Multiple Regression Approach To Experimental Design
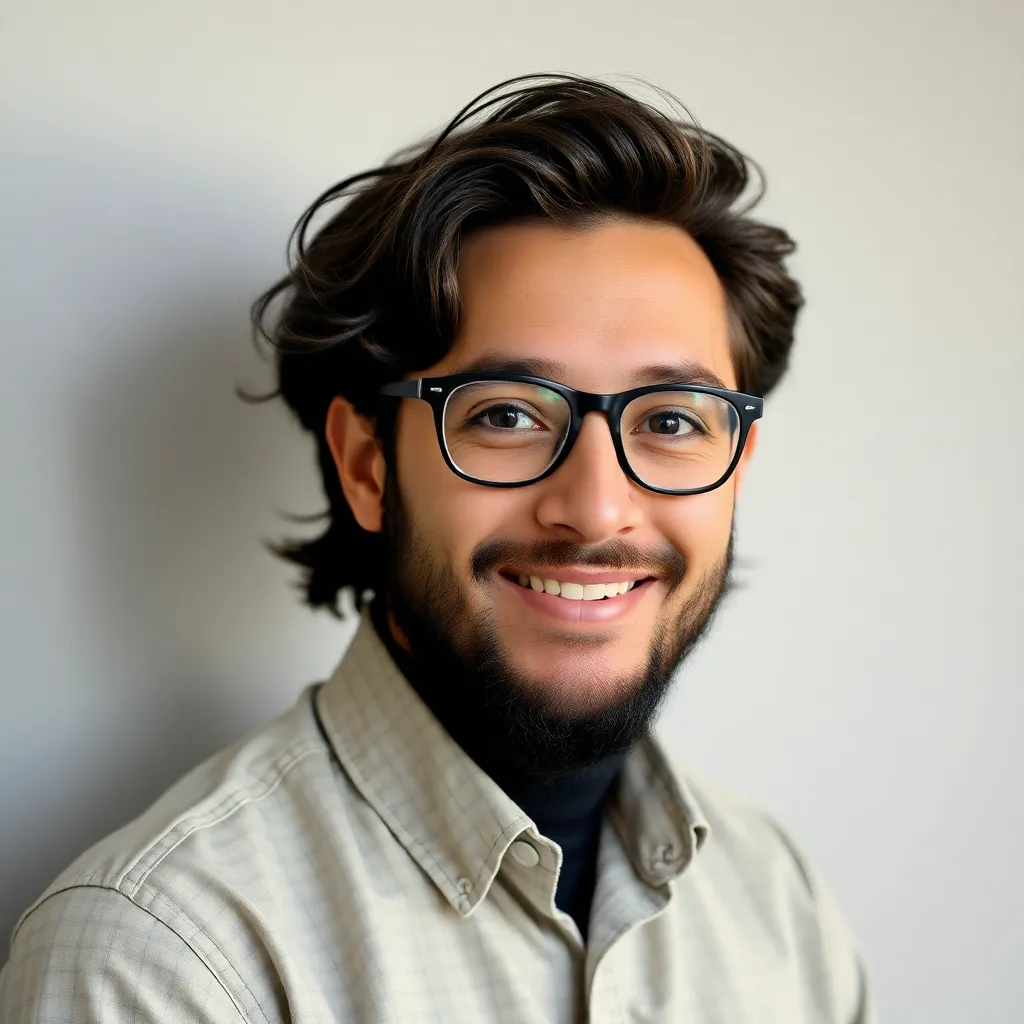
Muz Play
May 12, 2025 · 7 min read
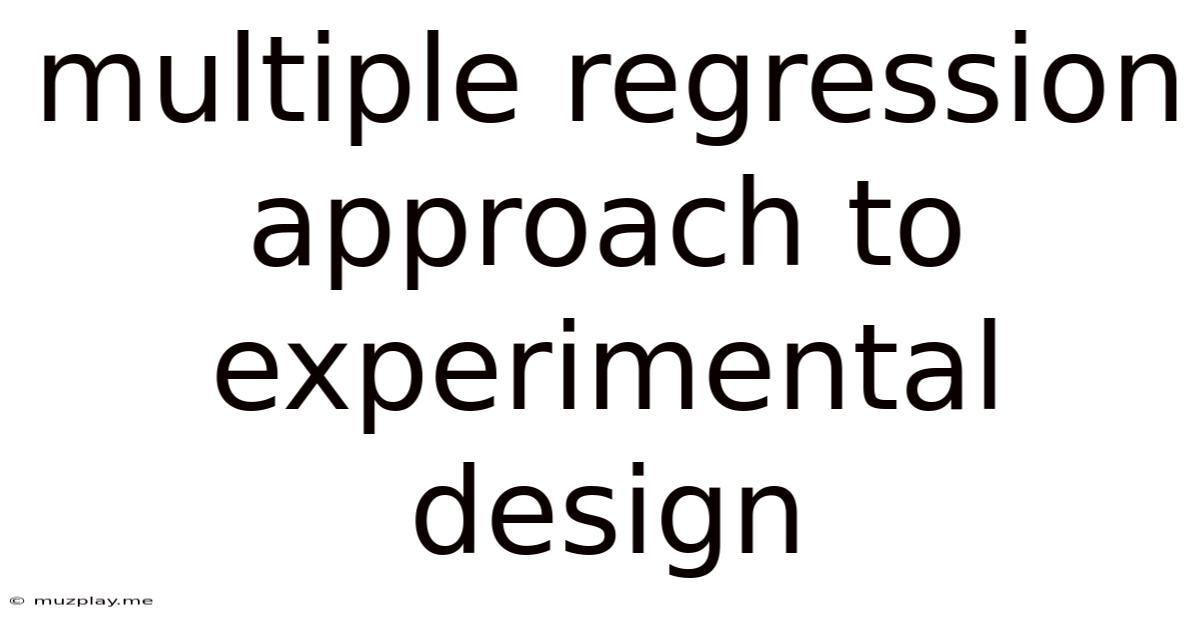
Table of Contents
Multiple Regression Approach to Experimental Design
Multiple regression analysis is a powerful statistical technique used extensively in experimental design to model the relationship between a dependent variable and multiple independent variables. This approach allows researchers to understand not only the individual effects of each independent variable but also their combined and interactive influences on the outcome. This article delves into the multiple regression approach in experimental design, exploring its applications, assumptions, interpretations, and limitations. We'll cover everything from designing your experiment to interpreting the resulting regression model.
Understanding the Fundamentals
Before diving into the specifics of multiple regression in experimental design, let's briefly review the core concepts.
Dependent and Independent Variables
-
Dependent Variable (Y): This is the variable you are trying to predict or explain. It's the outcome of your experiment, and its value depends on the independent variables. For example, if you're studying the effect of fertilizer on crop yield, crop yield is the dependent variable.
-
Independent Variables (X1, X2, X3...): These are the variables you manipulate or control in your experiment. They are believed to influence the dependent variable. In our fertilizer example, the type of fertilizer, the amount of fertilizer, and the watering frequency could all be independent variables.
The Multiple Regression Model
The multiple regression model expresses the relationship between the dependent and independent variables mathematically:
Y = β0 + β1X1 + β2X2 + β3X3 + ... + βnXn + ε
Where:
- Y is the dependent variable.
- β0 is the intercept (the value of Y when all X's are zero).
- β1, β2, β3...βn are the regression coefficients, representing the change in Y for a one-unit change in the corresponding X, holding all other X's constant.
- X1, X2, X3...Xn are the independent variables.
