One Sample Z Interval For A Population Proportion
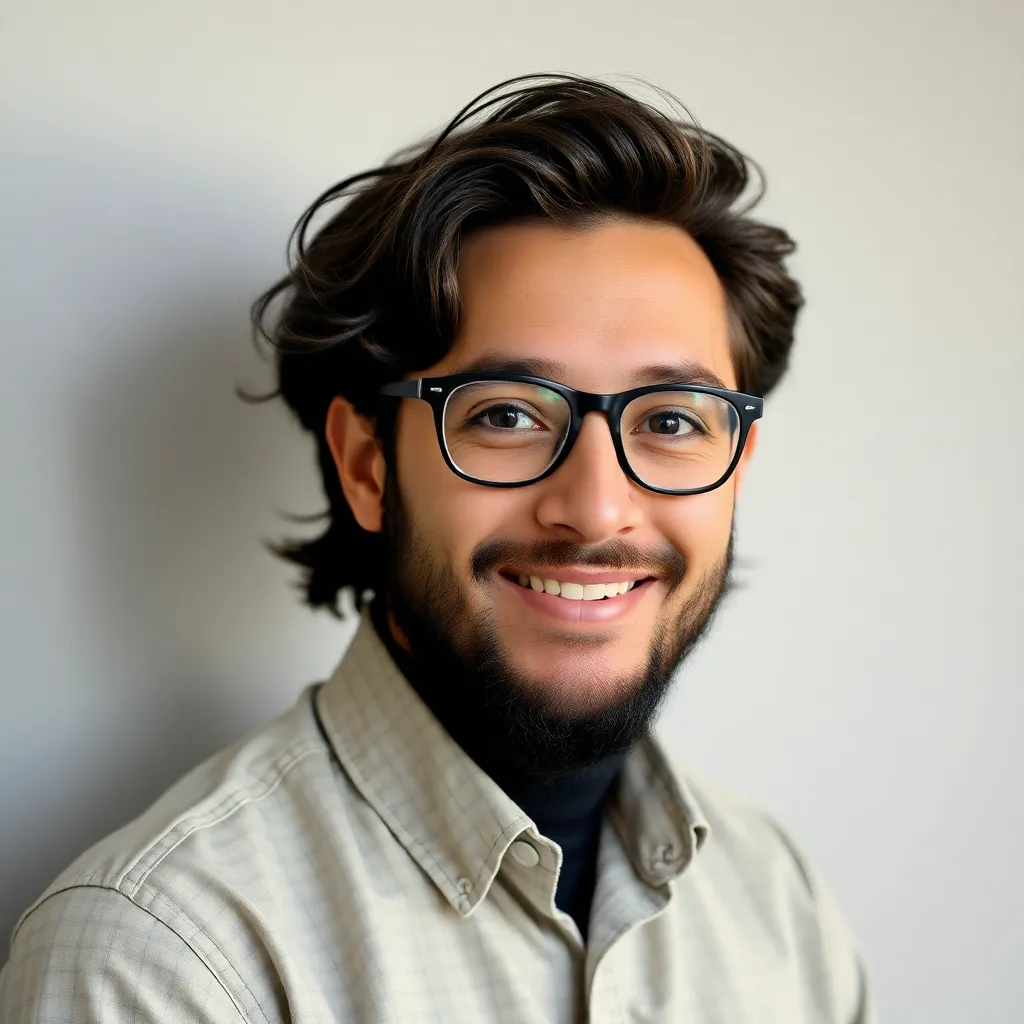
Muz Play
Apr 05, 2025 · 6 min read
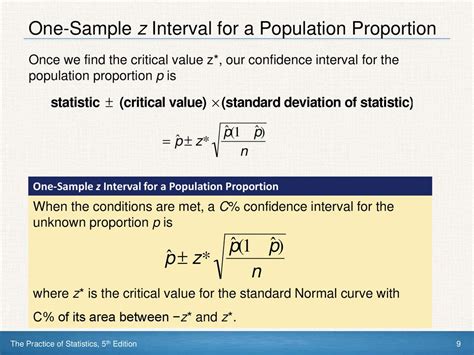
Table of Contents
One-Sample Z-Interval for a Population Proportion: A Comprehensive Guide
Understanding and applying statistical inference is crucial for drawing meaningful conclusions from data. One common inferential procedure is constructing a confidence interval, which provides a range of plausible values for a population parameter. This article delves into the one-sample z-interval for a population proportion, explaining its underlying principles, assumptions, calculations, and interpretations. We will also explore common pitfalls and offer practical advice for its effective application.
Understanding Population Proportions and Sampling
Before diving into the specifics of the z-interval, let's clarify some fundamental concepts. A population proportion, denoted as p, represents the percentage of individuals in a population who possess a specific characteristic or attribute. For example, p could represent the proportion of voters who favor a particular candidate, the proportion of defective items in a manufacturing batch, or the proportion of students who pass a specific exam.
In most cases, it's impractical or impossible to examine the entire population. Therefore, we rely on sampling – selecting a representative subset of the population – to estimate the population proportion. The sample proportion, denoted as p̂ (p-hat), is the proportion of individuals in the sample possessing the characteristic of interest. p̂ serves as our point estimate for p.
The Central Limit Theorem and the Z-Interval
The foundation of the one-sample z-interval lies in the Central Limit Theorem (CLT). The CLT states that the sampling distribution of the sample proportion p̂ approximates a normal distribution, provided certain conditions are met. Specifically:
- Random Sampling: The sample must be selected randomly from the population to ensure representativeness.
