Statistics And Probability With Applications Pdf
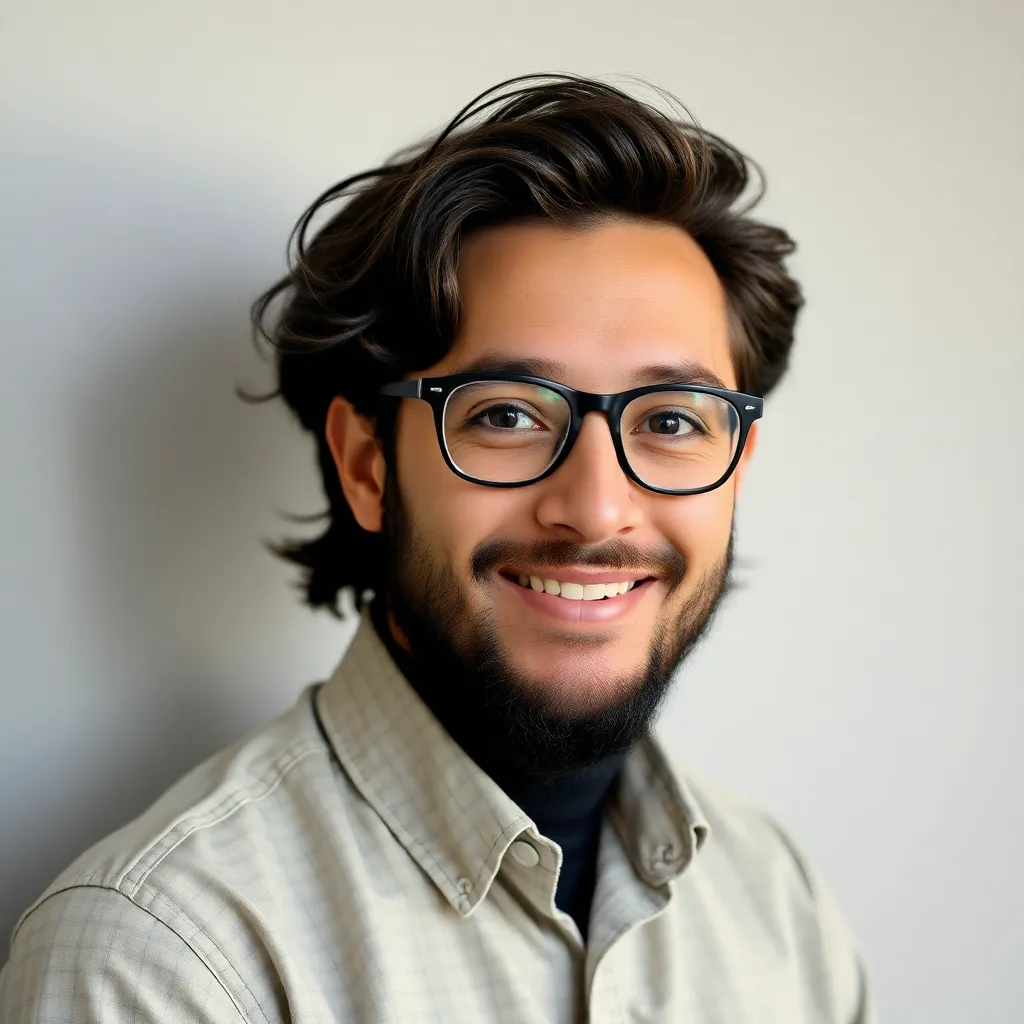
Muz Play
Apr 12, 2025 · 6 min read

Table of Contents
Statistics and Probability with Applications: A Comprehensive Guide
The fields of statistics and probability are fundamental to numerous disciplines, providing the tools to analyze data, make informed decisions, and model real-world phenomena. This comprehensive guide explores the core concepts of statistics and probability, illustrating their applications across various fields with practical examples. While a PDF download isn't provided here (as per instructions), this article serves as a thorough resource, covering key topics in detail.
I. Understanding Probability: The Foundation of Statistical Inference
Probability quantifies the likelihood of an event occurring. It forms the bedrock upon which statistical inference is built. We explore several key concepts:
A. Basic Probability Concepts
- Experiment: Any process that results in an outcome. Examples include flipping a coin, rolling a die, or surveying a population.
- Sample Space (S): The set of all possible outcomes of an experiment. For a coin flip, S = {Heads, Tails}. For rolling a six-sided die, S = {1, 2, 3, 4, 5, 6}.
- Event (E): A subset of the sample space. For example, in rolling a die, the event "rolling an even number" would be E = {2, 4, 6}.
- Probability of an Event: The ratio of the number of favorable outcomes to the total number of possible outcomes. For example, the probability of rolling an even number on a fair six-sided die is P(E) = 3/6 = 1/2.
Calculating Probabilities:
Several methods exist for calculating probabilities, including:
- Classical Probability: Assumes all outcomes are equally likely (e.g., a fair coin or die).
- Empirical Probability: Based on observed frequencies from experiments or data. For example, if a coin is flipped 100 times and lands on heads 55 times, the empirical probability of heads is 0.55.
- Subjective Probability: Based on personal judgment or belief.
