The Sampling Distribution Of The Difference Helps Us Determine ________.
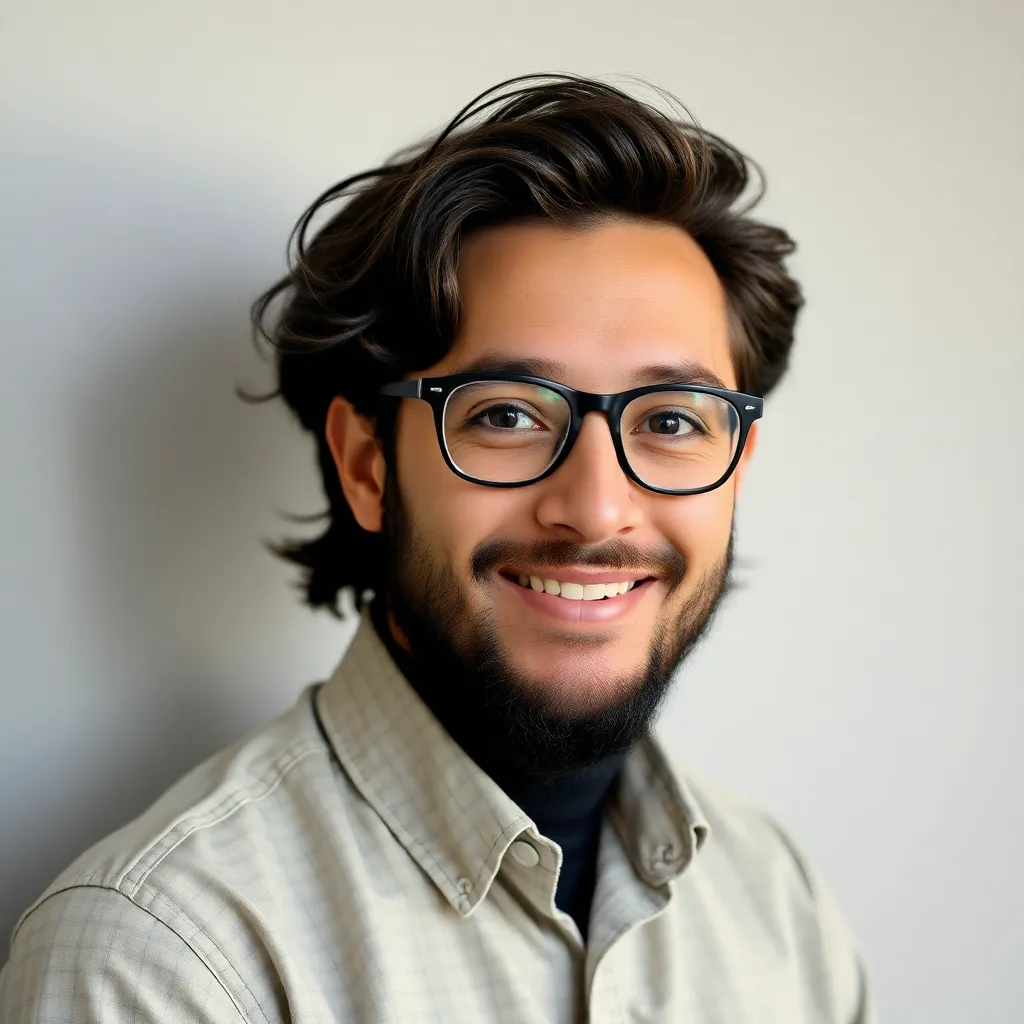
Muz Play
Apr 04, 2025 · 7 min read

Table of Contents
The Sampling Distribution of the Difference Helps Us Determine the Significance of Differences Between Two Groups
The sampling distribution of the difference between two means is a critical concept in inferential statistics. It helps us determine the statistical significance of observed differences between two groups. This means it allows us to assess whether the difference we see in our sample data is likely to reflect a real difference in the populations from which the samples were drawn, or whether it's simply due to random chance. Understanding this distribution is crucial for making informed conclusions based on statistical analysis.
