Point Estimate Of The Population Standard Deviation
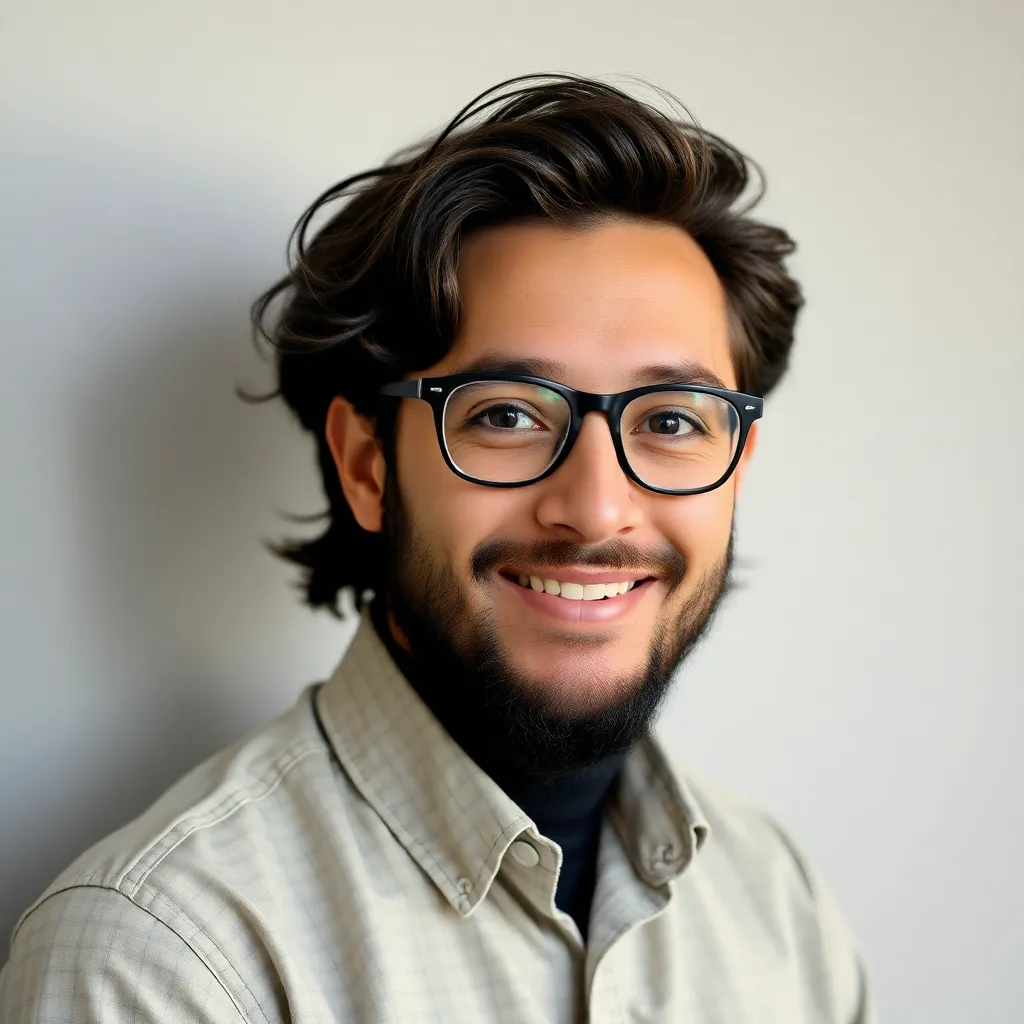
Muz Play
Apr 03, 2025 · 6 min read
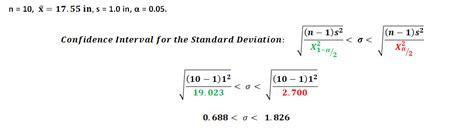
Table of Contents
Point Estimate of the Population Standard Deviation: A Comprehensive Guide
Estimating the population standard deviation is a crucial task in statistical inference. Unlike the population mean, which has a straightforward point estimate (the sample mean), estimating the population standard deviation requires a more nuanced approach. This article delves deep into the concept of the point estimate of the population standard deviation, exploring its various methods, assumptions, and applications. We will also discuss the limitations and potential biases associated with different estimation techniques.
Understanding the Population Standard Deviation
Before diving into estimation methods, it's crucial to understand what the population standard deviation represents. The population standard deviation (σ) measures the dispersion or spread of data points in an entire population. A higher standard deviation indicates greater variability, while a lower standard deviation suggests data points cluster closely around the population mean (μ). In most real-world scenarios, accessing the entire population is impractical or impossible, leading to the need for estimation using sample data.
Sample Standard Deviation: The Foundation of Estimation
The sample standard deviation (s) is the most common starting point for estimating the population standard deviation. It's calculated from a sample of data drawn from the population and serves as an approximation of the population's variability. The formula for the sample standard deviation is:
s = √[Σ(xi - x̄)² / (n - 1)]
where:
- xi represents individual data points in the sample.
- x̄ represents the sample mean.
- n represents the sample size.
- (n-1) represents the Bessel's correction, crucial for unbiased estimation.
Why Bessel's Correction?
Bessel's correction (using n-1 instead of n in the denominator) adjusts the sample standard deviation to provide an unbiased estimator of the population standard deviation. Without this correction, the sample standard deviation would consistently underestimate the population standard deviation, particularly with smaller sample sizes. This is because the sample mean tends to underestimate the true population mean and subsequently underestimates the variability around it. The use of (n-1) corrects for this inherent underestimation, offering a more reliable estimate.