B. Types of Probability Distributions
Understanding probability distributions is crucial for statistical analysis. Key distributions include:
- Binomial Distribution: Models the probability of getting a certain number of successes in a fixed number of independent Bernoulli trials (trials with only two outcomes, like success/failure). Examples include the number of heads in 10 coin flips or the number of defective items in a batch.
- Poisson Distribution: Models the probability of a certain number of events occurring in a fixed interval of time or space, when events occur independently and at a constant average rate. Examples include the number of customers arriving at a store per hour or the number of typos on a page.
- Normal Distribution: A continuous probability distribution characterized by its bell shape. It's crucial in many statistical applications due to the Central Limit Theorem, which states that the average of a large number of independent and identically distributed random variables tends to follow a normal distribution, regardless of the original distribution's shape.
II. Descriptive Statistics: Summarizing Data
Descriptive statistics involves methods for summarizing and presenting data in a meaningful way. This includes:
A. Measures of Central Tendency
These values describe the center of a dataset:
- Mean: The average of the data points.
- Median: The middle value when the data is sorted.
- Mode: The most frequent value.
The choice of central tendency measure depends on the data's distribution. For symmetrical distributions, the mean, median, and mode are typically similar. For skewed distributions, the median is often a more robust measure.
B. Measures of Dispersion
These values describe the spread or variability of the data:
- Range: The difference between the maximum and minimum values.
- Variance: The average of the squared deviations from the mean.
- Standard Deviation: The square root of the variance, providing a measure of spread in the original units.
- Interquartile Range (IQR): The difference between the 75th percentile (Q3) and the 25th percentile (Q1). Robust to outliers.
C. Data Visualization
Visualizing data is essential for understanding patterns and trends. Common methods include:
- Histograms: Display the frequency distribution of a continuous variable.
- Box Plots: Show the median, quartiles, and potential outliers.
- Scatter Plots: Illustrate the relationship between two variables.
III. Inferential Statistics: Making Inferences from Data
Inferential statistics involves drawing conclusions about a population based on a sample. Key concepts include:
A. Sampling and Sampling Distributions
- Population: The entire group of interest.
- Sample: A subset of the population.
- Sampling Distribution: The probability distribution of a sample statistic (e.g., the sample mean) across all possible samples of a given size. The Central Limit Theorem is crucial here.
B. Hypothesis Testing
Hypothesis testing is a formal procedure for deciding whether there is enough evidence to reject a null hypothesis (a statement of no effect or difference). Key steps include:
- State the null and alternative hypotheses.
- Choose a significance level (alpha).
- Calculate a test statistic.
- Determine the p-value.
- Make a decision. Reject the null hypothesis if the p-value is less than alpha.
C. Confidence Intervals
A confidence interval provides a range of values that is likely to contain the true population parameter with a certain level of confidence. For example, a 95% confidence interval for the population mean suggests that there's a 95% chance the true mean lies within that range.
D. Regression Analysis
Regression analysis examines the relationship between a dependent variable and one or more independent variables. Linear regression models a linear relationship, while other models handle more complex relationships. Applications range from predicting house prices based on features to analyzing the effect of advertising on sales.
IV. Applications of Statistics and Probability
The applications of statistics and probability are vast and span numerous fields:
A. Business and Finance
- Market research: Analyzing consumer preferences and trends.
- Risk management: Assessing and mitigating financial risks.
- Investment analysis: Evaluating the performance of investments.
- Actuarial science: Assessing and managing insurance risks.
B. Healthcare
- Clinical trials: Evaluating the effectiveness of new treatments.
- Epidemiology: Studying the distribution and causes of diseases.
- Public health: Monitoring and improving public health outcomes.
C. Engineering
- Quality control: Ensuring the quality of manufactured products.
- Reliability engineering: Assessing the reliability of systems.
- Experimental design: Designing experiments to test hypotheses.
D. Social Sciences
- Survey research: Gathering and analyzing data from surveys.
- Social network analysis: Studying social networks and their structure.
- Political science: Analyzing voting patterns and political opinions.
E. Environmental Science
- Environmental monitoring: Monitoring environmental conditions and changes.
- Ecological modeling: Modeling ecological systems and their dynamics.
- Climate change research: Studying the causes and effects of climate change.
V. Conclusion: Mastering the Power of Data
Statistics and probability provide the essential tools for understanding and interpreting data in a wide variety of contexts. From basic descriptive statistics to complex inferential methods, the ability to analyze data effectively is increasingly valuable in today's data-rich world. This comprehensive overview has touched upon key concepts and applications, showcasing the power and versatility of these powerful mathematical disciplines. Continued learning and practical application will solidify understanding and enhance one's ability to extract meaningful insights from data, driving informed decision-making and contributing to advancements across numerous fields.
Latest Posts
Latest Posts
-
What Is The Direction Of Centripetal Acceleration
Apr 13, 2025
-
What Type Of Solid Is A Diamond
Apr 13, 2025
-
How To Find Sample Mean From Confidence Interval
Apr 13, 2025
-
What Is The Difference Between A Proton And An Electron
Apr 13, 2025
-
Identifying Oxidized And Reduced Reactants In A Single Displacement Reaction
Apr 13, 2025
Related Post
Thank you for visiting our website which covers about Statistics And Probability With Applications Pdf . We hope the information provided has been useful to you. Feel free to contact us if you have any questions or need further assistance. See you next time and don't miss to bookmark.