Nonequivalent Control Group Interrupted Time-series Design
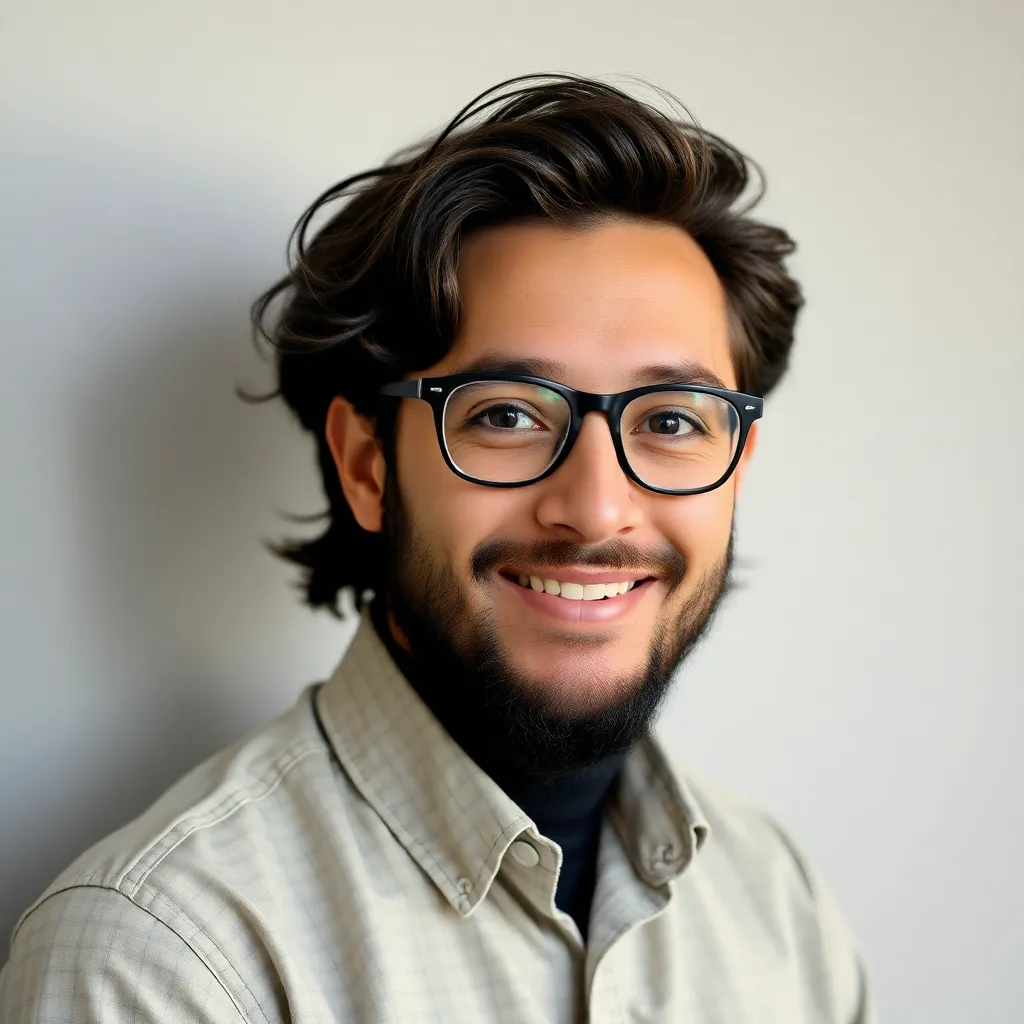
Muz Play
May 11, 2025 · 6 min read
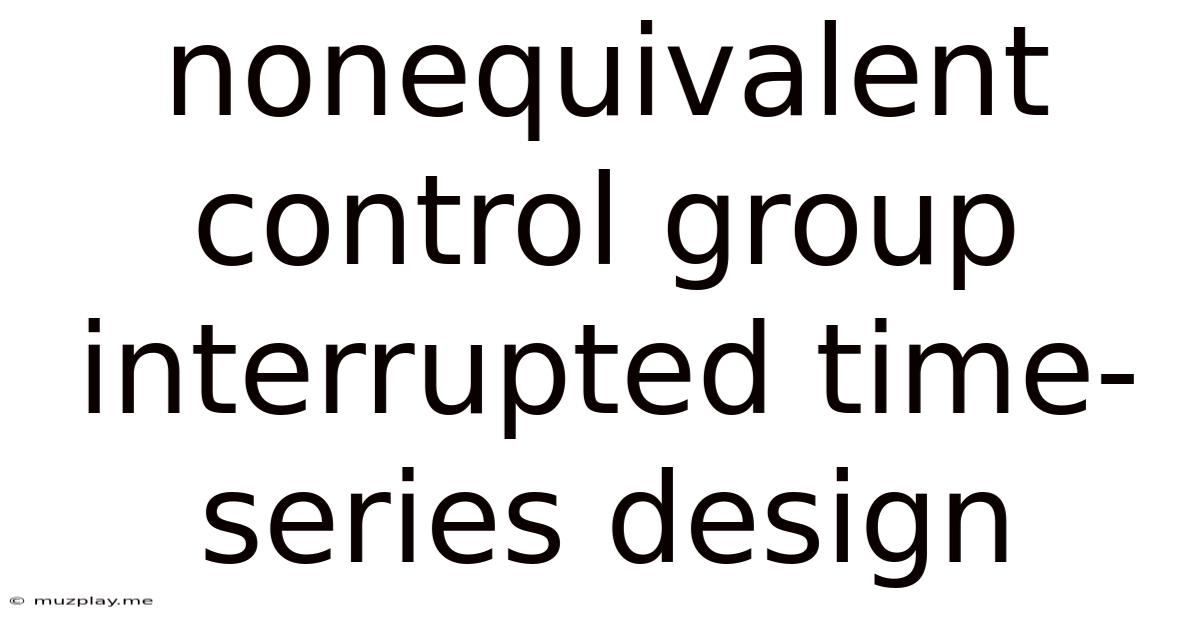
Table of Contents
Nonequivalent Control Group Interrupted Time-Series Design: A Deep Dive
The nonequivalent control group interrupted time-series design is a powerful quasi-experimental research design used to evaluate the impact of an intervention or treatment. It combines elements of both interrupted time-series designs and control group designs, offering a robust approach to assessing causal relationships when true randomization isn't feasible. This article will explore this design in detail, covering its strengths, weaknesses, and practical applications.
Understanding the Components
This design hinges on two key components:
1. Interrupted Time-Series Design:
This involves collecting data on an outcome variable over time, both before and after an intervention is implemented. The intervention acts as an "interrupt" to the pre-existing trend in the data. By analyzing changes in the trend around the intervention point, researchers can assess its potential effect. The data itself forms a time series – a sequence of observations taken over time.
2. Nonequivalent Control Group:
A control group, not subjected to the intervention, is also included. Crucially, this group isn't randomly assigned; hence, the "nonequivalent" descriptor. The control group serves as a comparison point, allowing researchers to distinguish between changes in the treatment group that are genuinely due to the intervention and changes that might be due to extraneous factors (e.g., seasonal variations, maturation).
How it Works: A Step-by-Step Guide
-
