Is The P Value The Critical Value
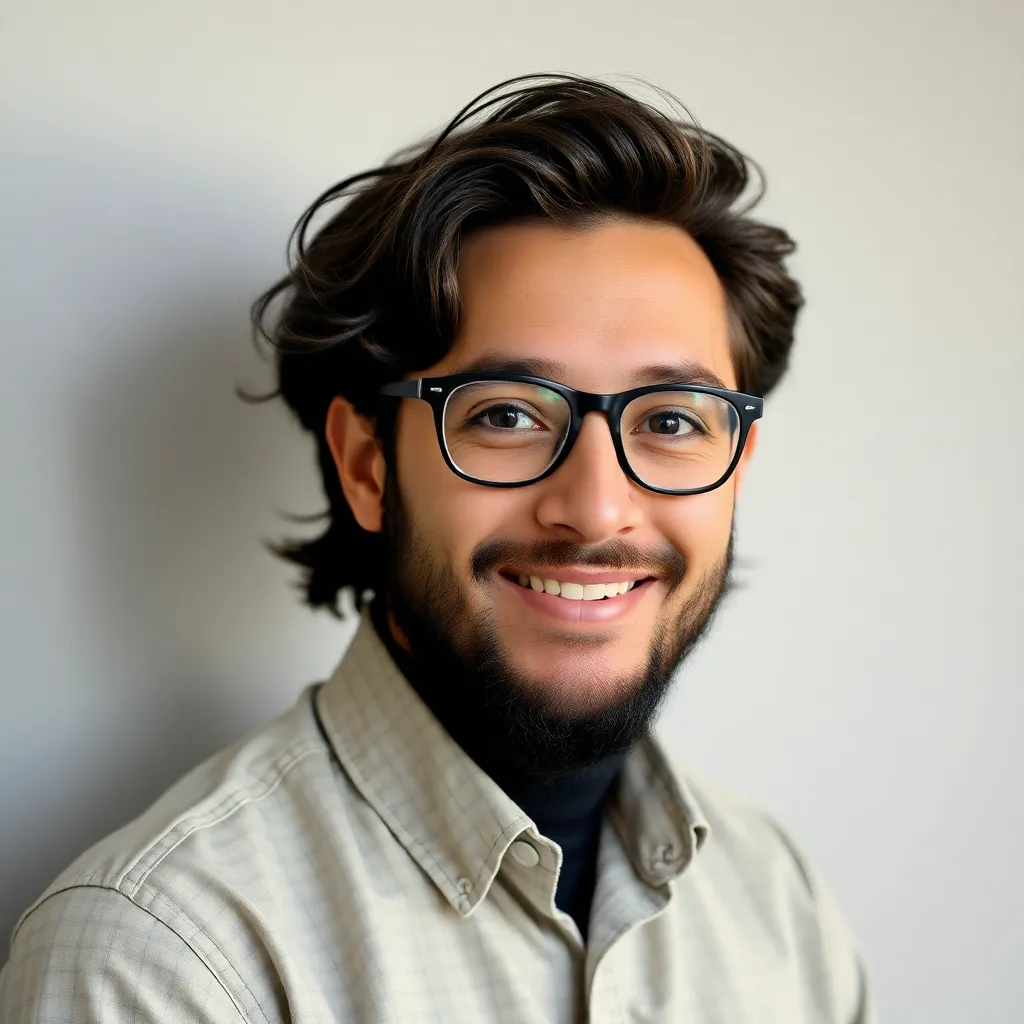
Muz Play
Apr 10, 2025 · 6 min read

Table of Contents
Is the P-value the Critical Value? Understanding the Nuances of Statistical Significance
The question, "Is the p-value the critical value?", is a common point of confusion in statistics. While both p-values and critical values play crucial roles in hypothesis testing, they are distinct concepts with different interpretations and applications. Understanding their differences is vital for accurate statistical inference and avoiding misinterpretations of research findings. This article will delve deep into the nuances of p-values and critical values, clarifying their relationship and highlighting the potential pitfalls of conflating the two.
Understanding P-values: The Probability of Observing Data
The p-value represents the probability of obtaining results as extreme as, or more extreme than, the observed results, assuming the null hypothesis is true. In simpler terms, it quantifies the evidence against the null hypothesis. A small p-value suggests that the observed data are unlikely to have occurred by chance alone if the null hypothesis were true, leading us to reject the null hypothesis.
Key characteristics of a p-value:
- It's a probability: The p-value is always a number between 0 and 1.
- It's conditional: It's the probability given that the null hypothesis is true.
- It's not the probability that the null hypothesis is true: This is a common misconception. The p-value doesn't tell us the probability of the null hypothesis being true or false.
- It depends on sample size: Larger sample sizes can lead to smaller p-values, even with small effect sizes.
- It's influenced by effect size and variability: A larger effect size and smaller variability generally result in smaller p-values.
