Pretest And Posttest Control Group Design
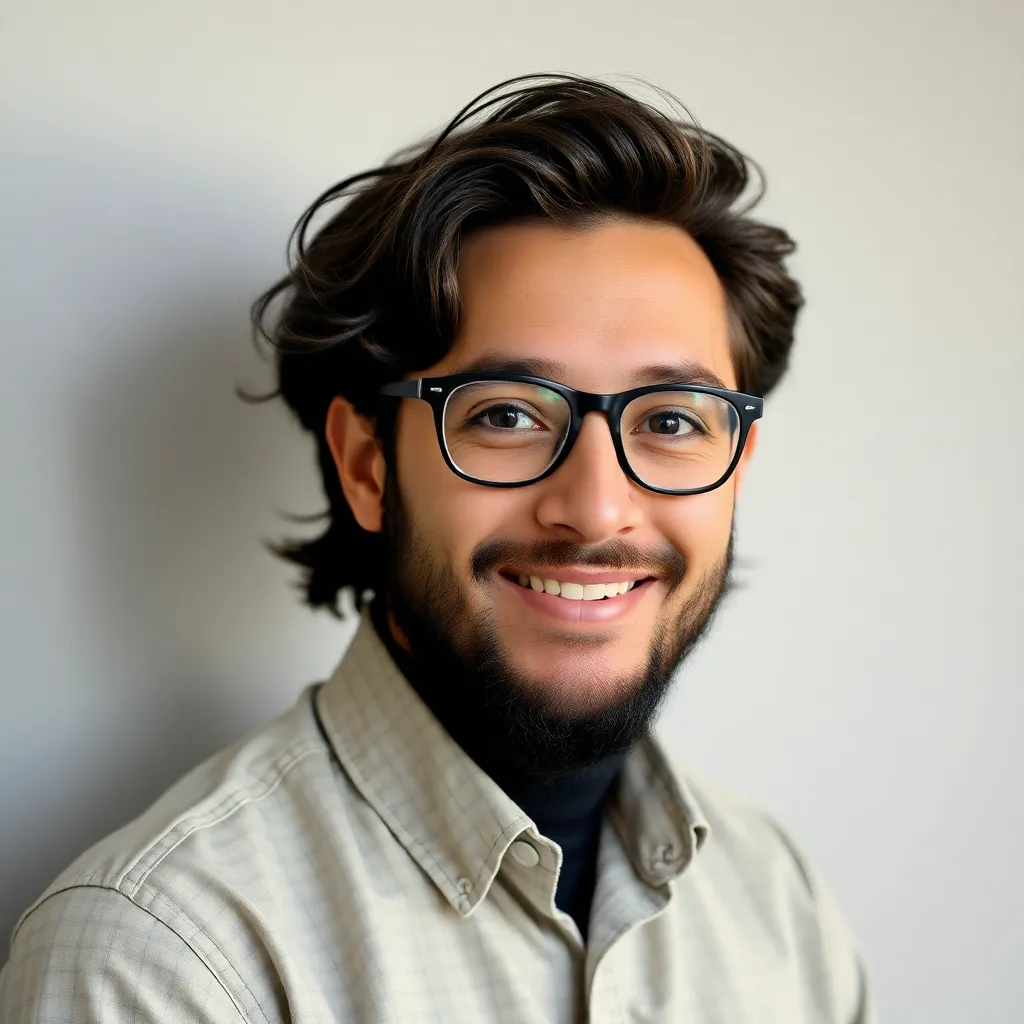
Muz Play
Apr 04, 2025 · 6 min read

Table of Contents
Pretest-Posttest Control Group Design: A Comprehensive Guide
The pretest-posttest control group design is a classic and robust experimental design frequently used in research across various fields, from education and psychology to medicine and marketing. It's a powerful tool for establishing cause-and-effect relationships by comparing the outcomes of an experimental group exposed to a treatment or intervention with a control group that is not. This article provides a comprehensive overview of this design, detailing its strengths, weaknesses, and variations, along with practical considerations for implementation and analysis.
Understanding the Pretest-Posttest Control Group Design
At its core, the pretest-posttest control group design involves two groups:
- Experimental Group: This group receives the treatment or intervention being studied.
- Control Group: This group does not receive the treatment. It serves as a baseline for comparison, helping researchers isolate the effects of the treatment.
Both groups are measured on the dependent variable (the outcome of interest) before (pretest) and after (posttest) the treatment is administered to the experimental group. This pre- and post-measurement allows researchers to assess the change in the dependent variable within each group and to compare the change between the two groups.
The basic structure looks like this:
Group | Pretest | Treatment | Posttest |
---|---|---|---|
Experimental Group | O1 | X | O2 |
Control Group | O3 | O4 |
Where:
- O1 and O3: Represent the pretest scores for the experimental and control groups respectively.
- X: Represents the treatment or intervention.
- O2 and O4: Represent the posttest scores for the experimental and control groups respectively.
Strengths of the Pretest-Posttest Control Group Design
This design offers several key advantages:
- Stronger Causal Inference: By comparing changes in both groups, this design significantly strengthens the ability to infer causality. If the experimental group shows a greater change than the control group, it provides strong evidence that the treatment caused the observed effect.
- Assessment of Pretreatment Differences: The pretest allows researchers to assess whether the groups are equivalent at the baseline. Any significant differences detected at the pretest can be statistically controlled for in the analysis, reducing the risk of spurious results.