Identify the Intervention: Clearly define the intervention or treatment you're evaluating. This needs to be a clearly delineated event with a specific start and (potentially) end date.
-
Select Treatment and Control Groups: Choose a treatment group that will receive the intervention and a comparable control group that will not. Careful consideration is crucial here; the groups should be as similar as possible regarding relevant characteristics, minimizing pre-existing differences that could confound the results. The more similar the groups, the stronger the causal inference.
-
Data Collection: Collect data on the outcome variable of interest at multiple time points before and after the intervention in both the treatment and control groups. The more data points, the better the power of the analysis to detect effects. Consistent data collection methods are critical to maintain internal validity.
-
Data Analysis: Statistical analysis is essential to discern the intervention's impact. Visual inspection of the time series plots can be informative, but rigorous statistical methods are necessary to quantify the effect and account for potential confounding factors. Common techniques include:
-
Visual Inspection: Plotting the time series data for both groups allows for a visual assessment of changes in trends before and after the intervention. A clear shift in the treatment group's trend, absent in the control group, suggests a potential causal effect.
-
Regression Discontinuity Design (RDD): RDD is particularly useful when the intervention is assigned based on a cutoff score. It analyzes the discontinuity in the outcome around the cutoff to estimate the causal effect.
-
Segmented Regression: This method models the time series data with separate regression lines before and after the intervention, assessing the change in slope (the magnitude of the intervention effect).
