The Mean Of The Sampling Distribution Of Means Is Always
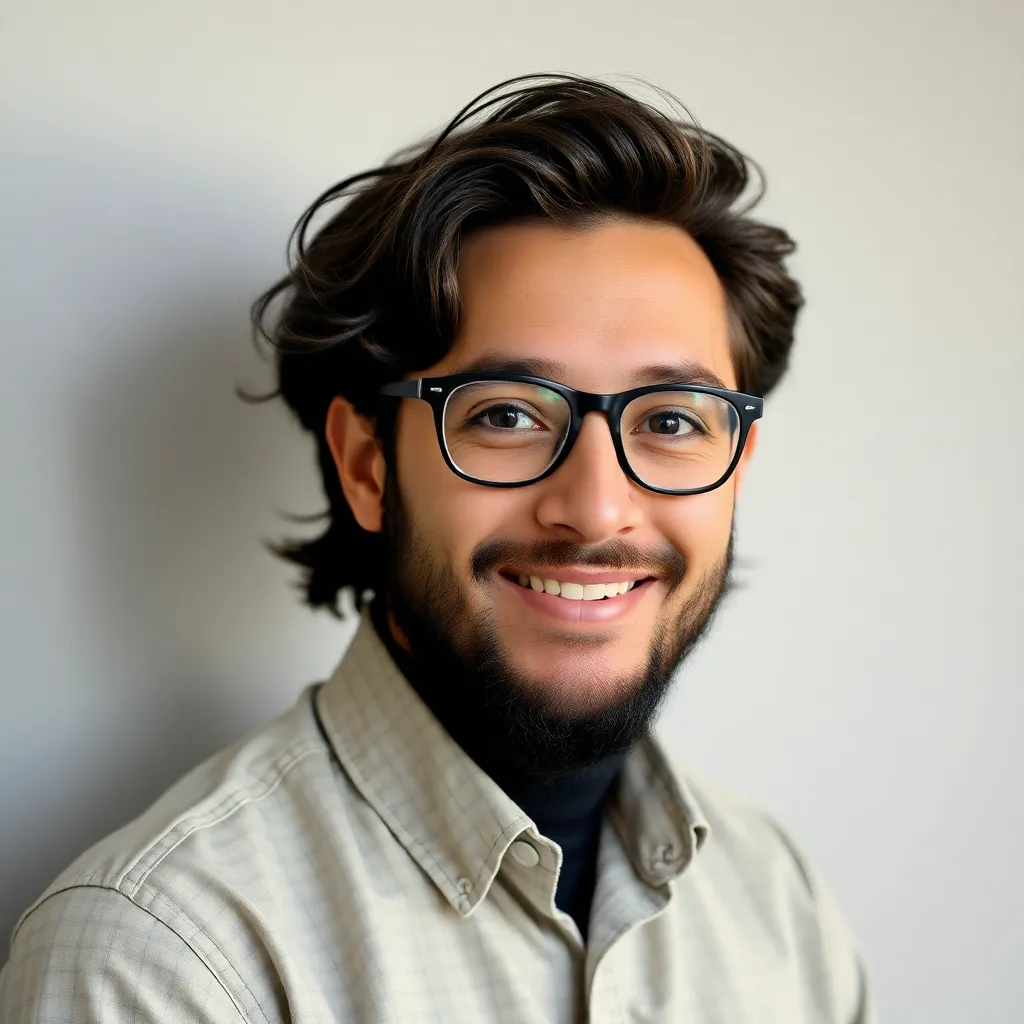
Muz Play
Apr 08, 2025 · 6 min read

Table of Contents
The Mean of the Sampling Distribution of Means is Always Equal to the Population Mean: A Deep Dive
Understanding the sampling distribution of the means is crucial for anyone working with statistical inference. A common, yet fundamental, concept within this area is that the mean of the sampling distribution of means is always equal to the population mean. This principle underpins many statistical tests and estimations, and a thorough grasp of it is vital for accurate data analysis and interpretation. This article will delve into this principle, providing a detailed explanation, practical examples, and addressing common misconceptions.
What is a Sampling Distribution of Means?
Before exploring the central theorem, let's define our key concept: the sampling distribution of means. Imagine you have a population of data – this could be the height of every adult in a country, the weight of every apple in an orchard, or the scores of every student on a particular exam. The population mean (µ) represents the average value of this data.
Now, imagine taking multiple random samples from this population. Each sample will have its own mean (x̄). If you were to plot all these sample means, you would create a new distribution – the sampling distribution of means. This distribution doesn't represent the original data points but rather the distribution of the averages calculated from numerous samples.
The Central Limit Theorem: The Foundation of the Principle
The assertion that the mean of the sampling distribution of means is equal to the population mean is directly linked to the Central Limit Theorem (CLT). This theorem is one of the most important in statistics. It states that, regardless of the shape of the original population distribution, the sampling distribution of the means will approximate a normal distribution as the sample size (n) increases.
This approximation becomes increasingly accurate as the sample size grows larger. Typically, a sample size of 30 or more is considered sufficient for the CLT to hold reasonably well, especially if the original population is not too heavily skewed.
