Left Skewed Stem And Leaf Plot
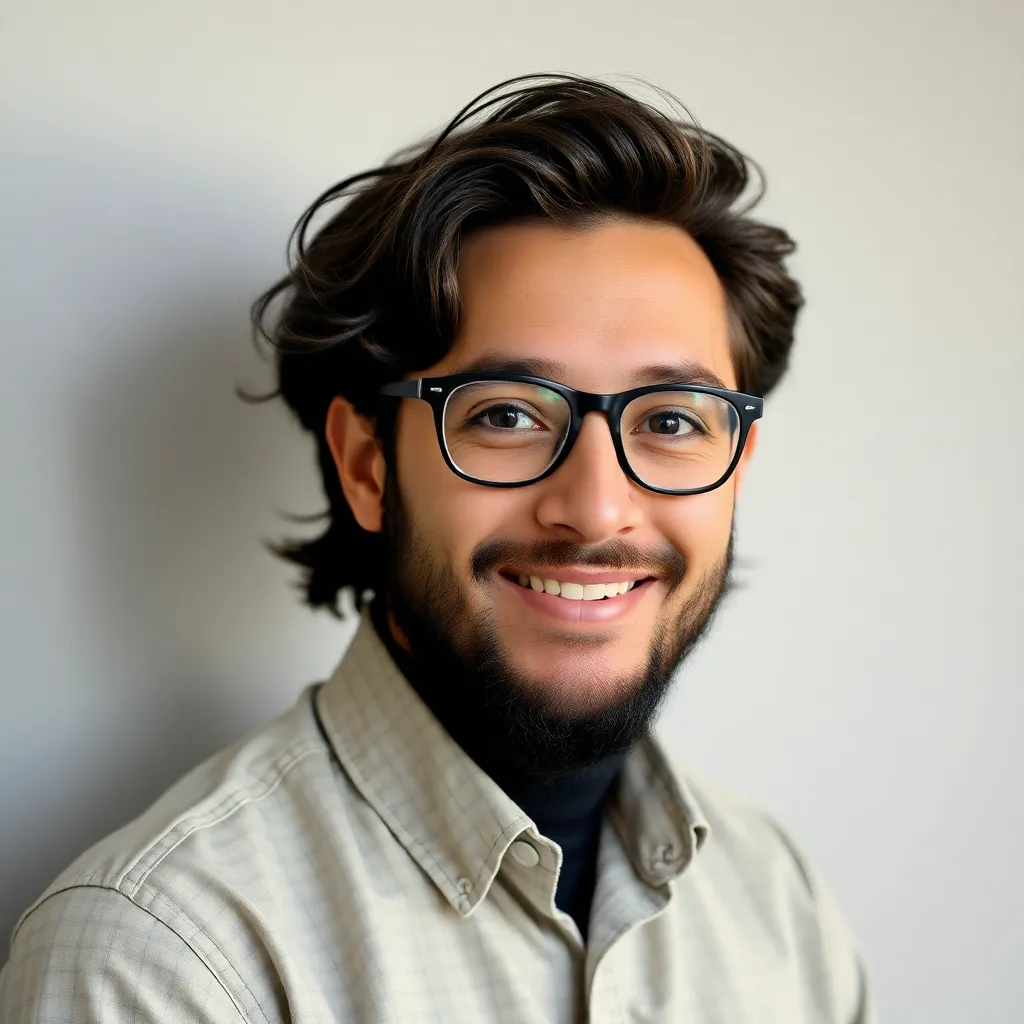
Muz Play
May 10, 2025 · 6 min read
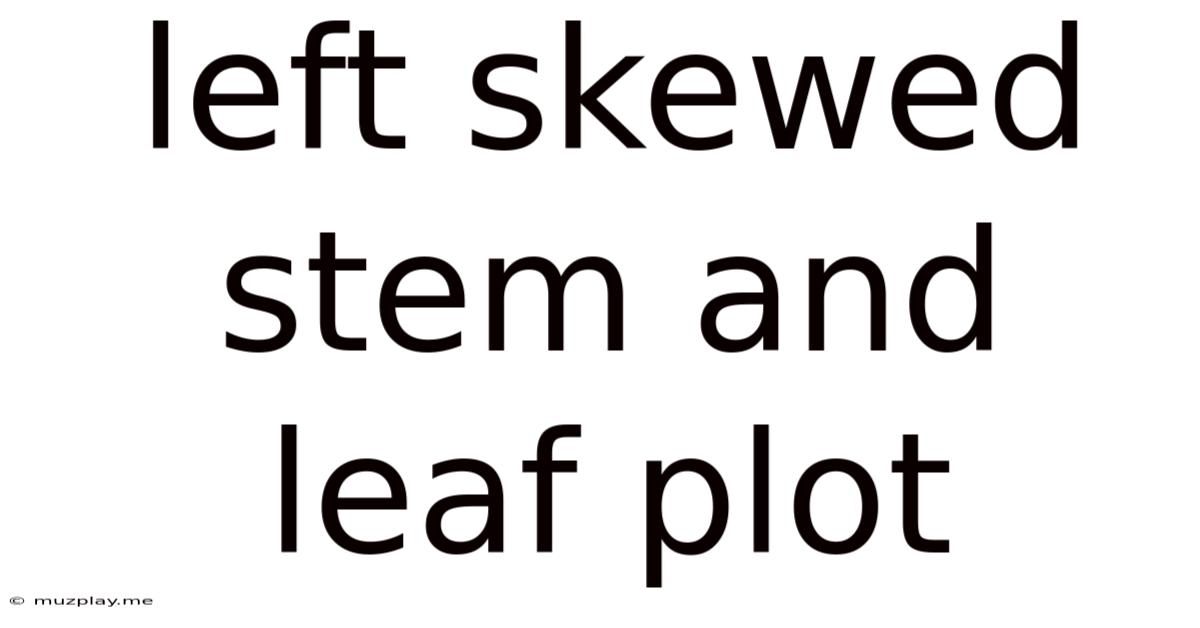
Table of Contents
Left-Skewed Stem and Leaf Plots: Understanding and Interpreting Data
A stem and leaf plot is a fantastic tool for visualizing data, particularly when dealing with smaller datasets. It offers a clear and concise way to understand the distribution of your data, highlighting central tendency, spread, and the presence of outliers. While stem and leaf plots are relatively simple, understanding how to interpret different distributions, such as a left-skewed distribution, is crucial for effective data analysis. This comprehensive guide will explore left-skewed stem and leaf plots in detail, teaching you how to identify, understand, and interpret them.
What is a Stem and Leaf Plot?
Before diving into left-skewed distributions, let's refresh our understanding of stem and leaf plots. Essentially, it's a way to organize and display numerical data in a visual format. Each data point is split into a "stem" and a "leaf." The stem represents the leading digit(s) of the data point, while the leaf represents the trailing digit(s).
For example, consider the data set: 12, 15, 18, 21, 23, 25, 29, 31, 32.
A stem and leaf plot for this data would look like this:
Stem | Leaf
-----|-----
1 | 2 5 8
2 | 1 3 5 9
3 | 1 2
Here, the stems are the tens digits (1, 2, 3), and the leaves are the units digits. This plot quickly shows the distribution of the data, allowing for a clear visualization of the frequency of different values.
Understanding Skewness
Skewness refers to the asymmetry of a probability distribution. A symmetrical distribution has a perfectly balanced distribution of data around the mean. In contrast, a skewed distribution has more data clustered on one side of the mean than the other.
We categorize skewness into two main types:
- Left Skewed (Negatively Skewed): The tail of the distribution extends more to the left. The mean is typically less than the median, which is less than the mode.
- Right Skewed (Positively Skewed): The tail of the distribution extends more to the right. The mean is typically greater than the median, which is greater than the mode.
Identifying a Left-Skewed Stem and Leaf Plot
A left-skewed stem and leaf plot displays data with a long tail extending towards the left. Visually, this means:
- Clustered Data on the Higher End: The majority of the data points will be concentrated towards the higher values (right side of the plot).
- Sparse Data on the Lower End: There will be fewer data points scattered towards the lower values (left side of the plot).
- Outliers on the Left: Extreme values (outliers) are more likely to be present on the left side of the distribution, pulling the tail out to the left.