- ε is the error term, representing the unexplained variation in Y.
This equation essentially states that the value of Y is a linear combination of the independent variables, plus some random error. The goal of multiple regression is to estimate the values of the β coefficients, which tell us the strength and direction of the relationship between each independent variable and the dependent variable.
Designing Experiments for Multiple Regression
Proper experimental design is crucial for obtaining meaningful results from multiple regression analysis. Key considerations include:
Sample Size
A sufficiently large sample size is necessary to ensure the reliability and statistical power of your analysis. The required sample size depends on several factors, including the number of independent variables, the expected effect size, and the desired level of significance. Power analysis can help determine the appropriate sample size.
Randomization
Randomly assigning subjects or experimental units to different treatment groups helps minimize bias and ensure that the results are generalizable to the broader population. Randomization helps to control for confounding variables—variables that are not explicitly included in the model but could influence the dependent variable.
Control Variables
Incorporating control variables helps to account for the effects of extraneous factors that could influence the dependent variable. These variables are not of primary interest but are included in the model to reduce the error variance and improve the precision of the estimates of the regression coefficients.
Factorial Designs
Factorial designs are particularly useful when studying the effects of multiple independent variables and their interactions. In a factorial design, all possible combinations of the independent variables are included in the experiment. This allows researchers to assess both the main effects of each independent variable and the interaction effects between them. For instance, if you're testing two fertilizer types (A and B) and two watering frequencies (high and low), a 2x2 factorial design would involve four treatment groups: A-high, A-low, B-high, and B-low.
