Mean Of The Sampling Distribution Of The Sample Mean
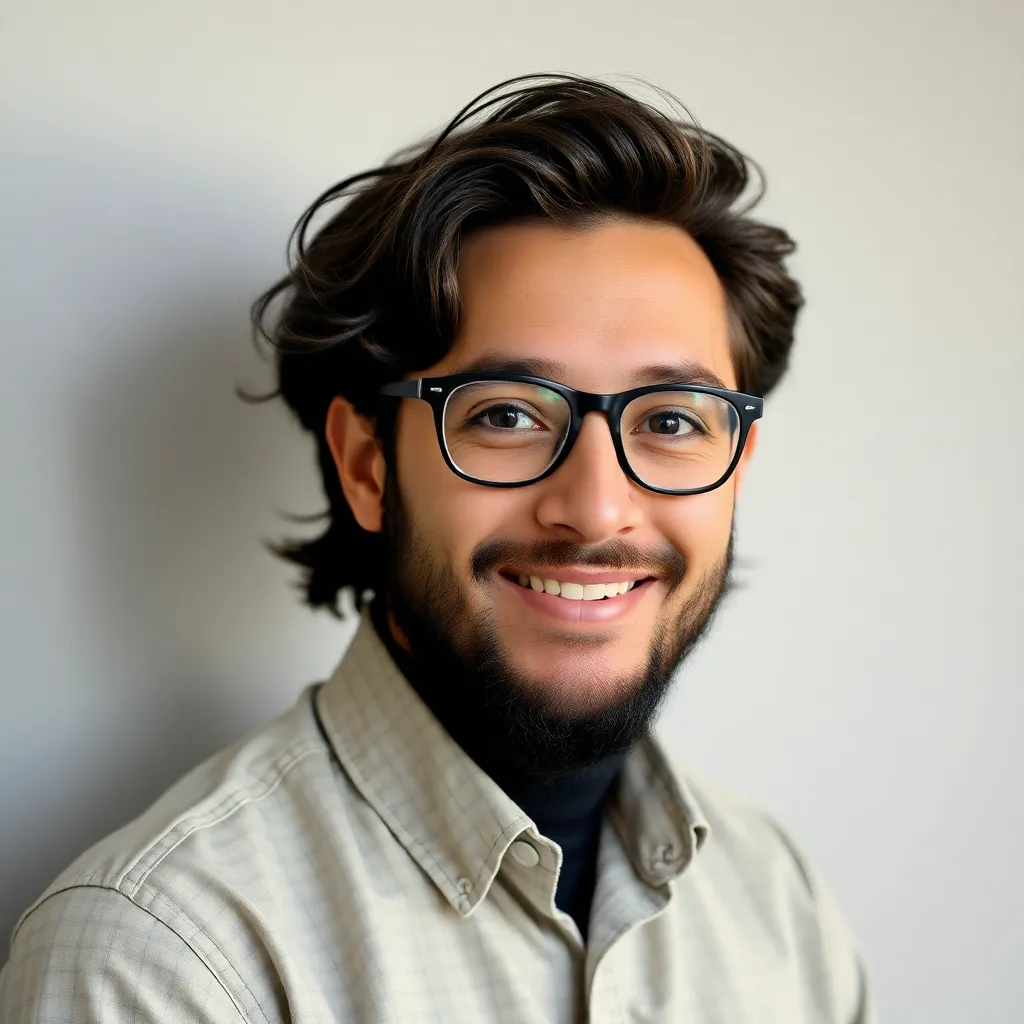
Muz Play
Apr 10, 2025 · 6 min read

Table of Contents
Understanding the Mean of the Sampling Distribution of the Sample Mean
The mean of the sampling distribution of the sample mean, often denoted as μ<sub>x̄</sub>, is a fundamental concept in statistics. It plays a crucial role in inferential statistics, allowing us to make inferences about a population based on sample data. This article provides a comprehensive explanation of this concept, exploring its properties, significance, and practical applications. We will delve into the underlying theory, illustrate it with examples, and highlight its importance in hypothesis testing and confidence intervals.
What is the Sampling Distribution of the Sample Mean?
Before understanding the mean of the sampling distribution, we must first grasp the concept of the sampling distribution itself. Imagine we have a population with a specific mean (μ) and standard deviation (σ). We then repeatedly draw random samples of a fixed size (n) from this population. For each sample, we calculate the sample mean (x̄). The collection of all these sample means forms the sampling distribution of the sample mean. This distribution, while seemingly abstract, is incredibly powerful because it provides a framework for understanding the behavior of sample means.
It's crucial to understand that the sampling distribution is not the population distribution. The population distribution describes the distribution of the individual data points in the entire population. The sampling distribution, on the other hand, describes the distribution of the means of multiple samples drawn from that population.
The Central Limit Theorem: A Cornerstone of Statistical Inference
The Central Limit Theorem (CLT) is a cornerstone of statistical inference, and it directly relates to the sampling distribution of the sample mean. The CLT states that, regardless of the shape of the population distribution, the sampling distribution of the sample mean will approximate a normal distribution as the sample size (n) increases. This is true even if the original population data isn't normally distributed. This remarkable property allows us to leverage the well-understood properties of the normal distribution to make inferences about the population mean.