Understanding the Concept: Sampling Distributions
Before diving into the difference between two means, let's review the fundamental concept of sampling distributions. A sampling distribution is the probability distribution of a statistic (like a mean, proportion, or difference between means) obtained from a large number of samples drawn from a population. It shows the range of values the statistic could take and the probability of each value occurring. The key takeaway is that these distributions are theoretical constructs; we don't actually collect thousands of samples to create one. Instead, we use statistical theory to understand their properties.
Why are Sampling Distributions Important?
Sampling distributions are vital because they allow us to make inferences about the population based on a single sample. Instead of relying solely on the results of our sample, we consider the variability inherent in sampling. The sampling distribution tells us how much variation we should expect to see in our sample statistic due to random sampling error. This understanding is essential for hypothesis testing and constructing confidence intervals.
The Sampling Distribution of the Difference Between Two Means
Now, let's focus on the specific case of the sampling distribution of the difference between two means. Suppose we have two populations (Population 1 and Population 2) and we draw independent random samples from each. We calculate the mean for each sample, obtaining $\bar{x}_1$ and $\bar{x}_2$. The difference between these sample means, $d = \bar{x}_1 - \bar{x}_2$, is a random variable. Its probability distribution is called the sampling distribution of the difference between two means.
Properties of the Sampling Distribution of the Difference
This sampling distribution has several important properties:
-
Center: The mean of the sampling distribution (often denoted as μ<sub>d</sub>) is equal to the difference between the population means: μ<sub>d</sub> = μ<sub>1</sub> - μ<sub>2</sub>. This means that, on average, the difference between sample means will equal the difference between the population means.
-
Spread: The standard deviation of the sampling distribution (often denoted as σ<sub>d</sub> or the standard error of the difference) reflects the variability of the difference between sample means. It depends on the standard deviations of the populations (σ<sub>1</sub> and σ<sub>2</sub>) and the sample sizes (n<sub>1</sub> and n<sub>2</sub>). The formula for the standard error of the difference is:
σ<sub>d</sub> = √[(σ<sub>1</sub>²/n<sub>1</sub>) + (σ<sub>2</sub>²/n<sub>2</sub>)]
Note that if the population standard deviations are unknown (which is typically the case), we estimate them using the sample standard deviations (s<sub>1</sub> and s<sub>2</sub>).
-
Shape: The shape of the sampling distribution approaches a normal distribution as the sample sizes (n<sub>1</sub> and n<sub>2</sub>) increase. This is due to the Central Limit Theorem, which states that the distribution of the sample mean (and hence the difference between sample means) tends towards normality as the sample size grows, regardless of the shape of the original population distribution. However, for smaller sample sizes, particularly if the population distributions are not normal, the sampling distribution may not be perfectly normal. In such cases, alternative methods like non-parametric tests might be necessary.
Using the Sampling Distribution to Test Hypotheses
The primary use of the sampling distribution of the difference between two means is in hypothesis testing. We often want to determine whether there's a statistically significant difference between the means of two populations. We do this by formulating a null hypothesis (H<sub>0</sub>) and an alternative hypothesis (H<sub>1</sub> or H<sub>a</sub>).
Common Hypotheses
-
Null Hypothesis (H<sub>0</sub>): There is no difference between the population means (μ<sub>1</sub> = μ<sub>2</sub> or μ<sub>1</sub> - μ<sub>2</sub> = 0).
-
Alternative Hypothesis (H<sub>1</sub>): There is a difference between the population means (μ<sub>1</sub> ≠ μ<sub>2</sub>, μ<sub>1</sub> > μ<sub>2</sub>, or μ<sub>1</sub> < μ<sub>2</sub>). The choice of alternative hypothesis depends on the research question.
