The Anova Test Assume The Samples Are Selected
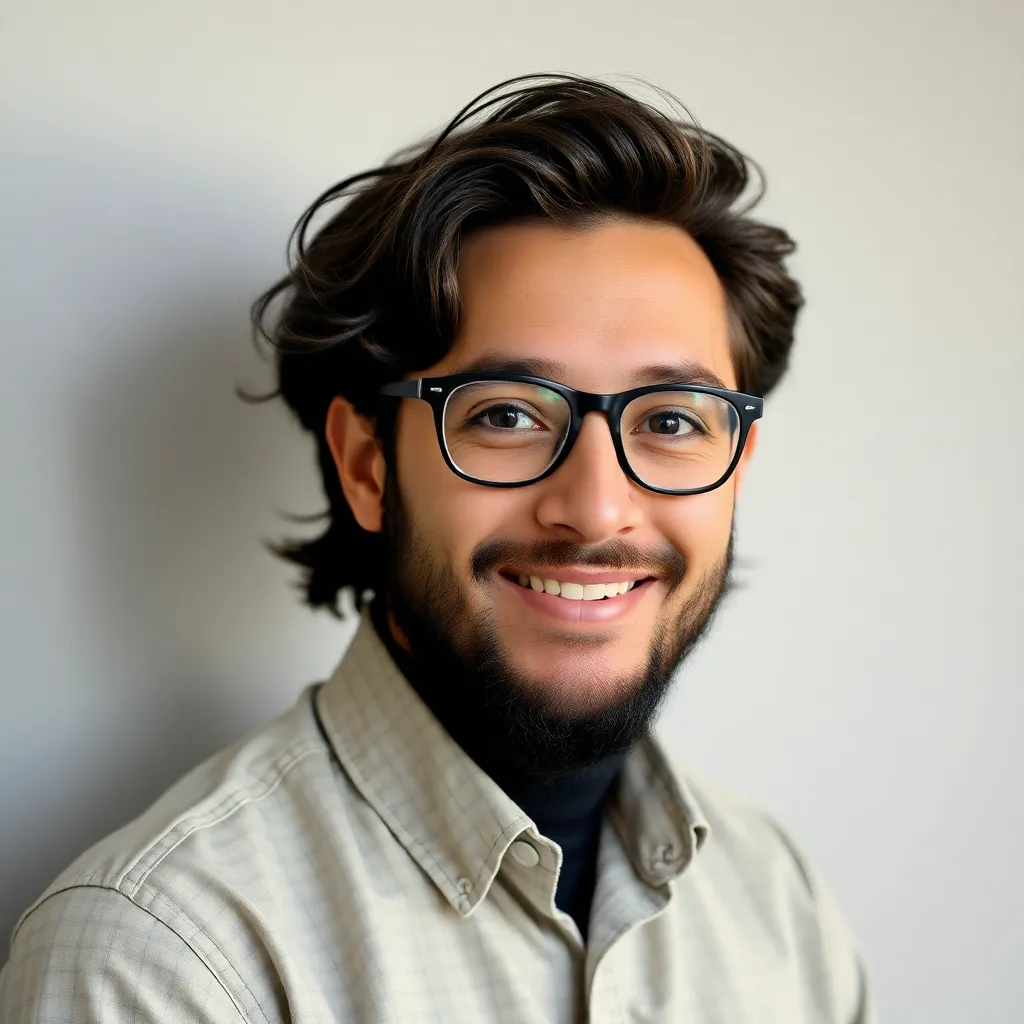
Muz Play
Apr 20, 2025 · 6 min read

Table of Contents
The ANOVA Test: Assumptions and the Crucial Role of Sample Selection
The Analysis of Variance (ANOVA) test is a powerful statistical tool used to compare the means of two or more groups. It's widely applied across various fields, from medicine and agriculture to economics and social sciences. However, the accuracy and reliability of ANOVA results heavily depend on several crucial assumptions, with the method of sample selection playing a pivotal role. This article delves deep into the assumptions underpinning ANOVA tests, specifically focusing on how the way samples are selected impacts the validity of the results. We'll explore different sampling techniques, their implications for ANOVA, and strategies to mitigate potential violations of assumptions.
Key Assumptions of ANOVA
Before delving into sample selection, let's first outline the fundamental assumptions ANOVA relies upon:
1. Independence of Observations:
This assumption is arguably the most critical. It states that observations within and between groups should be independent. This means that the value of one observation doesn't influence the value of another. Violation of independence can lead to inflated Type I error rates (false positives), where you incorrectly reject the null hypothesis.
Examples of violations: Repeated measures on the same subjects without appropriate adjustments (repeated measures ANOVA is needed in such cases), or data collected from clustered samples (e.g., multiple students from the same classroom).
2. Normality of Data:
ANOVA assumes that the data within each group are approximately normally distributed. While ANOVA is relatively robust to minor deviations from normality, particularly with larger sample sizes, severe departures can affect the accuracy of the results.
Consequences of violation: Inflated Type I error rate, particularly with small sample sizes and unequal group variances.
3. Homogeneity of Variances (Homoscedasticity):
This assumption posits that the variances of the dependent variable are equal across all groups. Heteroscedasticity (unequal variances) can lead to biased results and affect the accuracy of the F-statistic.