Consider this example of a left-skewed stem and leaf plot:
Stem | Leaf
-----|-----
1 | 5 8 9
2 | 1 2 4 6
3 | 0 2 3 5 7 8 9
4 | 1 3 5
5 | 0
Notice how the data is densely packed towards the stems 3 and 4, while there are only a few values at the lower end (stem 1). The data points at the lower end are further apart which also contributes to the left skew. This visual representation directly indicates a left-skewed distribution.
Interpreting a Left-Skewed Stem and Leaf Plot
Interpreting a left-skewed stem and leaf plot involves understanding the implications of this type of data distribution. Here are some key points to consider:
- Mean < Median < Mode: In a left-skewed distribution, the mean is typically the lowest value, followed by the median and then the mode. The outliers on the lower end pull the mean downward.
- Concentration of Data: A substantial portion of the data is concentrated towards the higher values. This suggests that a significant number of observations fall within a particular range of higher values.
- Presence of Outliers: The presence of outliers towards the left side of the distribution is a common characteristic of left-skewed data. These outliers can significantly influence the mean, making it a less reliable measure of central tendency than the median in such cases.
- Data Implications: The specific implications of a left-skewed distribution depend on the context of the data. For example, if the data represents income levels, a left-skewed distribution might indicate a high concentration of wealth among a small percentage of individuals, with most individuals having comparatively lower incomes.
Real-World Examples of Left-Skewed Data
Many real-world scenarios generate left-skewed data. Some common examples include:
- Test Scores: In a very easy test, most students will score high, with a few students scoring significantly lower.
- Income Distribution: In many countries, income distributions exhibit left skewness, with a large number of people earning lower incomes and a smaller number earning very high incomes.
- Age of Retirement: While the typical retirement age might be around 65, some individuals retire much earlier due to various factors, while others work much longer. This would exhibit left-skewness.
- Property Prices in an Upscale Neighborhood: The majority of houses may be in a similar high price range with some much cheaper properties (possibly due to older age or needing much renovation) at the lower tail.
Understanding the context of the data is essential when interpreting a left-skewed stem and leaf plot. The specific implications will vary depending on the nature of the variable being measured.
Comparing Left-Skewed and Other Distributions
To fully appreciate left-skewed distributions, it's helpful to compare them to other types of distributions:
- Symmetrical Distribution: A symmetrical distribution is balanced, with the mean, median, and mode all being approximately equal. The data is evenly distributed around the central tendency.
- Right-Skewed Distribution: A right-skewed distribution has a long tail extending to the right, indicating a concentration of data towards the lower values. The mean is typically greater than the median, and the median is greater than the mode.
Advanced Considerations: Outliers and Data Transformation
In many real-world datasets, outliers might heavily skew the data. In situations with extreme values impacting the overall distribution, it's crucial to investigate these outliers. They might indicate measurement errors or genuine unusual data points, requiring further analysis and careful consideration before drawing conclusions.
Data transformation techniques, such as logarithmic transformations, can help to reduce the impact of outliers and make the data distribution closer to normality (symmetrical). If the left skew is severely impacting your analysis or data visualization, a transformation might be appropriate. Remember however to carefully note that transformation has been used and the implications this has on your interpretation.
Conclusion
Left-skewed stem and leaf plots are valuable tools for understanding and visualizing data. By understanding the characteristics of a left-skewed distribution – the clustering of data at the higher end, the sparse data on the lower end, and the potential presence of outliers – you can accurately interpret the information conveyed by the plot. Remember to consider the context of the data and the implications of the skewness when drawing conclusions. With careful analysis and interpretation, left-skewed stem and leaf plots can offer valuable insights into your dataset. Combining this understanding with other statistical techniques and thoughtful consideration of outliers provides a robust approach to data analysis and visualization.
Latest Posts
Latest Posts
-
How To Find The Mad Of A Dot Plot
May 10, 2025
-
Using The General Properties Of Equilibrium Constants
May 10, 2025
-
Difference Between Electron Affinity And Electronegativity
May 10, 2025
-
What Is The Main Cause Of Any Change Of State
May 10, 2025
-
How Do You Find The Charge Of A Transition Metal
May 10, 2025
Related Post
Thank you for visiting our website which covers about Left Skewed Stem And Leaf Plot . We hope the information provided has been useful to you. Feel free to contact us if you have any questions or need further assistance. See you next time and don't miss to bookmark.