- Measurement of Change: The design directly measures the change in the dependent variable within each group, providing valuable information beyond just comparing posttest scores alone. This allows for a more nuanced understanding of the treatment's impact.
- Flexibility and Applicability: This design can be adapted to various research questions and settings. It can be used with a wide range of dependent variables (quantitative or qualitative), and the treatment can take many forms (e.g., educational program, medication, marketing campaign).
Weaknesses of the Pretest-Posttest Control Group Design
Despite its strengths, the pretest-posttest control group design is not without limitations:
- Testing Effects: The act of taking a pretest can influence participants' responses on the posttest. This effect can be amplified if the pretest itself is related to the treatment. For example, taking a pretest on a particular topic might improve participants' performance on a posttest, regardless of the treatment.
- History Effects: External events occurring between the pretest and posttest can influence the results. These events might affect both groups equally, or they might disproportionately affect one group. For instance, a major news event related to the study's topic could influence participants' responses.
- Maturation Effects: Natural changes in participants over time (e.g., growth, learning) can confound the results. These changes are independent of the treatment but might be mistaken for treatment effects.
- Instrumentation Effects: Changes in the way the dependent variable is measured between the pretest and posttest can also affect the results. This could involve changes in the measuring instrument or the way data is collected.
- Regression to the Mean: If participants are selected based on extreme scores on the pretest, they may tend to score closer to the mean on the posttest, regardless of the treatment. This is particularly relevant when studying populations with naturally fluctuating scores.
- Attrition: Participants may drop out of the study between the pretest and posttest. Differential attrition (where one group loses more participants than the other) can bias the results.
Addressing Weaknesses: Variations and Considerations
Researchers employ several strategies to mitigate the weaknesses inherent in the pretest-posttest control group design:
- Solomon Four-Group Design: This variation adds two more groups: a control group without a pretest and an experimental group without a pretest. This design helps isolate the effects of the pretest itself.
- Careful Control of Extraneous Variables: Researchers should strive to minimize the influence of history, maturation, and instrumentation effects through careful experimental control, standardized procedures, and rigorous data collection methods.
- Random Assignment: Randomly assigning participants to either the experimental or control group helps ensure that the groups are comparable at the outset, reducing the risk of selection bias.
- Statistical Control: Statistical techniques like analysis of covariance (ANCOVA) can be used to control for pre-existing differences between groups observed in the pretest.
- Addressing Attrition: Researchers should strive for high retention rates and analyze attrition patterns to assess its potential impact on the results. If attrition is substantial and non-random, it might invalidate the findings.
Statistical Analysis of Pretest-Posttest Control Group Data
The most common statistical tests used to analyze data from a pretest-posttest control group design are:
- Independent Samples t-test: This test compares the means of the two groups on the posttest scores, controlling for any initial differences observed on the pretest.
- Paired Samples t-test: This test is used within each group to compare pretest and posttest scores. It assesses the change within each group separately.
- Analysis of Covariance (ANCOVA): This more sophisticated test controls for the pretest scores as a covariate, allowing for a more precise comparison of the posttest scores while accounting for any initial differences between the groups.
The choice of statistical test depends on the specific research question and the characteristics of the data. It's crucial to select the appropriate test based on the assumptions of the chosen test.
Practical Applications and Examples
The pretest-posttest control group design finds broad applications across various domains. Here are a few examples:
- Education: Evaluating the effectiveness of a new teaching method by comparing student performance (measured by tests) in classrooms using the new method (experimental group) with classrooms using the traditional method (control group).
- Medicine: Assessing the efficacy of a new drug by comparing changes in patient symptoms (measured using scales or physiological measures) in patients receiving the drug (experimental group) with patients receiving a placebo (control group).
- Marketing: Measuring the impact of an advertising campaign by comparing sales or brand awareness (measured through surveys or sales data) in regions exposed to the campaign (experimental group) with regions that were not (control group).
- Psychology: Examining the effectiveness of a therapeutic intervention by comparing changes in patient mental health scores (measured using standardized questionnaires) in patients receiving the intervention (experimental group) with patients on a waiting list (control group).
Conclusion: Choosing the Right Design
The pretest-posttest control group design is a powerful tool for causal inference when applied correctly. Understanding its strengths, weaknesses, and variations is crucial for researchers. By carefully considering potential confounding variables, employing appropriate statistical analyses, and addressing limitations through design modifications, researchers can leverage this design to generate robust and meaningful results, contributing significantly to their respective fields of study. The decision to use this design should be guided by the research question, the available resources, and the feasibility of controlling for potential confounding factors. While it is a powerful tool, its limitations must be acknowledged and addressed strategically to ensure the validity and reliability of the findings.
Latest Posts
Latest Posts
-
Is An Alloy A Heterogeneous Mixture
Apr 12, 2025
-
Find The Set Of Solutions For The Linear System
Apr 12, 2025
-
What Is The Path Of Projectile
Apr 12, 2025
-
What Is A System In Thermodynamics
Apr 12, 2025
-
Plasma Membranes Are A Feature Of
Apr 12, 2025
Related Post
Thank you for visiting our website which covers about Pretest And Posttest Control Group Design . We hope the information provided has been useful to you. Feel free to contact us if you have any questions or need further assistance. See you next time and don't miss to bookmark.