypothesis.
The process typically involves these steps:
- State the hypotheses: Define H₀ and H₁.
- Set the significance level (α): This represents the probability of rejecting the null hypothesis when it is actually true (Type I error). Commonly, α is set at 0.05 (5%).
- Calculate the test statistic: This summarizes the data's evidence against the null hypothesis.
- Determine the p-value or critical value: This is where the core distinction lies, which we'll explore in detail.
- Make a decision: Based on the p-value or critical value, we decide whether to reject or fail to reject the null hypothesis.
What is a P-Value?
The p-value is the probability of observing results as extreme as, or more extreme than, the data obtained, assuming the null hypothesis is true. In simpler terms, it quantifies the strength of evidence against the null hypothesis.
-
Interpretation: A small p-value (typically less than α) suggests strong evidence against the null hypothesis, leading to its rejection. A large p-value suggests weak evidence against the null hypothesis, leading to a failure to reject it. It's crucial to remember that failing to reject the null hypothesis does not prove the null hypothesis is true.
-
Calculation: The exact method for calculating the p-value depends on the specific statistical test used (e.g., t-test, chi-square test, ANOVA). Statistical software packages readily provide p-values.
-
Advantages: P-values offer a direct measure of the evidence against the null hypothesis, providing a continuous scale of significance. They are easily interpretable and widely used in scientific literature.
-
Disadvantages: The reliance solely on p-values has been criticized for several reasons. The most significant is the potential for misinterpretations. A p-value doesn't measure the size of an effect, nor does it reflect the importance or practical significance of the findings. Furthermore, focusing solely on a pre-defined threshold (e.g., α = 0.05) can lead to flawed conclusions.
What is a Critical Value?
The critical value is a threshold value determined from the sampling distribution of the test statistic, under the assumption that the null hypothesis is true. It's the boundary that separates the rejection region from the non-rejection region.
-
Interpretation: If the calculated test statistic falls within the rejection region (i.e., beyond the critical value), we reject the null hypothesis. If it falls within the non-rejection region, we fail to reject the null hypothesis.
-
Calculation: Critical values are obtained from statistical tables or calculated using statistical software. They depend on the significance level (α), the degrees of freedom, and the type of statistical test being used.
-
Advantages: The critical value approach is straightforward and provides a clear decision rule. It emphasizes the pre-determined significance level and avoids the potential ambiguity associated with p-values.
-
Disadvantages: The critical value approach can be less informative than the p-value approach, as it only indicates whether to reject or fail to reject the null hypothesis without providing a measure of the strength of evidence.
Key Differences between P-Value and Critical Value
Feature | P-Value | Critical Value |
---|---|---|
Definition | Probability of observing data as extreme as, or more extreme than, the obtained data, assuming H₀ is true. | Threshold value separating the rejection and non-rejection regions of the test statistic's sampling distribution. |
Interpretation | Measures the strength of evidence against H₀. | Determines whether the test statistic falls in the rejection or non-rejection region. |
Output | Probability (between 0 and 1) | Specific value based on the test statistic's distribution |
Decision Rule | Reject H₀ if p-value < α | Reject H₀ if test statistic > critical value (for one-tailed tests), or if test statistic falls outside the critical values (for two-tailed tests). |
Information | Provides a measure of evidence strength | Provides a binary decision (reject/fail to reject) |
Are They Ever the Same?
While the methods differ, the decisions reached using p-values and critical values should, in principle, be the same. If the p-value is less than α, the test statistic will invariably fall in the rejection region defined by the critical value. Conversely, if the p-value is greater than or equal to α, the test statistic will fall in the non-rejection region.
The discrepancy may arise only due to rounding errors in calculations, which are generally insignificant.
The Importance of Context and Effect Size
It's crucial to remember that neither p-values nor critical values, in isolation, tell the complete story. A statistically significant result (p-value < α) doesn't automatically imply practical significance. The magnitude of the effect should always be considered. Effect size measures quantify the practical importance of the findings.
For instance, a statistically significant difference between two groups might be of little practical relevance if the difference is tiny. Conversely, a non-significant result might still represent a meaningful effect if the sample size was small, limiting the statistical power to detect it.
Beyond P-values and Critical Values: Modern Approaches
The widespread reliance on p-values and a rigid adherence to the 0.05 significance level have been subject to intense scrutiny. Statisticians and researchers are increasingly advocating for a shift toward more nuanced approaches to statistical inference, such as:
-
Confidence intervals: These provide a range of plausible values for the population parameter of interest, offering more information than a simple p-value.
-
Bayesian methods: These incorporate prior knowledge and beliefs into the analysis, providing a more holistic perspective.
-
Emphasis on effect size and practical significance: Focusing on the magnitude of the effect and its real-world implications provides a more balanced assessment.
-
Reporting full data and methodology: Transparency in data and methods allows others to scrutinize the findings and assess the limitations of the study.
Conclusion: Understanding the Nuances
While p-values and critical values are closely related and typically lead to the same conclusion in hypothesis testing, they represent distinct ways of approaching the problem. P-values provide a probabilistic measure of evidence against the null hypothesis, while critical values offer a straightforward decision rule based on thresholds. It’s crucial to understand both concepts to critically evaluate statistical analyses. However, relying solely on either without considering effect size, confidence intervals, or broader statistical context can lead to misinterpretations and misleading conclusions. A more holistic and nuanced approach to statistical inference is essential for drawing meaningful insights from data.
Latest Posts
Latest Posts
-
How To Know If A Compound Is Soluble
Apr 16, 2025
-
All Living Organisms Are Composed Of One Or More
Apr 16, 2025
-
What Do Triglycerides And Phospholipids Have In Common
Apr 16, 2025
-
How Is Antigenic Drift Beneficial For Viruses
Apr 16, 2025
-
Difference Between Silent And Neutral Mutation
Apr 16, 2025
Related Post
Thank you for visiting our website which covers about Is P Value The Same As Critical Value . We hope the information provided has been useful to you. Feel free to contact us if you have any questions or need further assistance. See you next time and don't miss to bookmark.