-
Time Series Analysis Techniques (ARIMA): Autoregressive integrated moving average (ARIMA) models can account for autocorrelation (the dependence of observations over time) in the data, providing a more accurate estimate of the intervention's impact.
-
Strengths of the Nonequivalent Control Group Interrupted Time-Series Design
-
Stronger Causal Inference: Compared to a single interrupted time-series design, the inclusion of a nonequivalent control group strengthens the causal inference by helping to rule out alternative explanations for observed changes in the treatment group. It controls for history, maturation, and other secular trends.
-
Practical Applicability: This design is often more feasible than randomized controlled trials (RCTs) in real-world settings, as it doesn't require random assignment. This is especially important when ethical considerations or logistical constraints preclude randomization.
-
Longitudinal Data: The design leverages longitudinal data, providing a rich understanding of the intervention's impact over time. It captures both immediate and longer-term effects.
Weaknesses of the Nonequivalent Control Group Interrupted Time-Series Design
-
Selection Bias: The lack of random assignment introduces the risk of selection bias. Pre-existing differences between the treatment and control groups could confound the results, making it difficult to isolate the true effect of the intervention. Careful selection of the control group and statistical adjustments are crucial to minimize this bias.
-
Threats to Internal Validity: Other threats to internal validity, such as history (external events influencing the outcome), maturation (natural changes over time), and instrumentation (changes in measurement), still need to be considered and addressed. Careful study design and data analysis techniques are crucial.
-
Statistical Complexity: The analysis of this design can be statistically complex, requiring expertise in time series analysis and regression techniques. Incorrect analysis can lead to inaccurate conclusions.
Addressing Limitations and Enhancing Validity
Several strategies can mitigate the weaknesses of this design:
-
Careful Group Matching: Employ techniques to match the treatment and control groups as closely as possible on key variables that might influence the outcome. Propensity score matching is a valuable tool in this regard.
-
Multiple Time Points: Collecting data at many time points before and after the intervention enhances the statistical power and allows for a more robust assessment of trends.
-
Control for Confounders: Incorporate relevant covariates into the statistical analysis to control for confounding variables and improve the precision of the effect estimate.
-
Robust Statistical Methods: Utilize advanced statistical methods, such as ARIMA modeling and segmented regression, to account for autocorrelation and other complexities in the time series data.
-
Triangulation: Combine this design with other data collection methods (qualitative data, surveys) to strengthen the validity of the findings.
Examples of Applications
This design finds utility across diverse fields:
-
Public Health: Evaluating the impact of a new public health intervention on disease rates.
-
Education: Assessing the effectiveness of a new educational program on student achievement.
-
Social Work: Examining the effects of a social work intervention on client outcomes.
-
Criminal Justice: Evaluating the impact of a crime prevention program on crime rates.
-
Environmental Science: Assessing the effects of an environmental policy on pollution levels.
Conclusion
The nonequivalent control group interrupted time-series design offers a valuable approach for evaluating interventions in situations where randomization isn't feasible. While it presents certain challenges, careful planning, rigorous data collection, and appropriate statistical analysis can yield strong evidence regarding an intervention's effectiveness. Understanding its strengths, weaknesses, and the methods for enhancing its validity is crucial for researchers seeking to use this powerful quasi-experimental design. By carefully considering the potential limitations and employing appropriate statistical techniques, researchers can maximize the contribution of this design to generating robust and meaningful research findings. Remember, meticulous attention to detail throughout the research process is paramount to drawing reliable conclusions from this complex yet rewarding approach. This design, while not perfect, offers a robust alternative when ethical or practical considerations preclude the use of randomized controlled trials.
Latest Posts
Latest Posts
-
According To The Second Formulation Of The Categorical Imperative
May 12, 2025
-
When Sodium Chloride Dissolves In Water
May 12, 2025
-
Study Of Matter And The Changes It Undergoes
May 12, 2025
-
Describe Three Uses Of Radioactive Isotopes
May 12, 2025
-
Can Neutralize A Base Physical Or Chemical
May 12, 2025
Related Post
Thank you for visiting our website which covers about Nonequivalent Control Group Interrupted Time-series Design . We hope the information provided has been useful to you. Feel free to contact us if you have any questions or need further assistance. See you next time and don't miss to bookmark.