Example: Imagine testing the effectiveness of a new drug. The null hypothesis is that the drug has no effect. A p-value of 0.01 would suggest that there's only a 1% chance of observing the drug's effect if it were truly ineffective. This low probability strengthens the evidence against the null hypothesis, supporting the conclusion that the drug is effective.
Understanding Critical Values: The Threshold for Rejection
The critical value is a threshold used in hypothesis testing to decide whether to reject the null hypothesis. It's determined by the significance level (α), usually set at 0.05 (5%), and the distribution of the test statistic (e.g., t-distribution, F-distribution, z-distribution). If the calculated test statistic exceeds the critical value (in absolute terms), we reject the null hypothesis.
Key characteristics of a critical value:
- It's a threshold: It defines the boundary between accepting and rejecting the null hypothesis.
- It depends on the significance level (α): A smaller α leads to a more stringent criterion for rejecting the null hypothesis, resulting in a larger critical value.
- It depends on the degrees of freedom: For many tests, the critical value also depends on the degrees of freedom, which are related to sample size.
- It's related to the test statistic: The critical value is a specific value of the test statistic that corresponds to the chosen significance level.
Example: Let's consider a t-test with a significance level of 0.05 and 10 degrees of freedom. The critical value might be approximately 2.23. If the calculated t-statistic is greater than 2.23 or less than -2.23, we reject the null hypothesis.
The Relationship Between P-values and Critical Values
While distinct, p-values and critical values are intimately linked in hypothesis testing. They both contribute to the decision of whether to reject the null hypothesis. The relationship can be summarized as follows:
- If the p-value is less than or equal to the significance level (α), the test statistic will exceed the critical value (in absolute terms), leading to the rejection of the null hypothesis. This is equivalent to saying that the observed result falls within the rejection region.
- If the p-value is greater than the significance level (α), the test statistic will not exceed the critical value, and we fail to reject the null hypothesis. This means the observed result falls within the acceptance region.
The Pitfalls of Confusing P-values and Critical Values
The crucial difference between p-values and critical values is often overlooked, leading to several potential pitfalls:
- Misinterpretation of p-values: A common mistake is interpreting the p-value as the probability that the null hypothesis is true. This is incorrect. The p-value only reflects the probability of observing the data given the null hypothesis is true.
- Overemphasis on significance levels: Focusing solely on whether the p-value is below a predefined significance level (e.g., 0.05) without considering the effect size and context can lead to misleading conclusions. A statistically significant result doesn't necessarily imply practical significance.
- Ignoring the limitations of p-values: P-values are susceptible to various factors, including sample size and the presence of outliers, which can influence their interpretation.
- Ignoring the possibility of Type I and Type II errors: Even with a statistically significant result (p-value < α), there's always a chance of making a Type I error (rejecting a true null hypothesis). Conversely, a non-significant result (p-value > α) doesn't necessarily mean the null hypothesis is true; there could be a Type II error (failing to reject a false null hypothesis).
Beyond P-values and Critical Values: Effect Size and Confidence Intervals
While p-values and critical values are essential components of hypothesis testing, a comprehensive analysis should extend beyond these metrics. Consider incorporating the following:
- Effect size: This quantifies the magnitude of the observed effect, providing a measure of practical significance. A large effect size is more meaningful than a small effect size, even if both are statistically significant.
- Confidence intervals: These provide a range of plausible values for the population parameter, giving a more nuanced understanding of the effect's uncertainty than a simple p-value.
Conclusion: A Holistic Approach to Statistical Inference
While p-values and critical values serve important roles in hypothesis testing, they should not be the sole basis for drawing conclusions. A complete understanding of their relationship, coupled with an appreciation for effect sizes, confidence intervals, and the limitations of hypothesis testing, is essential for sound statistical inference and accurate interpretation of research findings. Researchers should strive for a holistic approach that considers all relevant information before drawing conclusions. Focusing solely on p-values and critical values without considering the broader context can lead to misleading or even erroneous interpretations, hindering scientific progress and informed decision-making. Understanding the nuances of these concepts and their interconnectedness is vital for anyone involved in statistical analysis and interpretation. The emphasis should always be on a comprehensive understanding of the data and the context in which the analysis is being performed. Statistical significance is only one piece of a much larger puzzle.
Latest Posts
Latest Posts
-
Cation And Anion Held Together By Electrostatic Forces
Apr 18, 2025
-
The Metals In Groups 1a 2a And 3a
Apr 18, 2025
-
Is A Fat Or Phospholipid Less Soluble In Water
Apr 18, 2025
-
What Is The Total Magnification Of The Low Power Objective
Apr 18, 2025
-
Bacteriocins And Defensins Are Types Of Which Of The Following
Apr 18, 2025
Related Post
Thank you for visiting our website which covers about Is The P Value The Critical Value . We hope the information provided has been useful to you. Feel free to contact us if you have any questions or need further assistance. See you next time and don't miss to bookmark.