Mean Of Distribution Of Sample Means
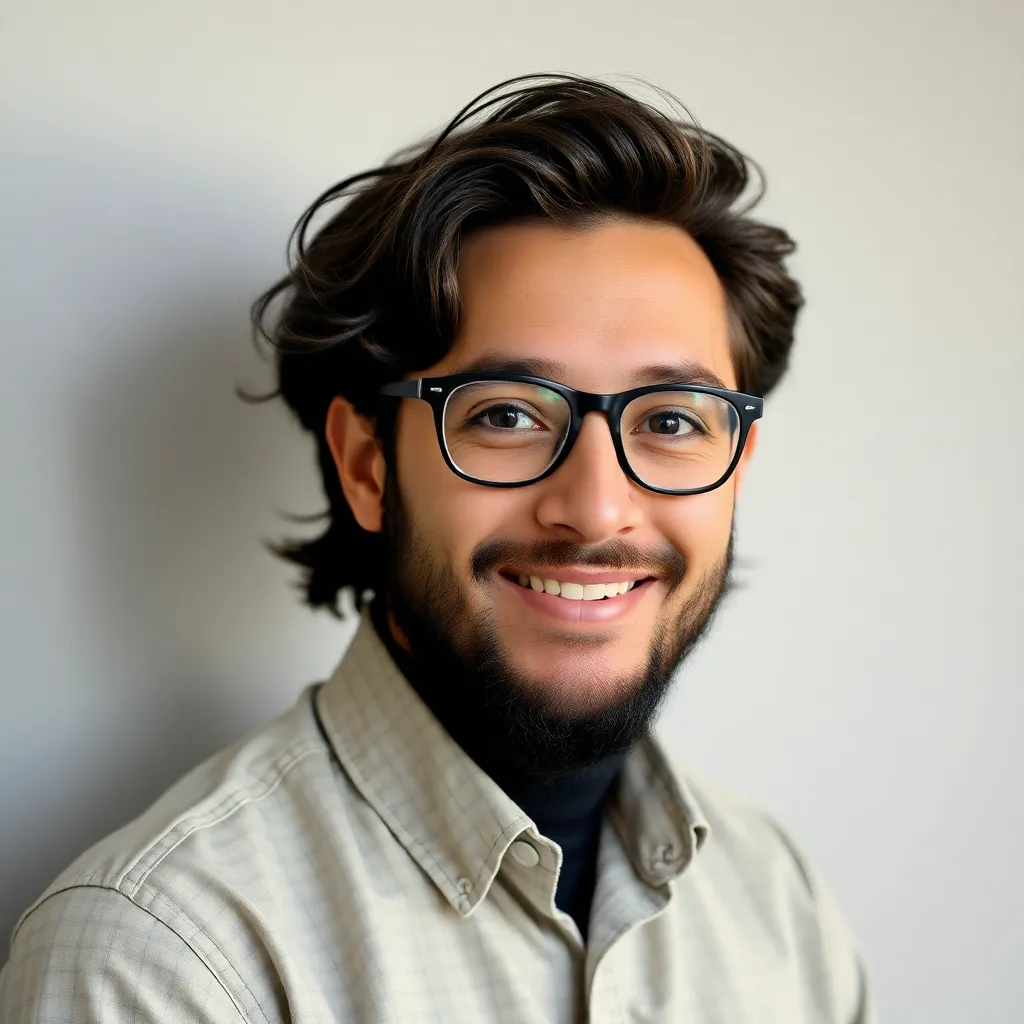
Muz Play
Apr 13, 2025 · 6 min read
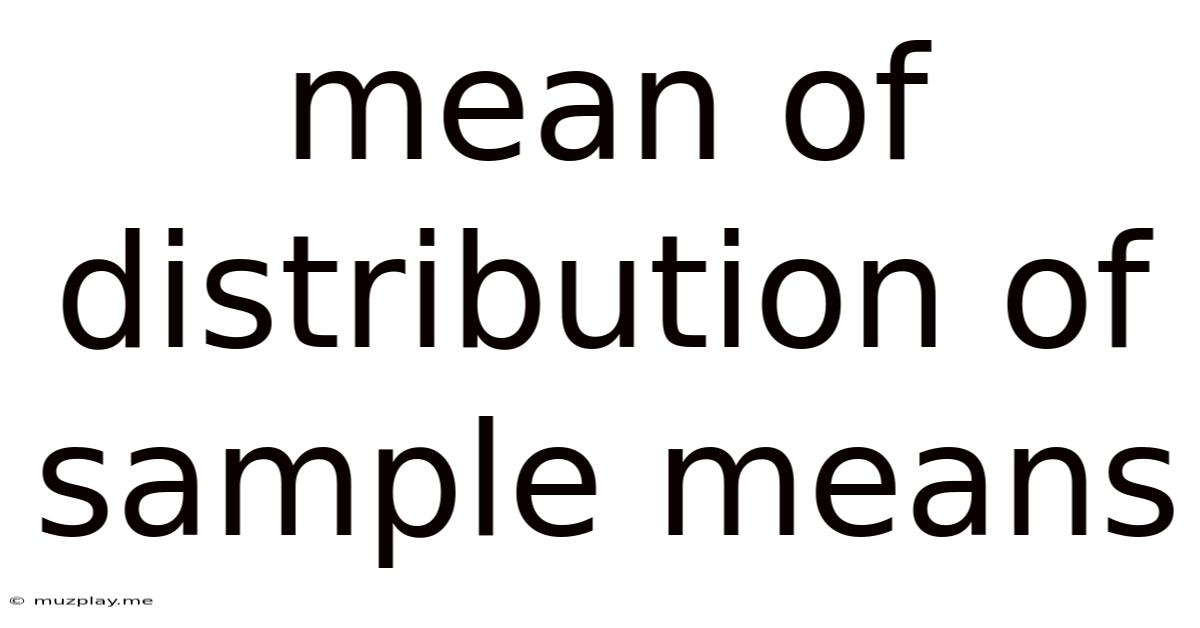
Table of Contents
Understanding the Mean of the Distribution of Sample Means
The mean of the distribution of sample means, often denoted as μ<sub>x̄</sub> (mu sub x-bar), is a fundamental concept in statistics. It represents the average of all possible sample means that could be obtained from a population. Grasping this concept is crucial for understanding statistical inference, hypothesis testing, and the central limit theorem. This article delves deep into the mean of the distribution of sample means, exploring its properties, applications, and significance in statistical analysis.
What is the Distribution of Sample Means?
Before understanding the mean of the distribution, let's clarify what the distribution of sample means actually is. Imagine you have a population – a complete set of data points. You could draw numerous random samples from this population, each sample containing a specific number of data points (the sample size, n). For each sample, you calculate the mean. The collection of all these sample means forms the distribution of sample means.
This distribution isn't just a random collection; it possesses specific characteristics that make it incredibly useful in statistical analysis. These characteristics are largely determined by the size of the samples and the characteristics of the original population.
Key Properties of the Distribution of Sample Means
- Central Tendency: The distribution of sample means tends to cluster around the population mean (μ). This is a key finding that underpins much of statistical inference.
