Point Estimate Of Population Standard Deviation
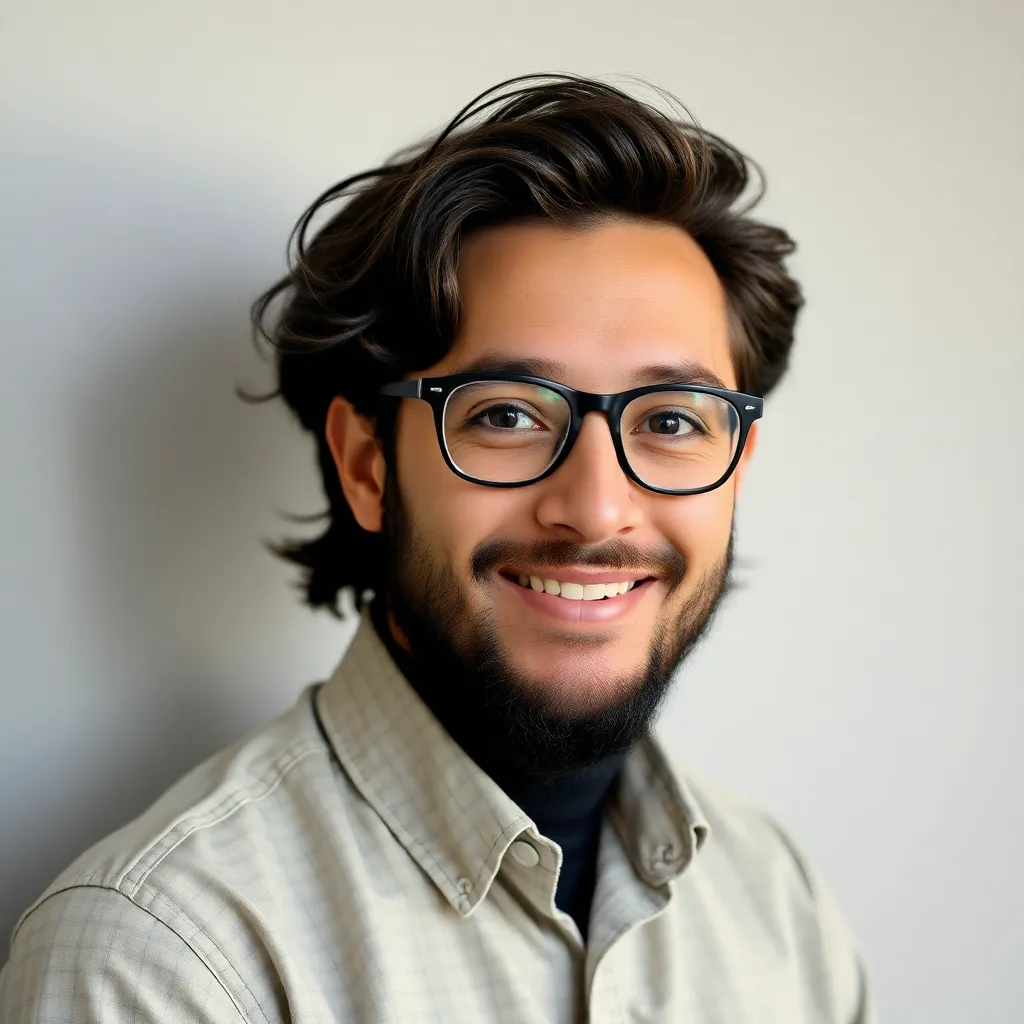
Muz Play
Mar 11, 2025 · 6 min read

Table of Contents
Point Estimate of Population Standard Deviation: A Comprehensive Guide
The population standard deviation, denoted by σ (sigma), is a crucial parameter in statistics, representing the dispersion or spread of data within a population. However, obtaining the true population standard deviation is often impractical, if not impossible, due to the cost and time involved in surveying every member of a large population. This is where the concept of a point estimate becomes vital. A point estimate is a single value that serves as the best guess for an unknown population parameter, in this case, the population standard deviation. This article delves deep into understanding point estimates for population standard deviation, exploring different methods, their applications, and limitations.
Understanding Population Standard Deviation
Before diving into point estimation, let's solidify our understanding of the population standard deviation. It measures how much individual data points deviate from the population mean (μ). A higher standard deviation indicates greater variability, while a lower value signifies data points clustered closely around the mean. Calculating the population standard deviation directly involves using every single data point from the entire population:
Formula: σ = √[ Σ(xi - μ)² / N ]
Where:
- σ: Population standard deviation
- xi: Individual data point
- μ: Population mean
- N: Total number of data points in the population
- Σ: Summation
The Need for Point Estimation
As mentioned earlier, obtaining the true population standard deviation is often infeasible. Therefore, we resort to sampling. We collect a sample from the population and use the sample data to estimate the population standard deviation. This estimate is our point estimate. The accuracy of this estimate depends on the sample size and the variability within the sample itself. A larger, representative sample generally leads to a more accurate estimate.