- Independence: The sample size should be less than 10% of the population size (to ensure independence between observations).
- Success-Failure Condition: Both np ≥ 10 and n(1-p) ≥ 10 (where n is the sample size). This condition ensures that the sampling distribution is approximately normal. Since we're estimating p, we replace p with p̂ in practice.
When these conditions are met, we can utilize the z-distribution to construct a confidence interval for the population proportion.
Calculating the One-Sample Z-Interval
The formula for the one-sample z-interval for a population proportion is:
p̂ ± z√(p̂(1-p̂)/n)*
Where:
- p̂: is the sample proportion.
- z:* is the critical z-value corresponding to the desired confidence level (e.g., 1.96 for a 95% confidence interval).
- n: is the sample size.
Let's break down the calculation steps with an example:
Example: Suppose a researcher wants to estimate the proportion of adults in a city who support a new park initiative. A random sample of 400 adults is surveyed, and 240 express support. Calculate a 95% confidence interval for the population proportion.
-
Calculate the sample proportion (p̂): p̂ = 240/400 = 0.6
-
Determine the critical z-value (z):* For a 95% confidence interval, z* = 1.96.
-
Calculate the standard error: √(p̂(1-p̂)/n) = √(0.6 * 0.4 / 400) ≈ 0.0245
-
Calculate the margin of error: z* * standard error = 1.96 * 0.0245 ≈ 0.048
-
Calculate the confidence interval: p̂ ± margin of error = 0.6 ± 0.048 = (0.552, 0.648)
Therefore, we are 95% confident that the true population proportion of adults who support the park initiative lies between 55.2% and 64.8%.
Interpreting the Confidence Interval
The confidence interval provides a range of plausible values for the population proportion. The confidence level (e.g., 95%) represents the long-run probability that a randomly selected confidence interval will contain the true population proportion. It's crucial to understand that the confidence interval does not mean there's a 95% chance that the true proportion falls within the calculated interval. The true proportion is either within the interval or it isn't; the confidence level refers to the reliability of the procedure used to construct the interval.
Assumptions and Limitations
The accuracy and validity of the z-interval depend heavily on the assumptions mentioned earlier. Violations of these assumptions can lead to unreliable results. For instance:
- Non-random sampling: If the sample is not representative of the population (e.g., biased sampling), the confidence interval will be inaccurate.
- Non-independence: If observations are not independent, the standard error will be underestimated, leading to a narrower and potentially misleading confidence interval.
- Small sample size: If the success-failure condition is not met, the normal approximation might not be accurate, and alternative methods (like the Clopper-Pearson interval) might be more appropriate.
Choosing the Right Confidence Level
The choice of confidence level often depends on the context of the study and the desired level of precision. Higher confidence levels (e.g., 99%) yield wider intervals, reflecting greater certainty but reduced precision. Conversely, lower confidence levels (e.g., 90%) result in narrower intervals with increased precision but decreased certainty. A 95% confidence level is commonly used as a balance between precision and confidence.
Sample Size Determination
The width of the confidence interval is directly influenced by the sample size. Larger sample sizes generally lead to narrower intervals, providing more precise estimates of the population proportion. Determining the appropriate sample size before conducting a study is crucial. The required sample size can be calculated using formulas that incorporate the desired margin of error, confidence level, and an estimate of the population proportion (or a conservative estimate of 0.5 if no prior information is available).
Comparing Confidence Intervals
Confidence intervals can be compared to assess the differences between population proportions. If two confidence intervals for different groups do not overlap significantly, it suggests a statistically significant difference between the population proportions. However, this comparison is only a rough assessment; formal hypothesis testing provides more rigorous conclusions about group differences.
Advanced Considerations
While the z-interval provides a straightforward method for estimating population proportions, it's important to be aware of its limitations. In situations with very small sample sizes or when the success-failure condition is violated, alternative methods, such as the Wilson score interval or Clopper-Pearson interval, might offer better performance. These methods are more robust to violations of the normality assumption. Furthermore, for complex sampling designs (e.g., stratified sampling, cluster sampling), adjustments to the standard error might be necessary to account for the sampling design's characteristics.
Conclusion
The one-sample z-interval is a valuable statistical tool for estimating population proportions. Its simplicity and wide applicability make it a staple in various fields. However, understanding the underlying assumptions, correctly interpreting the results, and being aware of potential limitations are crucial for its effective use. By carefully considering these aspects, researchers can draw more accurate and reliable inferences about population proportions from sample data. Always remember to report the confidence interval alongside the point estimate and appropriately contextualize the findings within the study's limitations. This comprehensive approach enhances the integrity and reliability of statistical analyses and supports informed decision-making.
Latest Posts
Latest Posts
-
A Colored Ion Generally Indicates A
Apr 06, 2025
-
Why Does Boron Have A Lower Ionization Energy Than Beryllium
Apr 06, 2025
-
Multiplying And Dividing Significant Figures Practice
Apr 06, 2025
-
Which Of The Following Accessory Organs Stores Bile
Apr 06, 2025
-
A Nonpolar Covalent Bond Is One In Which
Apr 06, 2025
Related Post
Thank you for visiting our website which covers about One Sample Z Interval For A Population Proportion . We hope the information provided has been useful to you. Feel free to contact us if you have any questions or need further assistance. See you next time and don't miss to bookmark.