Probability Distribution Mean And Standard Deviation
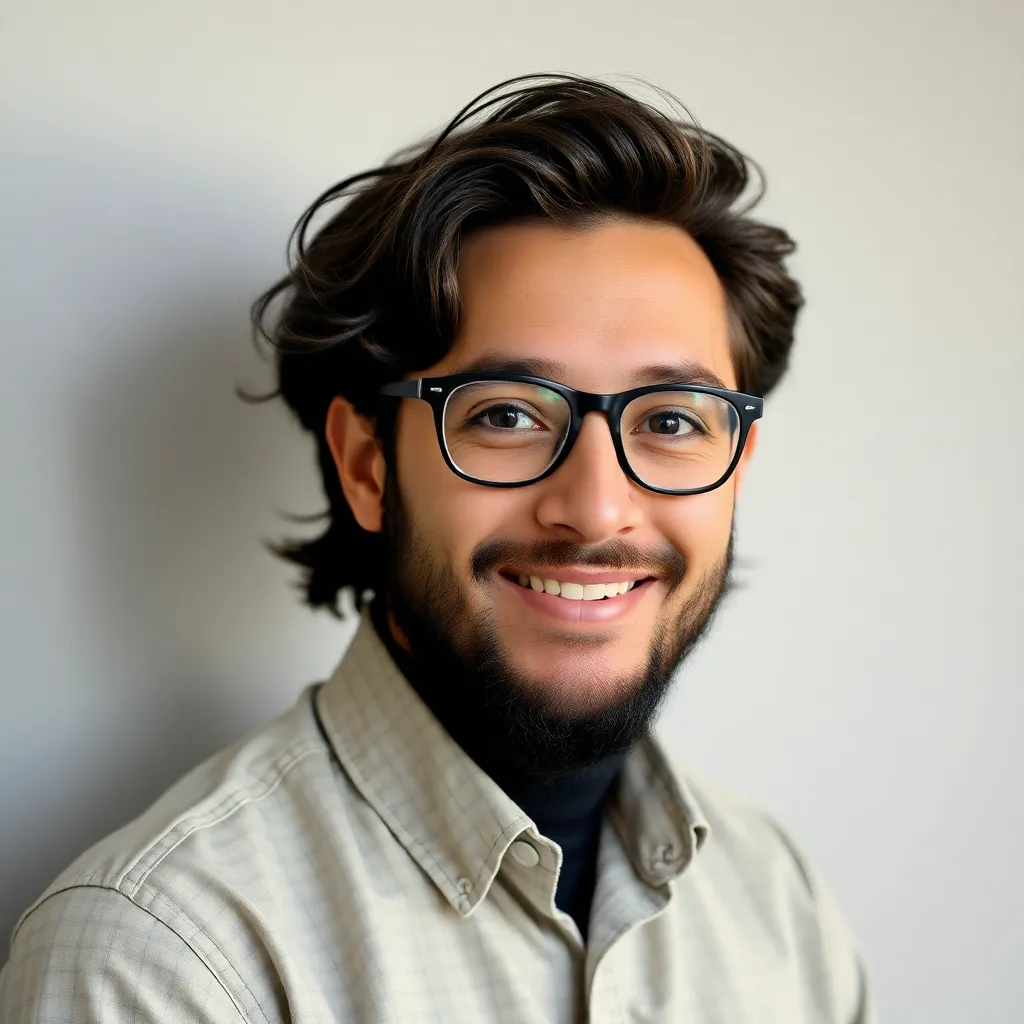
Muz Play
Mar 13, 2025 · 6 min read

Table of Contents
Probability Distribution: Mean and Standard Deviation – A Deep Dive
Understanding probability distributions is fundamental to statistics and numerous fields relying on data analysis. From finance and engineering to medicine and social sciences, the ability to describe and interpret the distribution of data is crucial. At the heart of this understanding lies the ability to calculate and interpret two key descriptive statistics: the mean and the standard deviation. This article delves deep into the concepts of mean and standard deviation within the context of various probability distributions, explaining their significance and providing practical examples.
What is a Probability Distribution?
A probability distribution describes the likelihood of obtaining different possible values for a random variable. It's a function that assigns probabilities to each possible outcome. There are several types of probability distributions, each with its own characteristics and applications. Some common examples include:
- Normal Distribution: The bell-shaped curve, symmetrical around the mean. It's prevalent in many natural phenomena and statistical analyses.
- Binomial Distribution: Models the probability of success or failure in a fixed number of independent Bernoulli trials (e.g., coin flips).
- Poisson Distribution: Describes the probability of a given number of events occurring in a fixed interval of time or space (e.g., number of cars passing a point on a highway in an hour).
- Uniform Distribution: All outcomes have equal probability.
- Exponential Distribution: Models the time between events in a Poisson process (e.g., time until a machine breaks down).
Mean: The Center of the Distribution
The mean, often referred to as the average or expected value, represents the central tendency of a probability distribution. It's the weighted average of all possible values, where the weights are the probabilities of those values. Mathematically, for a discrete probability distribution, the mean (μ) is calculated as:
μ = Σ [xi * P(xi)]
where:
- xi represents each possible value of the random variable.
- P(xi) represents the probability of the random variable taking on the value xi.
- Σ denotes the summation over all possible values of xi.
For a continuous probability distribution, the mean is calculated using an integral:
μ = ∫ x * f(x) dx
where:
- f(x) is the probability density function of the continuous random variable.
- The integral is taken over the entire range of possible values of x.
