T Test For A Correlation Coefficient
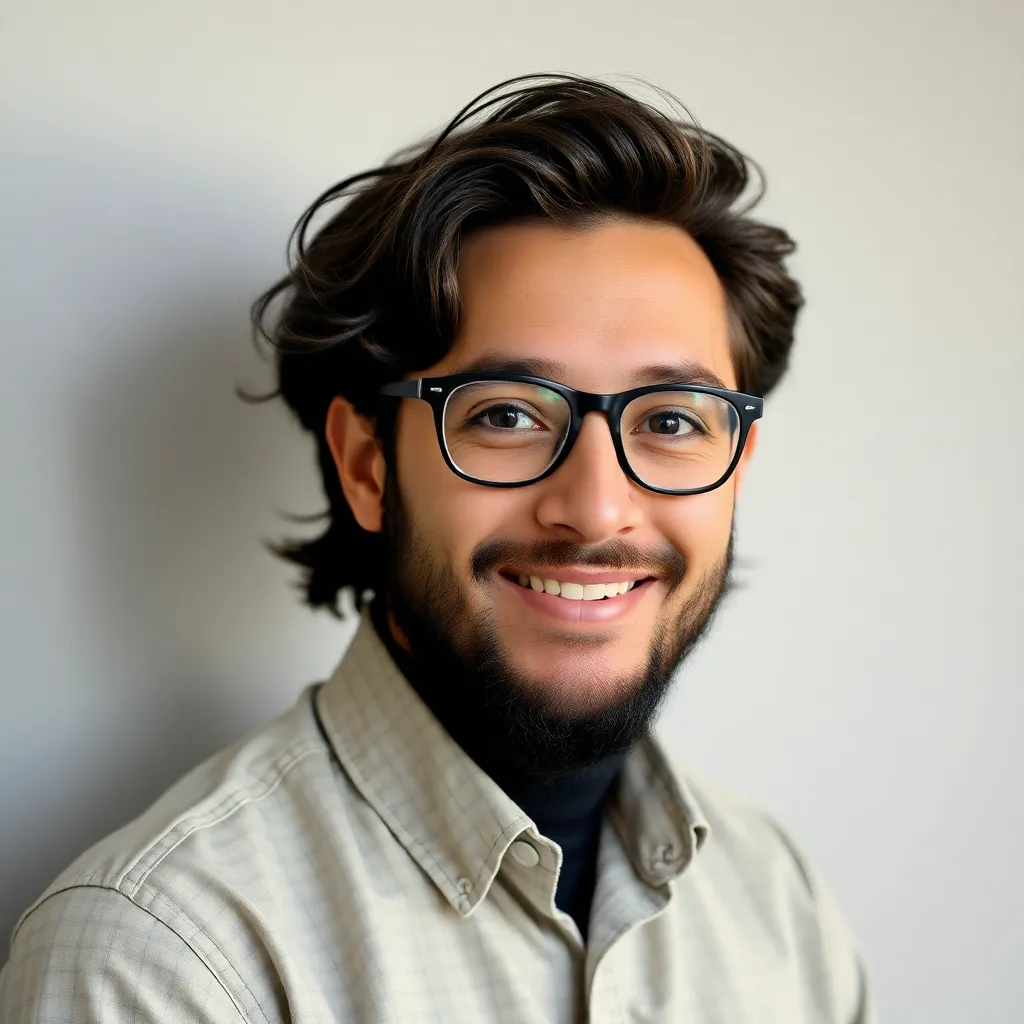
Muz Play
Mar 10, 2025 · 5 min read

Table of Contents
T-Test for a Correlation Coefficient: A Comprehensive Guide
The correlation coefficient, often represented as r, measures the strength and direction of a linear relationship between two variables. While calculating r provides valuable insight, it doesn't tell the whole story. A statistically significant correlation suggests that the observed relationship isn't merely due to random chance. This is where the t-test for a correlation coefficient comes in. This test assesses the statistical significance of the correlation, helping researchers determine if the observed relationship is likely to exist in the population from which the sample was drawn. This article provides a comprehensive guide to understanding and applying the t-test for a correlation coefficient.
Understanding Correlation and its Significance
Before diving into the t-test, let's revisit the concept of correlation. A correlation coefficient ranges from -1 to +1:
- +1: Indicates a perfect positive correlation; as one variable increases, the other increases proportionally.
- -1: Indicates a perfect negative correlation; as one variable increases, the other decreases proportionally.
- 0: Indicates no linear correlation between the variables.
However, a correlation coefficient alone doesn't indicate statistical significance. A seemingly strong correlation in a small sample might not hold true for a larger population. The t-test helps address this uncertainty.
Types of Correlation Coefficients
While Pearson's correlation coefficient is the most common, other types exist, each suited for different data types:
- Pearson's r: Used for continuous data with a linear relationship. Assumes normality and equal variance.
- Spearman's rho: Used for ordinal data or when the relationship isn't strictly linear. Less sensitive to outliers.
- Kendall's tau: Another non-parametric correlation coefficient, suitable for ordinal data and less sensitive to outliers than Spearman's rho. Often preferred when dealing with tied ranks.