Sample Standard Deviation: The Foundation of Point Estimation
The sample standard deviation, denoted by 's', serves as the foundation for point estimating the population standard deviation. It's calculated using a slightly different formula than the population standard deviation, primarily because we use the sample mean (x̄) as an estimate for the population mean (μ). The crucial difference lies in the denominator:
Formula: s = √[ Σ(xi - x̄)² / (n - 1) ]
Where:
- s: Sample standard deviation
- xi: Individual data point in the sample
- x̄: Sample mean
- n: Sample size
- Σ: Summation
Notice the (n-1) in the denominator. This is known as Bessel's correction. It adjusts the sample standard deviation to provide a less biased estimate of the population standard deviation. Without Bessel's correction, the sample standard deviation would tend to underestimate the population standard deviation, especially with smaller sample sizes.
Methods for Point Estimation of Population Standard Deviation
The sample standard deviation ('s') itself is the most common and widely used point estimate for the population standard deviation (σ). While it's not a perfect estimate, it's unbiased and consistent, meaning its value will converge towards the true population standard deviation as the sample size increases.
Understanding Bias and Consistency in Estimation
Let's briefly elaborate on bias and consistency in the context of point estimation:
-
Bias: A biased estimator consistently overestimates or underestimates the true population parameter. The sample standard deviation (with Bessel's correction) is an unbiased estimator of the population standard deviation.
-
Consistency: A consistent estimator's value gets closer to the true population parameter as the sample size increases. The sample standard deviation is a consistent estimator.
Confidence Intervals: Beyond Point Estimates
While a point estimate provides a single value, it doesn't give us a sense of the uncertainty associated with that estimate. This is where confidence intervals come into play. A confidence interval provides a range of values within which the true population parameter is likely to fall, with a specified level of confidence (e.g., 95%). For population standard deviation, constructing a confidence interval is more complex than for the mean, and often requires the use of the chi-square distribution.
Chi-Square Distribution and Confidence Intervals for Standard Deviation
The chi-square (χ²) distribution plays a crucial role in constructing confidence intervals for the population standard deviation. The calculation involves the sample standard deviation, the sample size, and the chi-square critical values corresponding to the desired confidence level and degrees of freedom (n-1).
The formula for a confidence interval is:
√[(n-1)s²/χ²<sub>(α/2, n-1)</sub>] < σ < √[(n-1)s²/χ²<sub>(1-α/2, n-1)</sub>]
Where:
- n: Sample size
- s: Sample standard deviation
- α: Significance level (1 - confidence level)
- χ²<sub>(α/2, n-1)</sub> and χ²<sub>(1-α/2, n-1)</sub>: Chi-square critical values from the chi-square distribution table with (n-1) degrees of freedom.
Assumptions and Limitations
The accuracy of the point estimate and confidence interval relies on certain assumptions:
-
Random Sampling: The sample must be randomly selected from the population to ensure it's representative.
-
Independence: Observations within the sample must be independent of each other.
-
Normality (for smaller samples): While the Central Limit Theorem allows for some relaxation of this assumption with larger samples, for smaller samples (generally less than 30), the underlying population should ideally be normally distributed. For non-normal distributions, other methods might be more appropriate.
Interpreting the Point Estimate and Confidence Interval
The point estimate ('s') gives a single best guess for σ. However, the confidence interval provides a more informative picture. For example, a 95% confidence interval of (10, 15) suggests that there's a 95% chance that the true population standard deviation lies between 10 and 15. It acknowledges the inherent uncertainty in estimating the population parameter from a sample.
Applications of Point Estimation of Population Standard Deviation
Point estimation of the population standard deviation finds applications in various fields:
-
Quality Control: Estimating the variability of a manufacturing process.
-
Finance: Assessing the risk associated with investments.
-
Healthcare: Measuring the variability in patient outcomes.
-
Environmental Science: Analyzing the variability of pollutant levels.
-
Social Sciences: Studying the variability in survey responses.
Advanced Methods and Considerations
While the sample standard deviation is the standard point estimate, other methods exist for specific scenarios or distributions. For example, robust estimators are less sensitive to outliers, which can significantly influence the sample standard deviation.
Conclusion
Point estimation of population standard deviation is a fundamental concept in inferential statistics. The sample standard deviation provides a practical and unbiased point estimate, though it's crucial to acknowledge the inherent uncertainty associated with this estimate. Confidence intervals, constructed using the chi-square distribution, provide a more complete picture by quantifying the range within which the true population standard deviation is likely to lie. Understanding these concepts is essential for researchers and practitioners across various disciplines who need to make inferences about population parameters based on sample data. Remember to always consider the assumptions and limitations of the chosen method and interpret the results appropriately within the context of the study. By carefully applying these techniques, you can obtain reliable and informative estimates of the population standard deviation.
Latest Posts
Latest Posts
-
Is Argon A Metal Metalloid Or Nonmetal
May 09, 2025
-
Why Are Cells Considered The Most Basic Unit Of Life
May 09, 2025
-
What Is The Importance Of Nad Cycling Backup To Glycolysis
May 09, 2025
-
Is Color An Intensive Or Extensive Property
May 09, 2025
-
Chemistry And Chemical Reactivity 10th Edition Pdf Free
May 09, 2025
Related Post
Thank you for visiting our website which covers about Point Estimate Of Population Standard Deviation . We hope the information provided has been useful to you. Feel free to contact us if you have any questions or need further assistance. See you next time and don't miss to bookmark.