Conducting the Hypothesis Test
-
Calculate the test statistic: This typically involves calculating a t-statistic (if population standard deviations are unknown) or a z-statistic (if population standard deviations are known). The formula for the t-statistic is:
t = (d - μ<sub>d</sub>) / s<sub>d</sub> where s<sub>d</sub> is the estimated standard error of the difference.
-
Determine the p-value: The p-value represents the probability of observing a sample difference as extreme as (or more extreme than) the one obtained, assuming the null hypothesis is true. It's calculated based on the t-distribution (or z-distribution).
-
Make a decision: Compare the p-value to the significance level (alpha), typically set at 0.05.
-
If the p-value is less than alpha, we reject the null hypothesis. This means there is sufficient evidence to conclude that there is a statistically significant difference between the population means.
-
If the p-value is greater than or equal to alpha, we fail to reject the null hypothesis. This means there is not enough evidence to conclude a statistically significant difference.
-
Interpreting the Results: Beyond Statistical Significance
While statistical significance is important, it's crucial to consider other factors when interpreting results. A statistically significant difference doesn't necessarily imply a practically meaningful difference. The magnitude of the difference, the context of the study, and the potential for bias should all be considered. A small difference might be statistically significant with large sample sizes but insignificant in practical terms. Similarly, large sample sizes can increase the power of the test, making it more likely to detect even small differences.
Effect Size
Effect size measures quantify the magnitude of the difference between two groups. Common effect sizes include Cohen's d, which represents the difference in means divided by the pooled standard deviation. Effect size provides a standardized way of comparing the magnitude of the difference across studies and helps to evaluate the practical significance of the findings.
Confidence Intervals
Besides hypothesis testing, the sampling distribution can be used to construct confidence intervals for the difference between two population means. A 95% confidence interval, for instance, provides a range of values within which we are 95% confident that the true population difference lies. This gives a more complete picture than just a p-value, providing information about both the precision and the magnitude of the estimate.
Assumptions and Considerations
The validity of inferences based on the sampling distribution of the difference depends on certain assumptions:
-
Independence: The samples must be independent of each other. This means that the selection of one sample should not influence the selection of the other sample.
-
Random Sampling: The samples must be randomly selected from their respective populations. This ensures that the samples are representative of the populations.
-
Normality (or large sample sizes): The populations from which the samples are drawn should be approximately normally distributed, or the sample sizes should be large enough (typically n<sub>1</sub> ≥ 30 and n<sub>2</sub> ≥ 30) for the Central Limit Theorem to apply. If the normality assumption is violated, especially with small samples, non-parametric tests may be more appropriate.
-
Equal Variances (sometimes): Some tests assume that the population variances are equal. However, there are versions of the t-test that can handle unequal variances.
Conclusion: The Power of Inference
The sampling distribution of the difference between two means is a fundamental tool in statistical inference. It enables us to draw conclusions about the differences between populations based on data from samples. By understanding the properties of this distribution and applying the appropriate statistical tests, we can determine whether observed differences are statistically significant and assess the magnitude of those differences. Remember, though, statistical significance is just one piece of the puzzle; interpreting the results requires careful consideration of effect sizes, confidence intervals, and the underlying assumptions of the statistical methods used. This holistic approach allows for more robust and meaningful interpretations of the data.
Latest Posts
Latest Posts
-
What Is A Cross Section In Geology
Apr 04, 2025
-
Domain Of A Function Practice Problems
Apr 04, 2025
-
Which Properties Change The Composition Of A Substance
Apr 04, 2025
-
Which Domain Do Humans Belong To
Apr 04, 2025
-
Which Metals Form Cations With Varying Positive Charges
Apr 04, 2025
Related Post
Thank you for visiting our website which covers about The Sampling Distribution Of The Difference Helps Us Determine ________. . We hope the information provided has been useful to you. Feel free to contact us if you have any questions or need further assistance. See you next time and don't miss to bookmark.