This article focuses primarily on the t-test used with Pearson's r, as it's the most widely applied in statistical analysis.
The T-Test for a Correlation Coefficient: A Step-by-Step Guide
The t-test for a correlation coefficient assesses the null hypothesis that the population correlation coefficient (ρ) is zero. In other words, it tests whether the observed correlation in the sample is strong enough to conclude that a true relationship exists in the larger population.
Hypotheses:
- Null Hypothesis (H₀): ρ = 0 (There is no correlation between the variables in the population.)
- Alternative Hypothesis (H₁): ρ ≠ 0 (There is a correlation between the variables in the population.) This can also be one-tailed: ρ > 0 (positive correlation) or ρ < 0 (negative correlation).
Formula:
The t-statistic is calculated using the following formula:
t = r√[(n-2)/(1-r²)]
Where:
- r: The sample correlation coefficient.
- n: The sample size.
Degrees of Freedom (df):
The degrees of freedom for this t-test are:
df = n - 2
Critical Value:
To determine statistical significance, you compare the calculated t-statistic to a critical value from the t-distribution table. This critical value depends on the chosen significance level (α, often 0.05) and the degrees of freedom.
Decision:
- If |t| > critical value: Reject the null hypothesis. There is sufficient evidence to conclude that a significant correlation exists between the variables.
- If |t| ≤ critical value: Fail to reject the null hypothesis. There is not enough evidence to conclude that a significant correlation exists.
Example: Applying the T-Test
Let's consider an example. Suppose a researcher is investigating the relationship between hours of study (X) and exam scores (Y). A sample of 20 students yields a correlation coefficient of r = 0.65. Let's test the significance of this correlation at α = 0.05.
-
Hypotheses:
- H₀: ρ = 0
- H₁: ρ ≠ 0 (two-tailed test)
-
Calculate the t-statistic:
- t = 0.65√[(20-2)/(1-0.65²)] ≈ 4.18
-
Degrees of freedom:
- df = 20 - 2 = 18
-
Find the critical value:
- Using a t-distribution table with df = 18 and α = 0.05 (two-tailed), the critical value is approximately ±2.101.
-
Decision:
- Since |4.18| > 2.101, we reject the null hypothesis. There is sufficient evidence to conclude that a significant positive correlation exists between hours of study and exam scores.
Interpreting the Results and Considerations
The t-test for a correlation coefficient provides a powerful tool for evaluating the statistical significance of a correlation. However, it's crucial to interpret the results cautiously:
-
Statistical Significance vs. Practical Significance: A statistically significant correlation doesn't necessarily imply practical significance. A small correlation coefficient, even if statistically significant, might not be meaningful in a real-world context. Consider the effect size alongside statistical significance.
-
Causation vs. Correlation: Correlation does not imply causation. Even a strong, statistically significant correlation doesn't prove that one variable causes changes in the other. Other factors might be involved.
-
Assumptions: The t-test for Pearson's r assumes linearity, normality, and homoscedasticity (equal variance). Violations of these assumptions can affect the validity of the results. Consider using non-parametric alternatives like Spearman's rho or Kendall's tau if assumptions are violated.
-
Outliers: Outliers can heavily influence the correlation coefficient. Inspect your data for outliers and consider their impact on the results. Robust correlation methods might be appropriate if outliers are present.
Advanced Topics and Extensions
Beyond the basic t-test, several advanced considerations enhance the analysis:
-
Confidence Intervals for ρ: Instead of just testing for significance, calculating a confidence interval for the population correlation coefficient (ρ) provides a range of plausible values.
-
Testing Differences Between Correlation Coefficients: Methods exist to compare correlation coefficients from different groups or samples.
-
Partial Correlation: This technique assesses the correlation between two variables while controlling for the influence of a third variable.
-
Multiple Regression: When dealing with multiple predictor variables, multiple regression analysis provides a more comprehensive approach to understanding relationships.
Conclusion: T-Test in the Broader Context of Statistical Analysis
The t-test for a correlation coefficient is a fundamental statistical tool used extensively across various disciplines. It enables researchers to move beyond simply observing a relationship between variables to making statistically sound inferences about the population. By understanding the procedure, interpreting results cautiously, and considering the assumptions and limitations, researchers can harness this test's power effectively to draw meaningful conclusions from their data. Remember to always consider the broader context of your research and use this test as one piece of evidence within a larger body of work. Further exploration of advanced statistical methods and appropriate data visualization techniques will strengthen your analysis and deepen your understanding of the relationships between variables.
Latest Posts
Latest Posts
-
How To Do Bohr Rutherford Diagrams
May 12, 2025
-
Is Milk Pure Substance Or Mixture
May 12, 2025
-
Power Series Of 1 1 X
May 12, 2025
-
Is Boron Trifluoride Polar Or Nonpolar
May 12, 2025
-
Which Point Of The Beam Experiences The Most Compression
May 12, 2025
Related Post
Thank you for visiting our website which covers about T Test For A Correlation Coefficient . We hope the information provided has been useful to you. Feel free to contact us if you have any questions or need further assistance. See you next time and don't miss to bookmark.