Testing A Hypothesis About A Population Mean
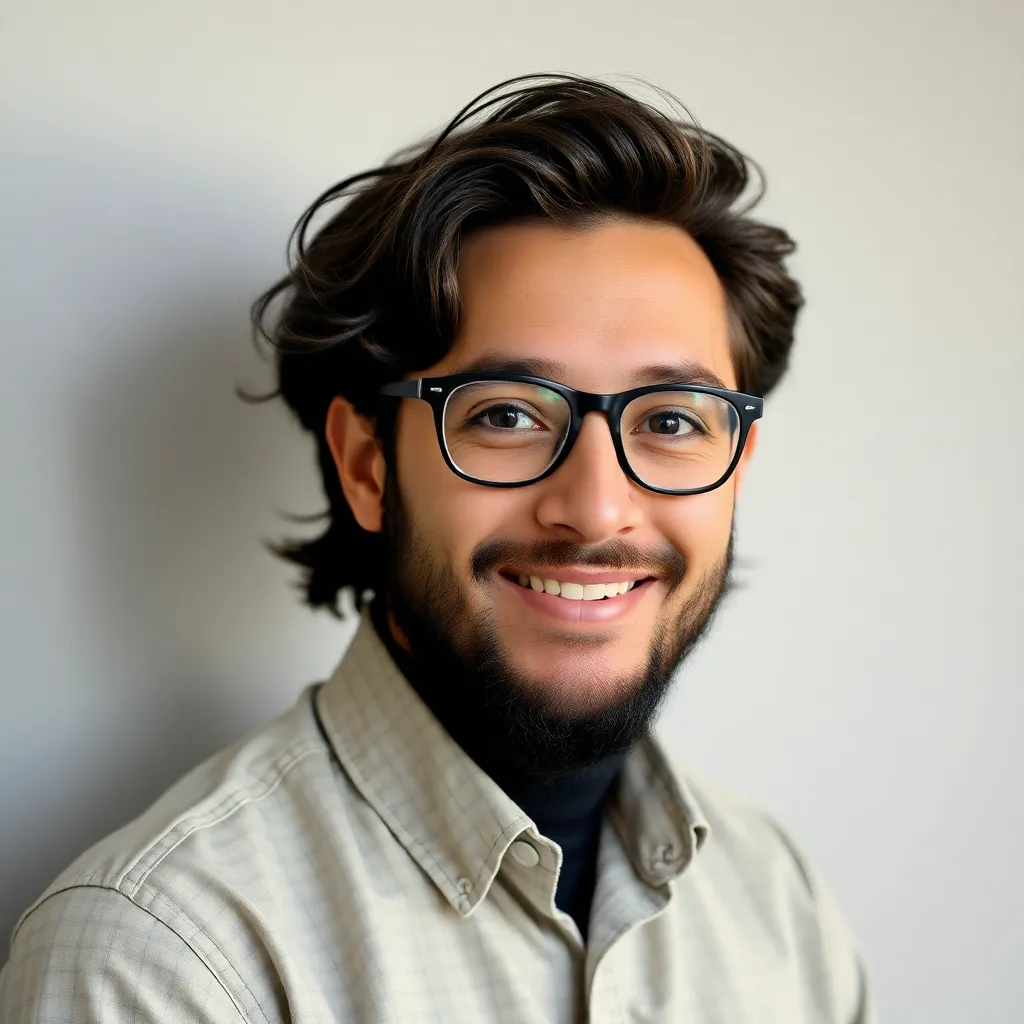
Muz Play
Apr 06, 2025 · 6 min read

Table of Contents
Testing a Hypothesis About a Population Mean: A Comprehensive Guide
Hypothesis testing is a cornerstone of statistical inference, allowing us to draw conclusions about a population based on a sample of data. One of the most common hypothesis tests involves examining a population mean. This article provides a comprehensive guide to understanding, performing, and interpreting these tests, covering various scenarios and considerations.
Understanding the Basics of Hypothesis Testing
Before diving into the specifics of testing a population mean, let's establish the fundamental concepts of hypothesis testing. The core idea revolves around formulating a null hypothesis (H₀) and an alternative hypothesis (H₁ or Hₐ).
-
Null Hypothesis (H₀): This is a statement of no effect or no difference. It's the status quo that we aim to disprove. For a population mean, the null hypothesis often states that the population mean (μ) is equal to a specific value (μ₀). For example: H₀: μ = 100.
-
Alternative Hypothesis (H₁): This is the statement we are trying to find evidence for. It contradicts the null hypothesis. There are three main types of alternative hypotheses:
- One-tailed (left-tailed): H₁: μ < μ₀ (The population mean is less than a specific value).
- One-tailed (right-tailed): H₁: μ > μ₀ (The population mean is greater than a specific value).
- Two-tailed: H₁: μ ≠ μ₀ (The population mean is different from a specific value).
The choice of alternative hypothesis depends on the research question. For instance, if we want to see if a new drug reduces blood pressure, we'd use a left-tailed test. If we want to see if a new fertilizer increases crop yield, we'd use a right-tailed test. If we simply want to know if a new teaching method changes test scores, we'd use a two-tailed test.
The process involves collecting a sample of data, calculating a test statistic, and comparing it to a critical value or calculating a p-value to determine whether there is enough evidence to reject the null hypothesis. Rejecting the null hypothesis means we have enough evidence to support the alternative hypothesis. Failing to reject the null hypothesis does not mean we've proven the null hypothesis is true; it simply means we don't have enough evidence to reject it.