Point Estimates of the Population Standard Deviation
While the sample standard deviation (s) is a good starting point, it's not a perfect point estimate of the population standard deviation (σ). Several other methods and considerations exist to refine this estimate:
1. Using the Sample Standard Deviation Directly: A Simple Approach
The most straightforward point estimate is simply using the sample standard deviation (s) as a direct estimate for σ. This approach is easy to compute but suffers from bias, especially with smaller samples. Its simplicity makes it suitable for quick estimations when high precision isn't critical. However, it's crucial to understand its limitations and acknowledge the potential for underestimation.
2. Bias Correction Techniques
Given the inherent bias of using 's' directly, several techniques aim to correct for this bias and generate a more accurate estimate. These techniques are mostly used when the sample size is small (generally considered less than 30). These include:
-
Adjusted Sample Standard Deviation: Some statisticians advocate using a slightly adjusted version of the sample standard deviation that incorporates a bias correction factor based on the sample size. These adjustments are often small for larger samples but can be significant for small ones.
-
Using Confidence Intervals: Instead of a single point estimate, a confidence interval provides a range of plausible values for σ. This approach acknowledges the uncertainty inherent in estimating a population parameter from a sample. The confidence interval is built around the sample standard deviation and uses a distribution (like the chi-squared distribution) to quantify the uncertainty.
3. Maximum Likelihood Estimation (MLE)
MLE provides another method for estimating σ. MLE finds the parameter value (in this case, σ) that maximizes the likelihood of observing the obtained sample data. The MLE estimate for σ is slightly different from the sample standard deviation; it uses 'n' instead of 'n-1' in the denominator. While this method is mathematically elegant, it tends to be biased, especially for small samples. Its use is more common in advanced statistical modeling and machine learning applications.
Assumptions and Considerations
The accuracy of any point estimate of the population standard deviation depends heavily on several assumptions:
-
Random Sampling: The sample data must be representative of the population. A biased sample will lead to a biased estimate of σ.
-
Normality: While the sample standard deviation is relatively robust to deviations from normality, assuming a normally distributed population simplifies calculations and enhances the reliability of confidence intervals. For heavily skewed data, alternative methods might be needed.
-
Sample Size: A larger sample size generally leads to a more accurate and precise estimate of σ. The law of large numbers dictates that as the sample size increases, the sample standard deviation converges towards the population standard deviation.
Applications of Population Standard Deviation Estimation
Estimating the population standard deviation finds wide applications across various fields:
-
Quality Control: In manufacturing, estimating the standard deviation of product dimensions or quality attributes is crucial for monitoring production processes and maintaining quality standards.
-
Finance: Estimating the standard deviation of stock returns helps investors assess risk and make informed investment decisions.
-
Healthcare: In clinical trials, estimating the standard deviation of treatment responses is essential for determining the effectiveness of new drugs or therapies.
-
Environmental Science: Estimating the standard deviation of pollutant concentrations in environmental samples helps in monitoring pollution levels and assessing environmental risks.
-
Social Sciences: Estimating the standard deviation of survey responses allows researchers to quantify the variability of opinions or behaviors within a population.
Limitations and Potential Biases
It's important to remember that any point estimate, including estimates of the population standard deviation, is just an approximation. Potential limitations and sources of bias include:
-
Small Sample Size Bias: As mentioned earlier, small sample sizes can significantly affect the accuracy of the estimate.
-
Outliers: Extreme values (outliers) can disproportionately influence the sample standard deviation, leading to an overestimation of the population standard deviation. Robust methods, which are less sensitive to outliers, might be more appropriate in these cases.
-
Non-Normality: Significant deviations from normality can also affect the accuracy of the estimate, especially when using methods that rely on the assumption of normality.
Choosing the Right Method
The best method for estimating the population standard deviation depends on the specific context, the sample size, the distribution of the data, and the desired level of accuracy. Consider these factors when selecting a method:
-
Sample Size: For large samples, the sample standard deviation with Bessel's correction often provides a sufficient estimate. For small samples, bias correction techniques or confidence intervals might be more appropriate.
-
Data Distribution: If the data is significantly non-normal, consider robust methods or non-parametric alternatives.
-
Accuracy Requirements: If high accuracy is required, using confidence intervals to provide a range of plausible values is more appropriate than relying on a single point estimate.
Conclusion
Estimating the population standard deviation is a crucial aspect of statistical inference. While the sample standard deviation is a common starting point, understanding its limitations and considering alternative methods, such as bias correction techniques, maximum likelihood estimation, and confidence intervals, is vital. The choice of method should be informed by the sample size, data distribution, and desired level of accuracy. By carefully considering these aspects, researchers and analysts can obtain reliable estimates of the population standard deviation, enhancing the quality of their statistical analyses and decision-making processes. Remember that context and assumptions are key to choosing and interpreting the best estimate. Always strive for transparency and clearly state the method used and any limitations encountered in the process.
Latest Posts
Latest Posts
-
Artists Of The Early 20th Century
Apr 04, 2025
-
How To Find The Resistance Of A Circuit
Apr 04, 2025
-
Identify The Features Associated With Waxes
Apr 04, 2025
-
Rna Differs From Dna In That Rna
Apr 04, 2025
-
What Is The Net Change In Math
Apr 04, 2025
Related Post
Thank you for visiting our website which covers about Point Estimate Of The Population Standard Deviation . We hope the information provided has been useful to you. Feel free to contact us if you have any questions or need further assistance. See you next time and don't miss to bookmark.