Blocking
Blocking is a technique used to reduce the impact of nuisance variables that are known to affect the response variable but are not of primary interest. By grouping similar experimental units into blocks, we can control for the variation introduced by these nuisance variables. For example, if you are studying the yield of different crops, blocking by soil type could help account for the differences in soil fertility.
Assumptions of Multiple Regression
Multiple regression analysis relies on several key assumptions. Violation of these assumptions can lead to biased or unreliable results. These assumptions include:
Linearity
The relationship between the dependent variable and each independent variable should be approximately linear. Scatter plots can be used to visually assess linearity. Transformations of the variables (e.g., logarithmic or square root transformations) can sometimes be used to address non-linearity.
Independence of Errors
The errors (residuals) should be independent of each other. Autocorrelation, where errors are correlated over time or space, can violate this assumption. This is particularly important in time series data.
Homoscedasticity
The variance of the errors should be constant across all levels of the independent variables. Heteroscedasticity, where the variance of the errors varies across different levels, can lead to inefficient and biased estimates.
Normality of Errors
The errors should be normally distributed. While mild deviations from normality are often acceptable, severe departures can affect the validity of hypothesis tests.
No Multicollinearity
Multicollinearity refers to a high correlation between two or more independent variables. This can make it difficult to isolate the individual effects of each variable and can lead to unstable regression coefficients.
Interpreting the Results
After performing multiple regression analysis, careful interpretation of the results is crucial. Key elements to consider include:
Regression Coefficients (β)
The regression coefficients indicate the change in the dependent variable associated with a one-unit change in the corresponding independent variable, holding all other independent variables constant. The sign of the coefficient indicates the direction of the relationship (positive or negative), and the magnitude indicates the strength of the relationship.
p-values
The p-value associated with each regression coefficient indicates the statistical significance of the relationship between that independent variable and the dependent variable. A low p-value (typically less than 0.05) suggests that the relationship is statistically significant.
R-squared (R²)
R-squared represents the proportion of variance in the dependent variable that is explained by the independent variables in the model. A higher R-squared indicates a better fit of the model. However, it's crucial to remember that a high R-squared doesn't necessarily imply a good model; it's just one aspect to consider. Adjusted R-squared considers the number of independent variables in the model, and provides a more reliable measure of model fit, especially when comparing models with different numbers of predictors.
Model Fit
Assessing the overall fit of the model involves examining various statistics, including R-squared, adjusted R-squared, and residual plots. Residual plots can reveal potential violations of the regression assumptions, such as non-linearity or heteroscedasticity.
Interaction Effects
In factorial designs, interpreting interaction effects is crucial. An interaction effect occurs when the effect of one independent variable on the dependent variable differs depending on the level of another independent variable. This means the combined effect of the two variables is not simply additive.
Limitations of Multiple Regression
Despite its power, multiple regression has limitations:
-
Assumption Violations: As discussed earlier, violations of the regression assumptions can lead to biased or unreliable results.
-
Causation vs. Correlation: Multiple regression can demonstrate correlations between variables, but it cannot definitively prove causation. Other factors not included in the model could be influencing the relationships observed.
-
Extrapolation: It's risky to extrapolate beyond the range of values of the independent variables used in the analysis. The model may not accurately predict outcomes outside this range.
-
Multicollinearity: As already mentioned, high multicollinearity among independent variables can severely hinder the interpretation and reliability of the results.
Conclusion
Multiple regression analysis is a valuable tool in experimental design, allowing researchers to model the complex relationships between a dependent variable and multiple independent variables. However, careful attention to experimental design, assumption checking, and interpretation is critical to ensure that the results are meaningful and reliable. Understanding both the strengths and limitations of multiple regression is essential for conducting robust and insightful research. Remember to always critically evaluate your findings and consider alternative explanations before drawing definitive conclusions. The careful application of multiple regression, combined with a thorough understanding of its underlying principles and limitations, allows for powerful insights into complex experimental data. This method empowers researchers to make informed decisions and contribute valuable knowledge to their respective fields.
Latest Posts
Related Post
Thank you for visiting our website which covers about Multiple Regression Approach To Experimental Design . We hope the information provided has been useful to you. Feel free to contact us if you have any questions or need further assistance. See you next time and don't miss to bookmark.