- Spread (Variability): The spread of the distribution, measured by its standard deviation (standard error), is related to the population standard deviation (σ) and the sample size (n). Larger sample sizes generally lead to a smaller spread.
- Shape: As the sample size increases, the distribution of sample means increasingly resembles a normal distribution, regardless of the shape of the original population distribution. This is the essence of the central limit theorem.
The Mean of the Distribution of Sample Means (μ<sub>x̄</sub>)
The mean of this distribution, μ<sub>x̄</sub>, is simply the average of all the sample means. This might seem complex, but it has a remarkably simple relationship with the population mean:
μ<sub>x̄</sub> = μ
This equality holds true regardless of the sample size (n). The mean of the distribution of sample means is always equal to the population mean. This is a crucial fact, making the distribution of sample means a powerful tool for estimating population parameters.
Why is μ<sub>x̄</sub> = μ so important?
This equality forms the bedrock of many statistical techniques. It means that if we take many samples and calculate their means, the average of those means will be an unbiased estimator of the population mean. This allows us to make inferences about the population based on sample data, even if we don't have access to the entire population.
This principle is used extensively in:
- Confidence Intervals: Calculating confidence intervals for the population mean relies heavily on the knowledge that μ<sub>x̄</sub> = μ. The confidence interval provides a range of values within which the true population mean is likely to fall.
