Law Of Large Number Population Mean
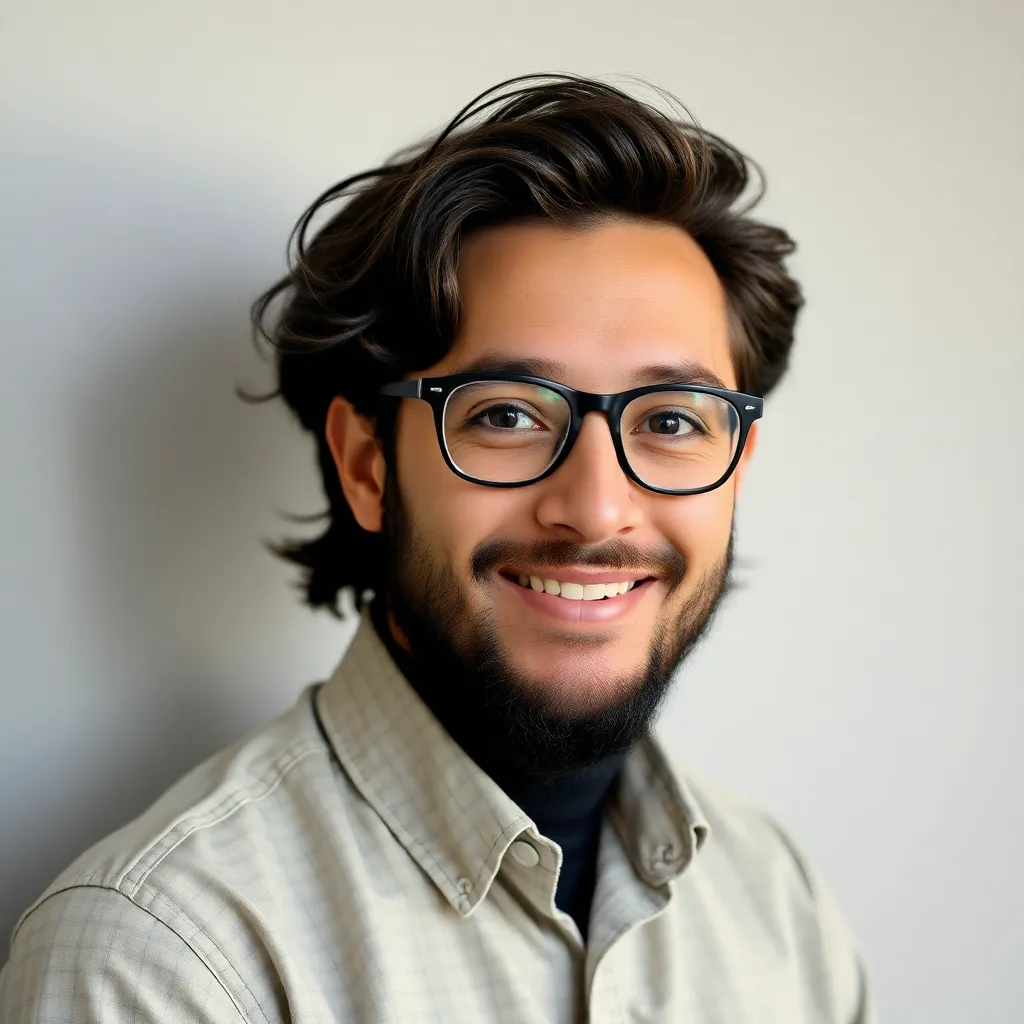
Muz Play
Mar 12, 2025 · 6 min read

Table of Contents
The Law of Large Numbers and Population Mean: Understanding the Fundamentals of Statistical Inference
The Law of Large Numbers (LLN) is a cornerstone of statistics, providing a crucial link between theoretical probability and observed frequencies. It underpins much of statistical inference, allowing us to make reliable estimates about population parameters based on sample data. This article will delve into the intricacies of the LLN, particularly its relationship to the population mean, exploring its implications, applications, and limitations.
Understanding the Law of Large Numbers
The LLN essentially states that as the number of independent and identically distributed (i.i.d.) random variables in a sample increases, the sample average converges towards the expected value (population mean) of the underlying distribution. In simpler terms, the more data you collect, the closer your sample average will get to the true population average.
This doesn't mean that the sample average will exactly equal the population mean, even with an extremely large sample size. Instead, the LLN guarantees that the probability of the sample average being arbitrarily close to the population mean approaches 1 as the sample size grows infinitely large.
There are two main versions of the LLN: the weak law and the strong law.
The Weak Law of Large Numbers
The weak law states that for any positive number ε (epsilon), the probability that the sample average deviates from the population mean by more than ε approaches zero as the sample size (n) tends to infinity. Formally:
lim (n→∞) P(|X̄ - μ| > ε) = 0
where:
X̄
represents the sample average.μ
represents the population mean.ε
represents a small positive number.P()
denotes probability.
This means that the probability of a large discrepancy between the sample average and the population mean becomes increasingly small as the sample size increases.
The Strong Law of Large Numbers
The strong law is a more powerful statement. It asserts that the sample average converges almost surely to the population mean as the sample size approaches infinity. "Almost surely" means that the probability of the sample average converging to the population mean is 1. Formally:
P(lim (n→∞) X̄ = μ) = 1
The strong law is a stronger statement than the weak law because it implies that the sample average will eventually get arbitrarily close to the population mean and stay there with probability 1. The weak law only guarantees that the probability of a large deviation decreases with increasing sample size.