Choosing the Appropriate Test: t-test vs. z-test
The choice between a t-test and a z-test depends on whether the population standard deviation (σ) is known.
-
Z-test: Used when the population standard deviation (σ) is known. This is rare in practice. The z-test relies on the standard normal distribution.
-
T-test: Used when the population standard deviation (σ) is unknown. This is much more common. The t-test relies on the t-distribution, which accounts for the uncertainty in estimating the standard deviation from the sample. The t-distribution is similar to the normal distribution but has heavier tails, reflecting the extra uncertainty. The degrees of freedom (df) for a one-sample t-test are n-1, where n is the sample size.
Steps in Hypothesis Testing for a Population Mean
Let's outline the steps for conducting a hypothesis test for a population mean using a t-test (the more common scenario):
-
State the hypotheses: Clearly define the null and alternative hypotheses.
-
Set the significance level (α): This is the probability of rejecting the null hypothesis when it is actually true (Type I error). Common significance levels are 0.05 (5%) and 0.01 (1%).
-
Collect data and calculate the sample statistics: Obtain a random sample from the population and calculate the sample mean (x̄) and the sample standard deviation (s).
-
Calculate the test statistic: For a one-sample t-test, the test statistic is:
t = (x̄ - μ₀) / (s / √n)
where:
- x̄ is the sample mean
- μ₀ is the hypothesized population mean
- s is the sample standard deviation
- n is the sample size
-
Determine the critical value or p-value:
- Critical value approach: Using the t-distribution table with (n-1) degrees of freedom and the chosen significance level (α), find the critical t-value(s). If the calculated t-statistic falls within the critical region (beyond the critical value(s)), reject the null hypothesis.
- P-value approach: Calculate the p-value, which is the probability of observing a test statistic as extreme as, or more extreme than, the one calculated, assuming the null hypothesis is true. If the p-value is less than the significance level (α), reject the null hypothesis.
-
Make a decision: Based on the comparison of the test statistic to the critical value or the p-value to the significance level, decide whether to reject or fail to reject the null hypothesis.
-
Interpret the results: State your conclusion in the context of the problem. Explain what the results mean in terms of the original research question.
Example: Testing the Mean Weight of Apples
Let's illustrate this with an example. Suppose a farmer claims that the average weight of his apples is 150 grams. We want to test this claim. We collect a random sample of 25 apples and find the following:
- Sample mean (x̄) = 145 grams
- Sample standard deviation (s) = 10 grams
- Significance level (α) = 0.05
- Hypothesized population mean (μ₀) = 150 grams
-
Hypotheses:
- H₀: μ = 150 grams
- H₁: μ ≠ 150 grams (two-tailed test)
-
Significance level: α = 0.05
-
Sample statistics: Already provided above.
-
Test statistic:
t = (145 - 150) / (10 / √25) = -2.5
-
P-value: Using a t-distribution calculator with 24 degrees of freedom and a t-statistic of -2.5, we find a p-value of approximately 0.02.
-
Decision: Since the p-value (0.02) is less than the significance level (0.05), we reject the null hypothesis.
-
Interpretation: There is sufficient evidence to conclude that the average weight of the farmer's apples is different from 150 grams.
Assumptions of the t-test
The validity of the t-test relies on certain assumptions:
- Random sampling: The sample must be randomly selected from the population.
- Independence: The observations in the sample must be independent of each other.
- Normality: The population from which the sample is drawn should be approximately normally distributed, or the sample size should be large enough (generally n ≥ 30) for the Central Limit Theorem to apply. The Central Limit Theorem states that the sampling distribution of the mean will be approximately normal, regardless of the population distribution, if the sample size is sufficiently large.
- Homogeneity of variance (for some t-tests): This is relevant for independent samples t-tests, not for the one-sample t-test we’ve focused on.
Dealing with Violations of Assumptions
If the normality assumption is violated and the sample size is small, non-parametric alternatives to the t-test, such as the Wilcoxon signed-rank test (for one sample), should be considered. These tests do not rely on the assumption of normality.
Power and Sample Size
The power of a hypothesis test is the probability of correctly rejecting the null hypothesis when it is false. A higher power is desirable. Sample size plays a crucial role in power. Larger samples generally lead to higher power. Power analysis can be used to determine the appropriate sample size needed to achieve a desired level of power.
Conclusion
Testing a hypothesis about a population mean is a powerful statistical tool for drawing inferences from sample data. Understanding the underlying principles, choosing the appropriate test, and interpreting the results correctly are essential for valid and reliable conclusions. Remember to always consider the assumptions of the test and consider alternative methods if these assumptions are violated. Careful planning, including power analysis to determine sufficient sample size, is crucial for a successful and meaningful hypothesis test. This comprehensive guide provides a strong foundation for conducting and interpreting these crucial statistical analyses.
Latest Posts
Latest Posts
-
Biologists Use The Fluid Mosaic Model To Describe Membrane Structure
Apr 09, 2025
-
What Is The Location Of A Proton
Apr 09, 2025
-
The Lanthanides And Actinides Belong Between
Apr 09, 2025
-
What Is The Essential Element Common To All Biological Molecules
Apr 09, 2025
-
Naming Binary Compounds Worksheet With Answers
Apr 09, 2025
Related Post
Thank you for visiting our website which covers about Testing A Hypothesis About A Population Mean . We hope the information provided has been useful to you. Feel free to contact us if you have any questions or need further assistance. See you next time and don't miss to bookmark.