- Hypothesis Testing: Many hypothesis tests involve comparing a sample mean to a hypothesized population mean. The fact that μ<sub>x̄</sub> = μ provides a baseline against which to compare the sample mean.
Understanding the Standard Error
While the mean of the distribution of sample means is equal to the population mean, the standard deviation of the distribution, known as the standard error (SE), is different. The standard error indicates the variability or spread of the sample means around the population mean. A smaller standard error suggests that the sample means are clustered tightly around the population mean, indicating greater precision in estimating the population mean.
The formula for the standard error is:
SE = σ / √n
where:
- σ is the population standard deviation
- n is the sample size
This formula reveals several key insights:
- Influence of Population Standard Deviation: A larger population standard deviation leads to a larger standard error, meaning greater variability in sample means.
- Impact of Sample Size: Increasing the sample size (n) reduces the standard error, indicating that larger samples yield more precise estimates of the population mean. This is because larger samples provide more information about the population.
The Central Limit Theorem and its Relevance
The central limit theorem (CLT) is a cornerstone of statistical inference, and it directly relates to the distribution of sample means. The CLT states that, regardless of the shape of the population distribution, as the sample size (n) increases, the distribution of sample means approaches a normal distribution with a mean equal to the population mean (μ) and a standard deviation equal to the standard error (σ / √n).
This is incredibly powerful because it allows us to use the properties of the normal distribution to make inferences about the population mean, even if the population distribution isn't normal. The approximation to normality improves as the sample size increases; generally, a sample size of 30 or more is considered sufficient for a good approximation.
Implications of the Central Limit Theorem
- Normality Assumption: Many statistical tests assume that the data are normally distributed. The CLT allows us to relax this assumption when dealing with sample means, as the distribution of sample means tends toward normality even if the original data is not normally distributed.
- Simplified Calculations: The normal distribution has well-established properties and formulas, making calculations related to confidence intervals and hypothesis testing much simpler.
- Robustness of Statistical Tests: The CLT contributes to the robustness of many statistical tests, meaning that these tests remain relatively accurate even if the assumptions underlying them are slightly violated.
Practical Applications and Examples
The mean of the distribution of sample means has numerous applications across various fields:
- Quality Control: In manufacturing, the mean of the distribution of sample means helps monitor the average quality of products. By regularly sampling products and calculating the mean of the sample means, manufacturers can detect shifts in the average quality and take corrective actions.
- Public Health: Epidemiologists use the mean of the distribution of sample means to estimate the average prevalence of a disease in a population based on samples from different regions.
- Market Research: Market researchers employ this concept to estimate the average consumer preference for a product based on samples from different demographic groups.
- Environmental Science: Scientists use it to estimate the average concentration of a pollutant in a body of water based on samples collected at different locations.
Example:
Let's say a researcher wants to estimate the average height of adult women in a city. The researcher collects a random sample of 100 women and calculates their average height. They repeat this process many times, obtaining numerous sample means. The average of all these sample means will be an excellent estimate of the true average height of adult women in the city, and the standard error will tell the researcher how much the sample means vary.
Conclusion
The mean of the distribution of sample means, μ<sub>x̄</sub>, is a fundamental concept with far-reaching implications in statistics. Its equality to the population mean (μ) and the insights provided by the central limit theorem allow statisticians to make inferences about population parameters based on sample data. Understanding this concept is crucial for interpreting statistical results and making informed decisions in various fields. Mastering the mean of the distribution of sample means enhances your understanding of statistical inference and strengthens your analytical capabilities across numerous disciplines. From quality control to public health, the applications are vast and deeply impactful. As you delve further into the realm of statistics, remember the significance of this key concept and how it fundamentally shapes our approach to data analysis.
Latest Posts
Latest Posts
-
Real Life Example Of Double Replacement Reaction
May 09, 2025
-
Difference Between Strong Electrolyte And Weak Electrolyte
May 09, 2025
-
Diagramming A Cross Using A Punnett Square
May 09, 2025
-
Some Advantages Of The Intensification Of Globalization Include
May 09, 2025
-
Why Are Conifers And Cycads Considered Gymnosperms
May 09, 2025
Related Post
Thank you for visiting our website which covers about Mean Of Distribution Of Sample Means . We hope the information provided has been useful to you. Feel free to contact us if you have any questions or need further assistance. See you next time and don't miss to bookmark.