Is The Null Hypothesis Always Equal
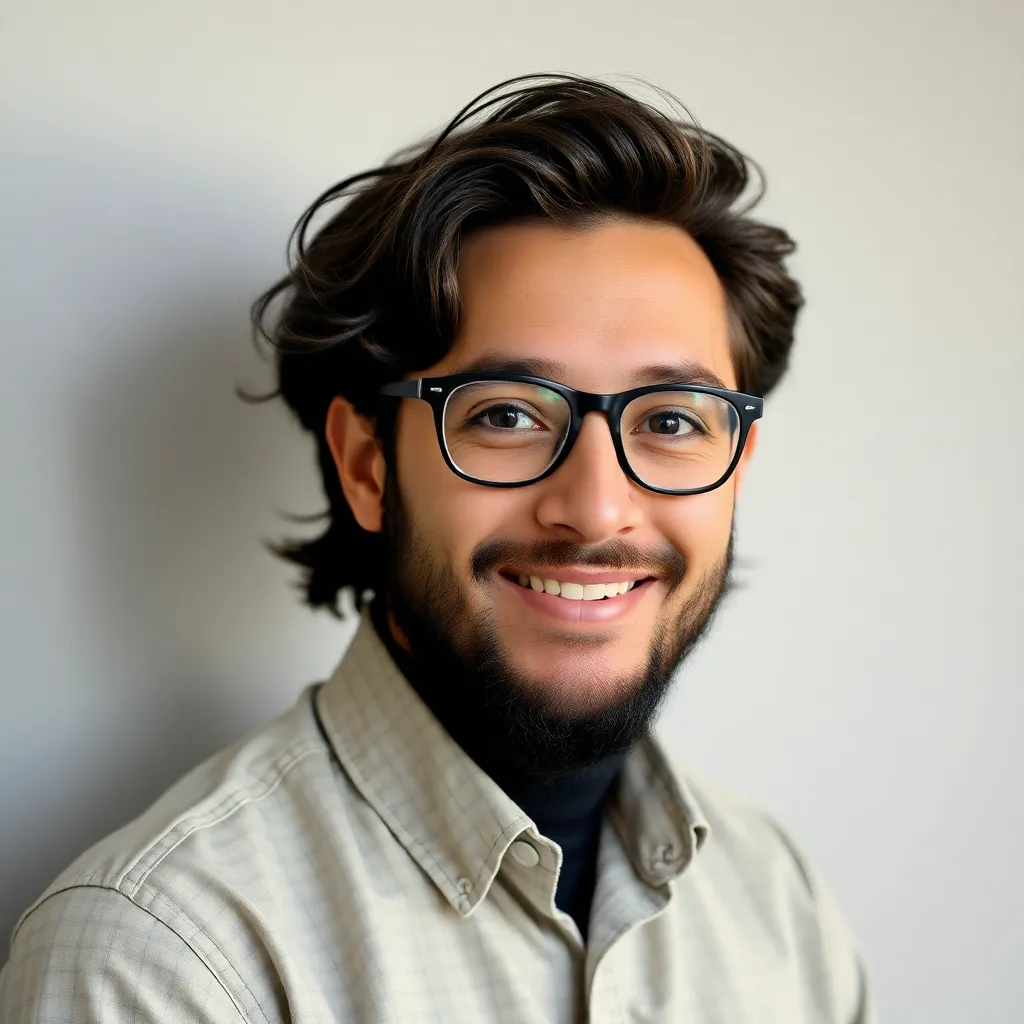
Muz Play
Apr 21, 2025 · 5 min read

Table of Contents
Is the Null Hypothesis Always Equal? A Deep Dive into Hypothesis Testing
The null hypothesis, a cornerstone of statistical hypothesis testing, often sparks confusion. A common misconception revolves around its relationship with equality: is the null hypothesis always stated as an equality? The short answer is no. While many introductory statistics courses focus on null hypotheses framed as equalities (e.g., μ₁ = μ₂), the reality is more nuanced. This article delves deep into the nature of the null hypothesis, exploring its various forms and clarifying the circumstances under which it might—or might not—involve an equality statement.
Understanding the Core Concept of the Null Hypothesis
Before we dissect the nuances of equality, let's establish a firm understanding of the null hypothesis itself. In essence, the null hypothesis (H₀) represents a statement of no effect, no difference, or no relationship between variables. It's the default assumption we begin with, the status quo we aim to challenge with our data. We don't expect to prove the null hypothesis; instead, we seek to find evidence to reject it in favor of an alternative hypothesis (H₁ or Hₐ).
Example: Let's say we're comparing the average height of men and women.
- Null Hypothesis (H₀): There is no difference in the average height of men and women (μ₁ = μ₂).
- Alternative Hypothesis (H₁): There is a difference in the average height of men and women (μ₁ ≠ μ₂).
This is a classic example of a null hypothesis expressed as an equality. However, the formulation can be different depending on the research question and the type of test employed.
Beyond Equality: One-Tailed and Two-Tailed Tests
The form of the null hypothesis is intimately linked to the type of hypothesis test conducted. We often encounter two types:
1. Two-Tailed Tests: The Equality Scenario
Two-tailed tests are designed to detect differences in either direction. In our height example above, we are interested in detecting whether men are taller or shorter than women. The null hypothesis, as shown earlier, is expressed as an equality (μ₁ = μ₂). The alternative hypothesis is a non-directional statement (μ₁ ≠ μ₂), encompassing both possibilities. The rejection region lies in both tails of the sampling distribution.