The mean provides a single value that summarizes the "typical" or "central" value of the distribution. However, it's important to remember that the mean can be misleading if the distribution is skewed (asymmetrical).
Examples of Mean Calculation:
Example 1 (Discrete): Consider a simple lottery with three possible prizes: $10 (probability 0.6), $50 (probability 0.3), and $100 (probability 0.1). The mean is:
μ = (10 * 0.6) + (50 * 0.3) + (100 * 0.1) = 6 + 15 + 10 = $31
Example 2 (Continuous): For a normally distributed variable, the mean is the point of symmetry. If a dataset is normally distributed with a mean of 70 and a standard deviation of 10, the mean is simply 70.
Standard Deviation: Measuring the Spread
The standard deviation (σ) measures the dispersion or spread of the data around the mean. A small standard deviation indicates that the data points are clustered closely around the mean, while a large standard deviation suggests that the data is more spread out. It quantifies the variability or uncertainty associated with the random variable.
For a discrete probability distribution, the standard deviation is calculated as:
σ = √[Σ [(xi - μ)² * P(xi)]]
For a continuous probability distribution:
σ = √[∫ (x - μ)² * f(x) dx]
The standard deviation is the square root of the variance (σ²), which is the average of the squared differences from the mean. The use of squared differences ensures that both positive and negative deviations contribute positively to the overall variance.
Interpreting Standard Deviation:
The standard deviation is often used in conjunction with the mean to provide a complete picture of the distribution. For example, in a normal distribution:
- Approximately 68% of the data falls within one standard deviation of the mean (μ ± σ).
- Approximately 95% of the data falls within two standard deviations of the mean (μ ± 2σ).
- Approximately 99.7% of the data falls within three standard deviations of the mean (μ ± 3σ).
This empirical rule is extremely useful for understanding the spread of data in normally distributed datasets.
Examples of Standard Deviation Calculation:
Example 1 (Discrete): Using the lottery example above, with a mean of $31:
σ = √[((10 - 31)² * 0.6) + ((50 - 31)² * 0.3) + ((100 - 31)² * 0.1)] ≈ √[252 + 117 + 476.1] ≈ √845.1 ≈ $29.07
Example 2 (Continuous): In the normal distribution example with a mean of 70 and a standard deviation of 10, the standard deviation is simply 10. This tells us that the data is relatively spread out around the mean.
Mean and Standard Deviation in Different Distributions:
The calculations for mean and standard deviation differ slightly depending on the type of probability distribution. Here's a brief overview for some common distributions:
Normal Distribution:
- Mean (μ): The mean is the center of the symmetrical bell curve.
- Standard Deviation (σ): Determines the width of the bell curve. A larger standard deviation results in a wider, flatter curve, while a smaller standard deviation results in a narrower, taller curve.
Binomial Distribution:
- Mean (μ): μ = n * p, where 'n' is the number of trials and 'p' is the probability of success in a single trial.
- Standard Deviation (σ): σ = √[n * p * (1 - p)]
Poisson Distribution:
- Mean (μ): The mean is equal to the parameter λ (lambda), which represents the average rate of events.
- Standard Deviation (σ): σ = √λ
Uniform Distribution:
- Mean (μ): μ = (a + b) / 2, where 'a' and 'b' are the lower and upper bounds of the distribution.
- Standard Deviation (σ): σ = √[(b - a)² / 12]
Significance of Mean and Standard Deviation:
The mean and standard deviation are crucial for:
- Summarizing data: They provide a concise summary of the central tendency and variability of a dataset.
- Comparing datasets: They allow for the comparison of different datasets based on their central tendency and spread.
- Making inferences: They are essential for making inferences about populations based on sample data.
- Hypothesis testing: They are key components of many statistical hypothesis tests.
- Risk assessment: In finance and other fields, they help quantify and manage risk.
- Quality control: In manufacturing and other industries, they are used to monitor and control the quality of products or processes.
Practical Applications:
The applications of mean and standard deviation are vast and widespread. Here are a few examples:
- Investment analysis: Investors use the mean and standard deviation of stock returns to assess risk and potential returns. A high standard deviation indicates higher risk.
- Medical research: Researchers use these statistics to analyze the effectiveness of treatments and to understand the variability of patient responses.
- Manufacturing: Quality control processes often rely on the mean and standard deviation to monitor the consistency of products.
- Weather forecasting: Meteorologists use these statistics to analyze weather patterns and make predictions.
Conclusion:
The mean and standard deviation are fundamental concepts in probability and statistics. Understanding how to calculate and interpret these statistics is essential for anyone working with data. They provide valuable insights into the central tendency and variability of data, enabling informed decisions in a wide range of fields. This article has provided a comprehensive overview of these concepts, encompassing their definitions, calculation methods, interpretations, and practical applications across different probability distributions. Mastering these concepts is key to unlocking a deeper understanding of data analysis and its implications.
Latest Posts
Latest Posts
-
The Diagram Below Shows Some Subatomic Particles
May 09, 2025
-
Stirring Increases The Rate Of Dissolution Because It
May 09, 2025
-
What Layer Of The Skin Is Avascular
May 09, 2025
-
Area Where Two Or More Bones Join Together
May 09, 2025
-
Do Isotopes Have The Same Mass Number
May 09, 2025
Related Post
Thank you for visiting our website which covers about Probability Distribution Mean And Standard Deviation . We hope the information provided has been useful to you. Feel free to contact us if you have any questions or need further assistance. See you next time and don't miss to bookmark.