However, it's crucial to emphasize that the CLT doesn't just state that the sampling distribution becomes approximately normal; it also dictates that the mean of this normal distribution is exactly equal to the population mean (µ). This is the core principle we're exploring.
Mathematically: E(x̄) = µ
Where:
- E(x̄) represents the expected value (mean) of the sampling distribution of means.
- µ represents the population mean.
This equation encapsulates the essence of the relationship: the average of all the sample means will always be the same as the average of the entire population.
Why is this Principle Important?
This principle's significance cannot be overstated. It forms the bedrock of many statistical procedures, including:
-
Hypothesis testing: Many statistical tests, like the t-test and z-test, rely on the assumption that the sampling distribution of means is approximately normal and has a known mean (the population mean). This allows us to calculate probabilities and make inferences about the population based on our sample data.
-
Confidence intervals: Constructing confidence intervals for the population mean uses the sampling distribution of means. Knowing that its mean equals the population mean helps in determining the range of values within which the true population mean likely falls.
-
Estimation: We often use sample means to estimate population means. The fact that the mean of the sampling distribution is equal to the population mean ensures that, on average, our sample means provide unbiased estimations.
Illustrative Example: Coin Tosses
Let's illustrate with a simple example: flipping a fair coin. The population consists of two outcomes: heads (H) and tails (T). We can assign numerical values: H=1, T=0. The population mean (µ) is 0.5 (50% chance of heads).
Now, let's take numerous samples of, say, 10 coin flips each. Each sample will yield a sample mean (x̄) – the proportion of heads in that sample. Some samples might have more heads (higher x̄), others fewer (lower x̄). If we plot all these sample means, we'll get the sampling distribution of means.
Even though individual sample means will vary, the average of all these sample means (E(x̄)) will converge towards 0.5 – the population mean – as the number of samples increases. This demonstrates the principle in action.
Addressing Common Misconceptions
Several misconceptions surround the sampling distribution of means. Let's clarify some common points of confusion:
-
Sample size doesn't affect the mean: While the sample size significantly impacts the shape and variability (standard error) of the sampling distribution, it doesn't alter its mean. The mean of the sampling distribution remains consistently equal to the population mean, regardless of sample size (although with smaller samples the approximation to normality is less reliable).
-
Population distribution doesn't affect the mean (but it does affect the shape): The shape of the original population distribution influences the shape of the sampling distribution, particularly at smaller sample sizes. However, it does not affect the mean of the sampling distribution. The mean remains equal to the population mean regardless of the original population's shape (normal, skewed, uniform, etc.).
-
The sampling distribution is always normal: While the Central Limit Theorem states that the sampling distribution approximates a normal distribution for large sample sizes, it's not always perfectly normal, especially with smaller samples or highly skewed populations. However, the mean remains the same even when the distribution isn't perfectly normal.
Practical Applications Beyond the Basics
The principle of the sampling distribution of means having a mean equal to the population mean extends beyond simple examples. Its implications are far-reaching in various statistical fields:
-
Quality Control: In manufacturing, this principle helps determine if a production process is generating products within acceptable tolerances. By taking samples and analyzing the mean of the sampling distribution, manufacturers can assess whether the process is centered around the desired target value.
-
Medical Research: Clinical trials rely heavily on statistical inference. Understanding the sampling distribution of means is crucial in analyzing the effectiveness of a new treatment by comparing the means of treatment and control groups. The principle ensures that the analysis is robust and reliable.
-
Social Sciences: Researchers use this principle when studying population characteristics like income, education levels, or voting patterns. Sampling and analysis of sample means provide valuable insights into the overall population.
-
Finance: Financial modeling and risk assessment often involve analyzing returns on investments. The sampling distribution of means provides a framework for understanding the expected return and its variability.
Conclusion
The mean of the sampling distribution of means is always equal to the population mean. This seemingly simple statement is a cornerstone of statistical inference. Understanding this principle is fundamental for accurately interpreting data, conducting hypothesis testing, constructing confidence intervals, and making sound estimations about populations based on sample data. The Central Limit Theorem provides the theoretical foundation, while its practical applications are ubiquitous across diverse fields, showcasing its importance in data-driven decision-making. A solid grasp of this concept is indispensable for anyone involved in statistical analysis.
Latest Posts
Latest Posts
-
Similarities Between Nervous System And Endocrine System
Apr 17, 2025
-
Explain How Dimensional Analysis Is Used To Solve Problems
Apr 17, 2025
-
Electric Field Lines Of Two Positive Charges
Apr 17, 2025
-
Atoms Of Which Elements Tend To Lose Electrons
Apr 17, 2025
-
Compound Made Up Of Carbon Oxygen Hydrogen Nitrogen And Sulfur
Apr 17, 2025
Related Post
Thank you for visiting our website which covers about The Mean Of The Sampling Distribution Of Means Is Always . We hope the information provided has been useful to you. Feel free to contact us if you have any questions or need further assistance. See you next time and don't miss to bookmark.