Key Characteristics:
- Null Hypothesis: Statement of equality (e.g., μ₁ = μ₂, p = 0.5).
- Alternative Hypothesis: Non-directional statement of inequality (e.g., μ₁ ≠ μ₂, p ≠ 0.5).
- Rejection Region: Located in both tails of the sampling distribution.
2. One-Tailed Tests: Beyond Simple Equality
One-tailed tests are more specific. They are used when we have a directional hypothesis – we predict the direction of the difference or effect.
Example: Let's say we hypothesize that a new drug reduces blood pressure.
- Null Hypothesis (H₀): The new drug does not reduce blood pressure (μ₁ ≥ μ₂). The null hypothesis here isn't strictly an equality; it encompasses all scenarios where the new drug's effect is equal to or greater than the existing treatment.
- Alternative Hypothesis (H₁): The new drug reduces blood pressure (μ₁ < μ₂).
This is a left-tailed test, because the rejection region is located in the left tail of the sampling distribution. We are only interested in detecting a reduction in blood pressure, not an increase.
Similarly, we can have a right-tailed test. For instance, if we hypothesize that a new fertilizer increases crop yield, the null hypothesis could be formulated as (μ₁ ≤ μ₂), encompassing scenarios where the yield remains the same or decreases.
Key Characteristics of One-Tailed Tests:
- Null Hypothesis: Statement of equality or inequality depending on the direction of the alternative hypothesis (e.g., μ₁ ≥ μ₂, μ₁ ≤ μ₂).
- Alternative Hypothesis: Directional statement of inequality (e.g., μ₁ < μ₂, μ₁ > μ₂).
- Rejection Region: Located in a single tail (left or right) of the sampling distribution.
Null Hypotheses in Different Statistical Tests
The way the null hypothesis is formulated also varies depending on the statistical test being used.
-
t-tests: Often involve comparing means, with the null hypothesis frequently stated as equality between means (e.g., H₀: μ₁ = μ₂ for an independent samples t-test). However, as discussed, one-tailed tests can also be used.
-
ANOVA (Analysis of Variance): Compares means across multiple groups. The null hypothesis generally states that all group means are equal (e.g., H₀: μ₁ = μ₂ = μ₃ = ... = μₖ).
-
Chi-square tests: Used to analyze categorical data. The null hypothesis typically states that there is no association between variables. It doesn't usually involve an explicit equality statement, but rather independence between variables.
-
Correlation tests: Examine the relationship between variables. The null hypothesis usually states that there is no correlation between the variables (ρ = 0). This is an equality statement, but the interpretation is different from comparing means.
The Importance of Correct Null Hypothesis Formulation
Accurately defining the null hypothesis is critical for several reasons:
-
Clear Research Question: A well-defined null hypothesis ensures a clear focus on the research question.
-
Appropriate Statistical Test: The null hypothesis guides the choice of the appropriate statistical test.
-
Valid Interpretation: A correctly formulated null hypothesis is essential for drawing valid conclusions from statistical analyses. An incorrect null hypothesis can lead to inaccurate conclusions and flawed interpretations.
-
Reproducibility and Transparency: Clearly stated hypotheses enhance the reproducibility and transparency of research.
Practical Considerations and Common Pitfalls
Here are some common pitfalls to avoid when formulating null hypotheses:
-
Failing to Consider Directional Hypotheses: Ignoring directional hypotheses when appropriate can lead to missed opportunities to detect effects of interest.
-
Overly Complex Hypotheses: Keep your hypotheses concise and focused. Avoid overly complex or ambiguous statements.
-
Confusing Null and Alternative Hypotheses: Be clear about the distinction between the null and alternative hypotheses.
-
Incorrect Interpretation of p-values: The p-value represents the probability of obtaining results as extreme as, or more extreme than, the observed results, assuming the null hypothesis is true. It does not prove or disprove the null hypothesis.
Conclusion: A Versatile Tool, Not a Rigid Rule
While the null hypothesis is frequently presented as an equality statement, especially in introductory statistics, it's crucial to recognize its broader role and adaptability. The form of the null hypothesis hinges on the research question, the nature of the data, and the selected statistical test. Understanding these nuances is paramount for conducting sound statistical analyses and interpreting results accurately. The key is to carefully formulate the null hypothesis based on the specific research objective, ensuring it accurately reflects the research question and guides the appropriate statistical test selection. This careful consideration ensures the robustness and validity of your research findings. The null hypothesis, while often simplified to equality in introductory contexts, serves as a highly versatile tool in the broader landscape of statistical inference.
Latest Posts
Latest Posts
-
One Of The Primary Functions Of Rna Molecules Is To
Apr 21, 2025
-
Which Elements Has Only One Valence Electron
Apr 21, 2025
-
Co2 Enters The Inner Spaces Of The Leaf Through The
Apr 21, 2025
-
How To Do Average Atomic Mass
Apr 21, 2025
-
What Is The Most Abundant Mineral In The Human Body
Apr 21, 2025
Related Post
Thank you for visiting our website which covers about Is The Null Hypothesis Always Equal . We hope the information provided has been useful to you. Feel free to contact us if you have any questions or need further assistance. See you next time and don't miss to bookmark.