Consequences of violation: Inflated Type I error rate, particularly if the group sizes are unequal. The F-test becomes less powerful, decreasing the probability of detecting a true effect.
The Importance of Sample Selection in Meeting ANOVA Assumptions
The way samples are selected significantly impacts whether these assumptions are met. Let's examine various sampling techniques and their influence on ANOVA:
1. Random Sampling:
Random sampling is the gold standard for ensuring the independence of observations and, to some extent, the normality and homogeneity of variances assumptions. Each member of the population has an equal chance of being selected. This helps to minimize bias and ensures a representative sample.
Advantages: Reduces bias, increases generalizability of results, and promotes the fulfillment of ANOVA assumptions.
Disadvantages: Can be challenging and time-consuming, especially for large populations. May not be feasible in certain situations.
2. Stratified Random Sampling:
Stratified random sampling divides the population into subgroups (strata) based on relevant characteristics and then randomly samples from each stratum. This is useful when you want to ensure representation from different subgroups within the population. It can improve the precision of estimates and help ensure homogeneity of variances if strata are chosen appropriately.
Advantages: Improved representation of subgroups, increased precision of estimates, potential for better meeting homogeneity of variance assumption.
Disadvantages: Requires prior knowledge about the population and its characteristics. Can be more complex to implement than simple random sampling.
3. Cluster Sampling:
Cluster sampling divides the population into clusters (e.g., schools, hospitals, geographical areas) and then randomly selects a sample of clusters. All units within the selected clusters are included in the sample. This is often more cost-effective than other methods but can lead to violations of the independence assumption if the clusters are not sufficiently independent.
Advantages: Cost-effective, easier to implement for large populations.
Disadvantages: Increased risk of violating the independence assumption, potentially leading to higher Type I error rates. Requires careful consideration of cluster selection and potential correlations within clusters.
4. Convenience Sampling:
Convenience sampling involves selecting participants based on their availability. This is a non-probability sampling method, meaning that not all members of the population have an equal chance of being selected. Convenience sampling is often associated with biased samples and increased risk of violating ANOVA assumptions.
Advantages: Easy and inexpensive.
Disadvantages: Highly susceptible to bias, poor representation of the population, and increased likelihood of violating assumptions, rendering ANOVA results unreliable.
5. Quota Sampling:
Quota sampling is a non-probability method where the researcher sets quotas for different subgroups within the population, aiming to achieve a sample that reflects the proportions of these subgroups in the population. While it aims for representation, it still doesn't guarantee random selection within each subgroup, which may affect ANOVA assumptions.
Advantages: Can provide a more representative sample than convenience sampling, especially regarding demographic characteristics.
Disadvantages: Selection within quotas is not random, making it susceptible to bias. This may lead to violations of independence and other ANOVA assumptions.
Dealing with Violations of ANOVA Assumptions
If the assumptions of ANOVA are violated, several strategies can be employed:
1. Transformations:
Data transformations (e.g., log transformation, square root transformation) can sometimes normalize the data and stabilize the variance, bringing the data closer to meeting the assumptions of ANOVA.
2. Non-parametric Tests:
If the assumptions of normality and homogeneity of variances are severely violated and cannot be remedied through transformations, non-parametric alternatives to ANOVA, such as the Kruskal-Wallis test, can be used. These tests are less powerful than ANOVA but do not rely on the same assumptions.
3. Robust ANOVA Methods:
Robust ANOVA methods are designed to be less sensitive to violations of assumptions, particularly regarding normality and homogeneity of variances. These methods offer a compromise between the power of standard ANOVA and the flexibility of non-parametric approaches.
4. Adjusting the Sample Size:
Increasing the sample size can sometimes improve the robustness of ANOVA to violations of assumptions. Larger samples tend to be more normally distributed according to the Central Limit Theorem.
Conclusion: Sample Selection and ANOVA Validity
The choice of sampling method significantly influences the validity of ANOVA results. While random sampling is the ideal approach for minimizing bias and ensuring the assumptions are met, it may not always be feasible. Careful consideration of the sampling method and potential violations of assumptions is crucial. If assumptions are violated, researchers should consider employing appropriate transformations, non-parametric alternatives, robust methods, or adjusting sample size. A well-designed study with a carefully selected sample is essential for obtaining accurate and reliable results when using ANOVA. Remember that understanding the limitations of your chosen sampling technique and acknowledging potential biases are key aspects of responsible statistical practice. Ignoring these factors can lead to misinterpretations of the data and flawed conclusions. Always prioritize transparency and careful consideration of your methodology when presenting and interpreting ANOVA results. By adhering to these principles, you can enhance the credibility and impact of your research findings.
Latest Posts
Latest Posts
-
Que Es Un Diagrama De Tiras
Apr 20, 2025
-
Nonverbal Communication Is Not Categorized By
Apr 20, 2025
-
Why Do Onions Have No Chloroplasts
Apr 20, 2025
-
Where Are The Respiratory Control Centers Located
Apr 20, 2025
-
The Most Reactive Group Of The Nonmetals Are The
Apr 20, 2025
Related Post
Thank you for visiting our website which covers about The Anova Test Assume The Samples Are Selected . We hope the information provided has been useful to you. Feel free to contact us if you have any questions or need further assistance. See you next time and don't miss to bookmark.