Population Mean and its Estimation
The population mean (μ) is a crucial parameter in statistics. It represents the average value of a characteristic across the entire population. Often, it's impossible or impractical to measure the characteristic for every individual in a population. This is where the LLN and sampling come into play. We use the sample mean (X̄) as an estimator of the population mean.
The sample mean is calculated by summing all the values in a sample and dividing by the sample size (n):
X̄ = (Σxi) / n
where:
xi
represents the i-th value in the sample.
The LLN assures us that, with a sufficiently large sample size, the sample mean (X̄) provides a reliable estimate of the population mean (μ).
Implications and Applications of the LLN in Estimating Population Mean
The LLN has profound implications across numerous fields, impacting our ability to make informed decisions based on data:
1. Market Research and Forecasting:
In market research, the LLN is crucial for estimating population preferences, brand awareness, and purchasing behaviors. By surveying a sufficiently large sample of the target population, researchers can extrapolate their findings to make predictions about the entire market. The accuracy of these predictions is directly related to the sample size and the LLN’s guarantee of convergence to the population mean.
2. Actuarial Science and Insurance:
Insurance companies rely heavily on the LLN to assess risk and set premiums. By analyzing a large number of past claims, they can estimate the average claim amount for a given population. This allows them to calculate premiums that are statistically sound and ensure the financial solvency of the company. The LLN provides the foundation for accurate risk assessment and pricing models.
3. Quality Control and Manufacturing:
In manufacturing, the LLN is used to monitor product quality. By randomly sampling products from a production line and measuring a key characteristic (e.g., weight, length, strength), manufacturers can estimate the average value of that characteristic for the entire production batch. This allows them to identify and correct any deviations from the desired specifications, ensuring consistent product quality.
4. Scientific Research and Experimentation:
Scientific experiments often involve collecting large datasets to test hypotheses and draw conclusions. The LLN provides a theoretical basis for the validity of using sample statistics to infer population parameters. For instance, in clinical trials, researchers use sample data to estimate the efficacy of a new drug, relying on the LLN to ensure that the sample results are representative of the larger population.
5. Finance and Investment:
In finance, the LLN plays a role in portfolio diversification and risk management. By investing in a large number of uncorrelated assets, investors can reduce the overall risk of their portfolio. The LLN helps explain how the average return of a diversified portfolio tends to converge towards the expected return of the underlying assets.
Limitations and Considerations
While the LLN is a powerful tool, it's essential to be aware of its limitations:
1. Sample Independence and Identical Distribution:
The LLN relies on the assumption that the samples are independent and identically distributed (i.i.d.). If the samples are not independent (e.g., due to autocorrelation or clustering), or if they do not come from the same distribution, the LLN may not hold. Violation of these assumptions can lead to biased estimates and inaccurate conclusions.
2. Sample Size Requirements:
While the LLN guarantees convergence in the limit as the sample size approaches infinity, it doesn't specify how large the sample needs to be for a reasonable approximation of the population mean. The required sample size depends on factors such as the variability of the underlying distribution, the desired level of accuracy, and the acceptable margin of error. Determining an appropriate sample size often requires careful statistical planning.
3. Outliers and Extreme Values:
Outliers, or extreme values, can significantly influence the sample mean, especially in smaller samples. While the LLN suggests that the impact of outliers will diminish with increasing sample size, it doesn't eliminate the possibility that a few extreme values could still distort the estimate, particularly in heavily skewed distributions. Robust statistical methods may be necessary to mitigate the effects of outliers.
4. Non-representative Samples:
The LLN only applies if the sample is representative of the population. If the sampling method introduces bias (e.g., through selection bias or undercoverage), the sample mean may not be a good estimate of the population mean, regardless of the sample size. Careful sampling techniques are essential to ensure the representativeness of the sample.
Conclusion
The Law of Large Numbers is a fundamental principle in statistics with far-reaching implications. It provides a theoretical justification for using sample means to estimate population means, enabling us to make informed decisions and inferences based on data analysis across various disciplines. Understanding its implications, limitations, and appropriate applications is crucial for conducting reliable statistical analyses and drawing valid conclusions. While the LLN guarantees convergence to the population mean with a sufficiently large sample, careful consideration of sample independence, distribution, size, and representativeness is vital for ensuring the accuracy and reliability of the estimates. By acknowledging these factors, we can harness the power of the LLN to extract meaningful insights from data and make sound judgements based on statistical evidence.
Latest Posts
Latest Posts
-
A Smooth Sustained Contraction Is Called
May 09, 2025
-
Which Of The Following Is A Precipitation Reaction
May 09, 2025
-
Chromosomes Line Up Along The Equator Not In Homologous Pairs
May 09, 2025
-
Do Covalent Bonds Have Low Melting Points
May 09, 2025
-
Class Ii Mhc Molecules Are Found Primarily On
May 09, 2025
Related Post
Thank you for visiting our website which covers about Law Of Large Number Population Mean . We hope the information provided has been useful to you. Feel free to contact us if you have any questions or need further assistance. See you next time and don't miss to bookmark.