The approximation to normality becomes increasingly accurate as the sample size grows larger. A common rule of thumb is that a sample size of at least 30 is sufficient for the CLT to hold reasonably well, particularly if the population distribution isn't too skewed. However, for smaller sample sizes or highly skewed populations, the approximation might not be as accurate.
The Mean of the Sampling Distribution: μ<sub>x̄</sub> = μ
This is where the key concept comes in. The mean of the sampling distribution of the sample mean (μ<sub>x̄</sub>) is equal to the population mean (μ). This is a powerful and essential result. In mathematical terms: μ<sub>x̄</sub> = μ
This means that, on average, the sample means will center around the true population mean. If we were to take countless samples and calculate their means, the average of all those sample means would be the true population mean. This equality forms the basis of many statistical procedures used to estimate population parameters.
Why is μ<sub>x̄</sub> = μ Important?
The equality of μ<sub>x̄</sub> and μ has profound implications for statistical inference:
-
Unbiased Estimation: The sample mean (x̄) is an unbiased estimator of the population mean (μ). This means that the average of all possible sample means will equal the true population mean. There is no systematic overestimation or underestimation.
-
Foundation for Confidence Intervals: Confidence intervals, which provide a range of values likely to contain the population mean, rely heavily on the properties of the sampling distribution, specifically its mean. The fact that μ<sub>x̄</sub> = μ ensures that the confidence interval is centered around the true population mean.
-
Hypothesis Testing: Many hypothesis tests involve comparing a sample mean to a hypothesized population mean. The understanding of the sampling distribution, and its mean being equal to the population mean, allows us to determine the probability of observing a sample mean as extreme as the one obtained if the null hypothesis (a statement about the population mean) were true.
Standard Error of the Mean: A Measure of Variability
While the mean of the sampling distribution is equal to the population mean, the variability of the sampling distribution is different from the population variability. This variability is measured by the standard error of the mean (SEM), which is calculated as:
SEM = σ / √n
where:
- σ is the population standard deviation
- n is the sample size
Notice that the standard error is inversely proportional to the square root of the sample size. This means that as the sample size increases, the standard error decreases. This makes intuitive sense: larger samples tend to provide more precise estimates of the population mean, resulting in a smaller spread in the sampling distribution.
Practical Examples
Let's illustrate these concepts with examples:
Example 1: Heights of Adults
Suppose we want to estimate the average height of adult women in a country. The true population mean height (μ) and standard deviation (σ) are unknown. We collect a random sample of 100 women and calculate the sample mean height (x̄). According to the CLT, the sampling distribution of the sample mean will be approximately normal, with a mean (μ<sub>x̄</sub>) equal to the true population mean height and a standard error equal to σ/√100 = σ/10.
Example 2: Exam Scores
Consider a professor wanting to estimate the average score on a final exam. The population of exam scores has a certain mean (μ) and standard deviation (σ). The professor takes several random samples of 30 students each and calculates the mean score for each sample. The average of these sample means (μ<sub>x̄</sub>) will be approximately equal to the true population mean score, and the standard error will be σ/√30.
Implications for Sample Size and Precision
The standard error of the mean highlights the importance of sample size in statistical inference. A larger sample size leads to a smaller standard error, resulting in a more precise estimate of the population mean. The sampling distribution becomes narrower, concentrating the sample means more closely around the true population mean. This directly impacts the width of confidence intervals and the power of hypothesis tests – larger samples yield narrower confidence intervals and more powerful tests.
Dealing with Unknown Population Standard Deviation
In many real-world scenarios, the population standard deviation (σ) is unknown. In such cases, we estimate σ using the sample standard deviation (s). The standard error is then estimated as:
SEM ≈ s / √n
The use of the sample standard deviation introduces some additional uncertainty, but the principle that μ<sub>x̄</sub> = μ remains unchanged. The sampling distribution is now approximated by a t-distribution rather than a normal distribution, especially for smaller sample sizes. The t-distribution accounts for the additional uncertainty due to the estimation of the population standard deviation.
Conclusion
The mean of the sampling distribution of the sample mean is a cornerstone of statistical inference. The understanding that μ<sub>x̄</sub> = μ allows us to make reliable inferences about population means based on sample data. This principle, underpinned by the Central Limit Theorem, is crucial for constructing confidence intervals, performing hypothesis tests, and understanding the precision of our estimates. The standard error of the mean further quantifies the variability of the sampling distribution, illustrating the relationship between sample size and the precision of our estimations. Mastering this concept is essential for anyone working with statistical data analysis and inference. Further exploration of related topics like confidence intervals, hypothesis testing, and the t-distribution will solidify this foundational understanding and empower you to make informed statistical decisions.
Latest Posts
Latest Posts
-
Is A Fat Or Phospholipid Less Soluble In Water
Apr 18, 2025
-
What Is The Total Magnification Of The Low Power Objective
Apr 18, 2025
-
Bacteriocins And Defensins Are Types Of Which Of The Following
Apr 18, 2025
-
An Ion Can Be Formed By
Apr 18, 2025
-
Displacement Is How Far An Object Moves
Apr 18, 2025
Related Post
Thank you for visiting our website which covers about Mean Of The Sampling Distribution Of The Sample Mean . We hope the information provided has been useful to you. Feel free to contact us if you have any questions or need further assistance. See you next time and don't miss to bookmark.